Attentional Speech Recognition Models Misbehave on Out-of-domain Utterances
arxiv(2020)
摘要
We discuss the problem of echographic transcription in autoregressive sequence-to-sequence attentional architectures for automatic speech recognition, where a model produces very long sequences of repetitive outputs when presented with out-of-domain utterances. We decode audio from the British National Corpus with an attentional encoder-decoder model trained solely on the LibriSpeech corpus. We observe that there are many 5-second recordings that produce more than 500 characters of decoding output (i.e. more than 100 characters per second). A frame-synchronous hybrid (DNN-HMM) model trained on the same data does not produce these unusually long transcripts. These decoding issues are reproducible in a speech transformer model from ESPnet, and to a lesser extent in a self-attention CTC model, suggesting that these issues are intrinsic to the use of the attention mechanism. We create a separate length prediction model to predict the correct number of wordpieces in the output, which allows us to identify and truncate problematic decoding results without increasing word error rates on the LibriSpeech task.
更多查看译文
关键词
speech,models,recognition,out-of-domain
AI 理解论文
溯源树
样例
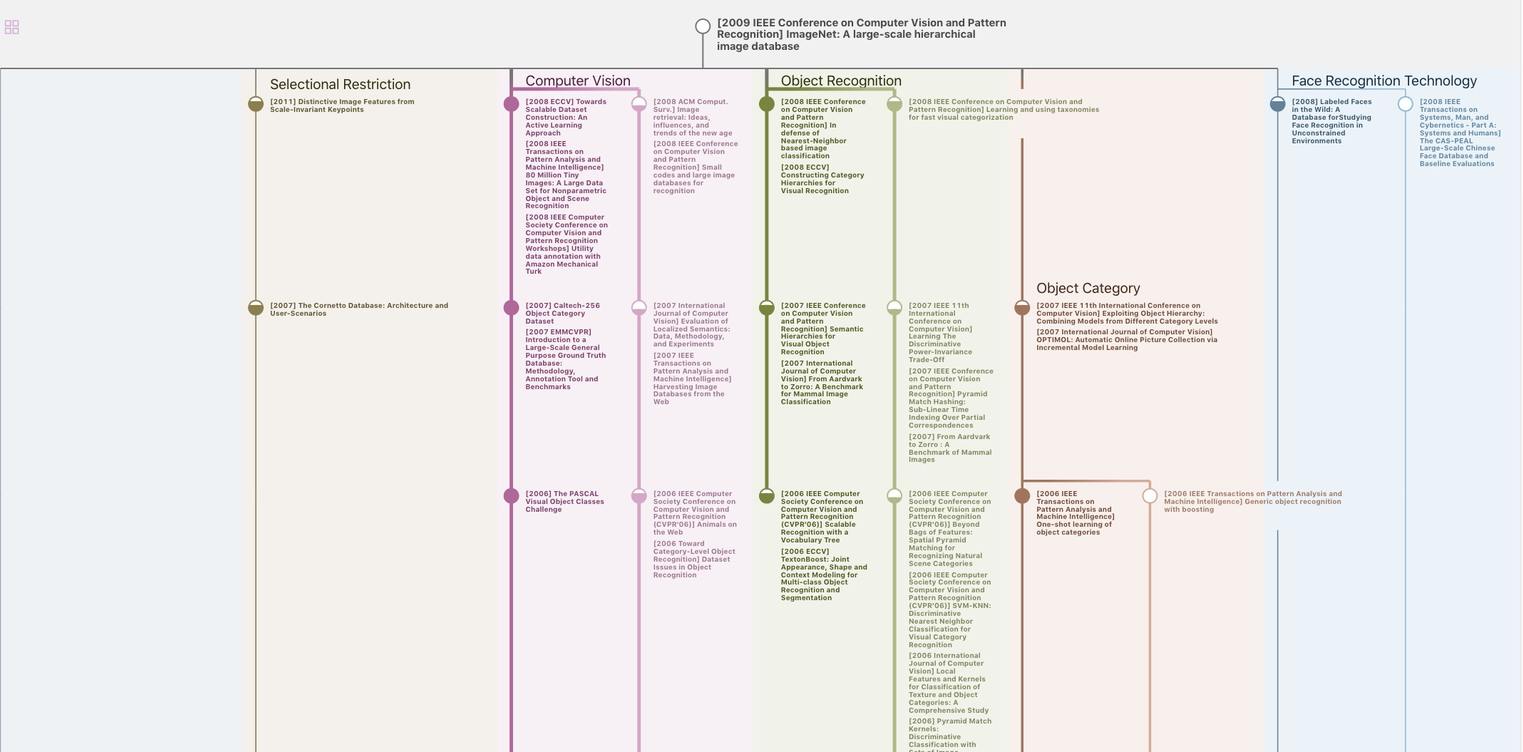
生成溯源树,研究论文发展脉络
Chat Paper
正在生成论文摘要