Inverse Coefficient of Variation Feature and Multilevel Fusion Technique for Hyperspectral and LiDAR Data Classification
IEEE Journal of Selected Topics in Applied Earth Observations and Remote Sensing(2020)
摘要
Multisource remote sensing data contain complementary information on land covers, but fusing them is a challenging problem due to the heterogeneous nature of the data. This article aims to extract and integrate information from hyperspectral image (HSI) and light detection and ranging (LiDAR) data for land cover classification. As there is a scarcity of a large number of training samples for remotely sensed hyperspectral and LiDAR data, in this article, we propose a model, which is able to perform impressively using a limited number of training samples by extracting effective features representing different characteristics of objects of interest from these two complementary data sources (HSI and LiDAR). A novel feature extraction method named inverse coefficient of variation (ICV) is introduced for HSI, which considers the Gaussian probability of neighborhood between every pair of bands. We, then, propose a two-stream feature fusion approach to integrate the ICV feature with several features extracted from HSI and LiDAR data. We incorporate a fusion unit named canonical correlation analysis as a basic unit for fusing two different sets of features within each stream. We also incorporate the concept of ensemble classification where the features produced by two-stream fusion are distributed into subsets and transformed to improve the feature quality. We compare our method with the existing state-of-the-art methods, which are based on deep learning or handcrafted feature extraction or using both of them. Experimental results show that our proposed approach performs better than other existing methods with a limited number of training samples.
更多查看译文
关键词
Canonical correlation analysis (CCA),fusion,hyperspectral,light detection and ranging (LiDAR),multisource
AI 理解论文
溯源树
样例
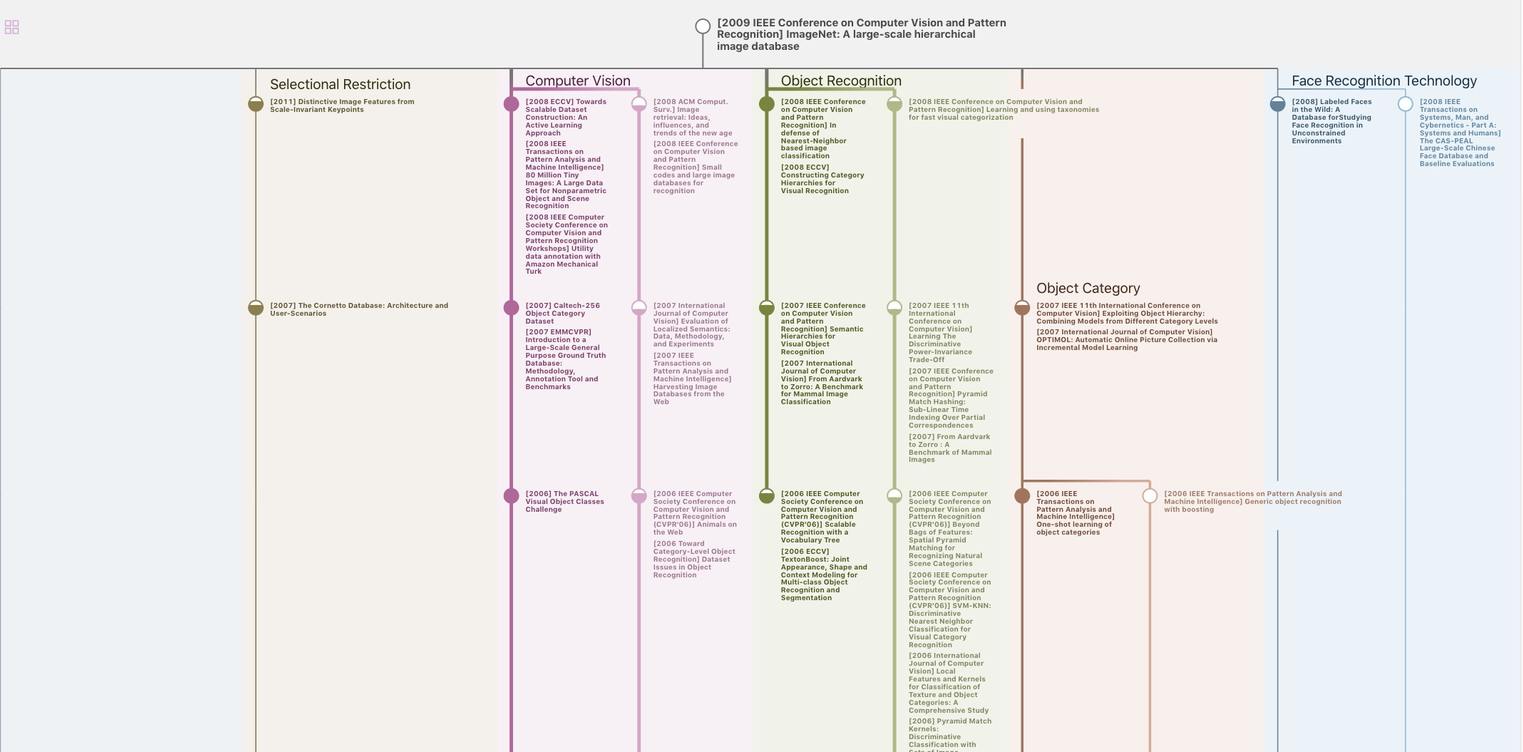
生成溯源树,研究论文发展脉络
Chat Paper
正在生成论文摘要