A hybrid optimized error correction system for time series forecasting.
Applied Soft Computing(2020)
摘要
Time series forecasting is a challenging task in machine learning. Real world time series are often composed by linear and nonlinear structures which need to be mapped by some forecasting method. Linear methods such as autoregressive integrated moving average (ARIMA) and nonlinear methods such as artificial neural networks (ANNs) could be employed to handle such problems, however model misspecification hinders the forecasting process producing inaccurate models. Hybrid models based on error forecasting and combination can reduce the misspecification of single models and improve the accuracy of the system. This work proposes a hybrid system that is composed of three parts: a) linear modeling of the time series, b) nonlinear modeling of the error series, and c) combination of the forecasts using three distinct approaches. The system performs a search for the best parameters of the linear and nonlinear components, and of the combination approaches. Particle swarm optimization is used to find suitable architecture and weights. Experiments show that the proposed technique achieved promising results in time series forecasting.
更多查看译文
关键词
Time series forecasting,Particle swarm optimization,Extreme learning machines,Hybrid intelligent systems,Artificial neural networks
AI 理解论文
溯源树
样例
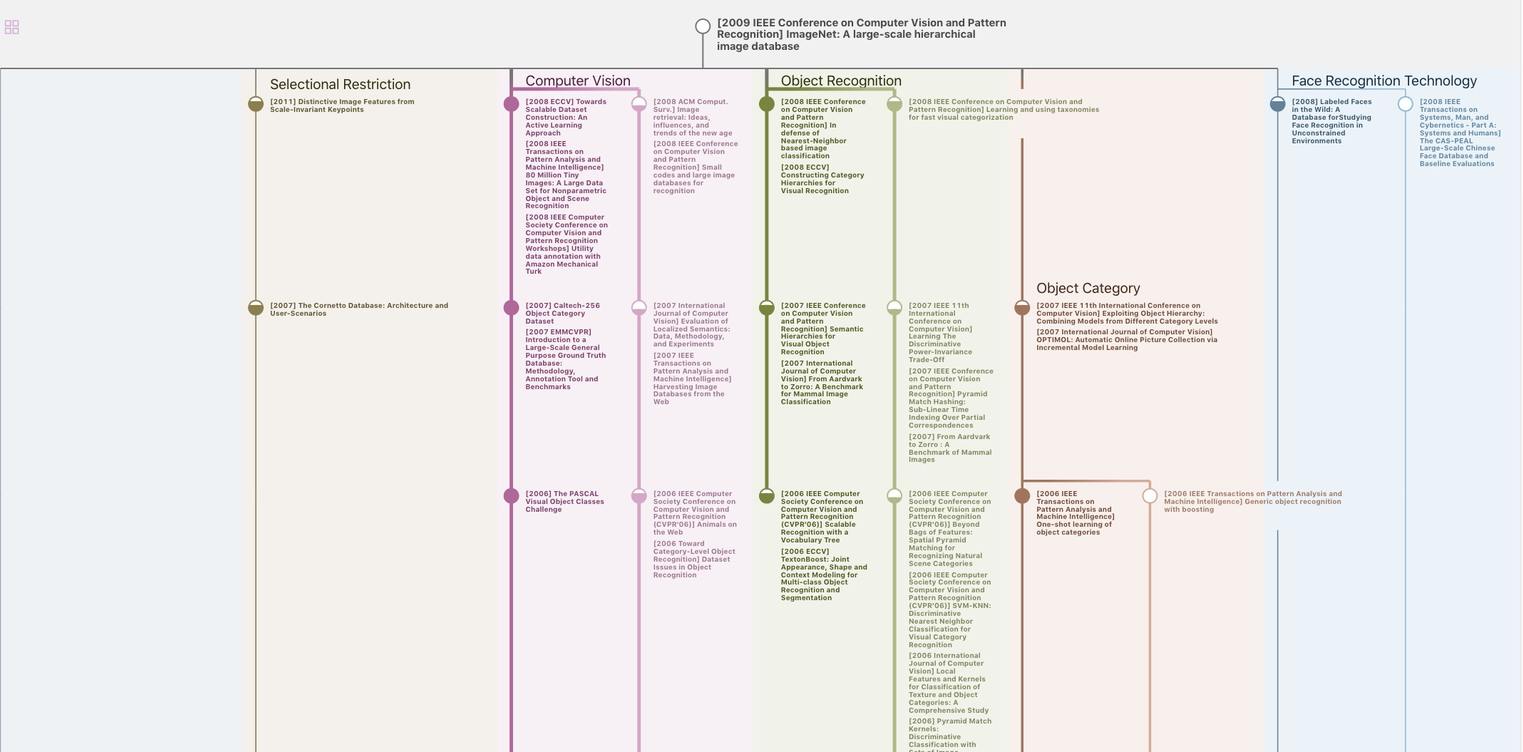
生成溯源树,研究论文发展脉络
Chat Paper
正在生成论文摘要