Reward-rational (implicit) choice: A unifying formalism for reward learning
NIPS 2020(2020)
摘要
It is often difficult to hand-specify what the correct reward function is for a task, so researchers have instead aimed to learn reward functions from human behavior or feedback. The types of behavior interpreted as evidence of the reward function have expanded greatly in recent years. We've gone from demonstrations, to comparisons, to reading into the information leaked when the human is pushing the robot away or turning it off. And surely, there is more to come. How will a robot make sense of all these diverse types of behavior? Our key insight is that different types of behavior can be interpreted in a single unifying formalism - as a reward-rational choice that the human is making, often implicitly. The formalism offers both a unifying lens with which to view past work, as well as a recipe for interpreting new sources of information that are yet to be uncovered. We provide two examples to showcase this: interpreting a new feedback type, and reading into how the choice of feedback itself leaks information about the reward.
更多查看译文
关键词
choice,learning,unifying formalism,reward-rational
AI 理解论文
溯源树
样例
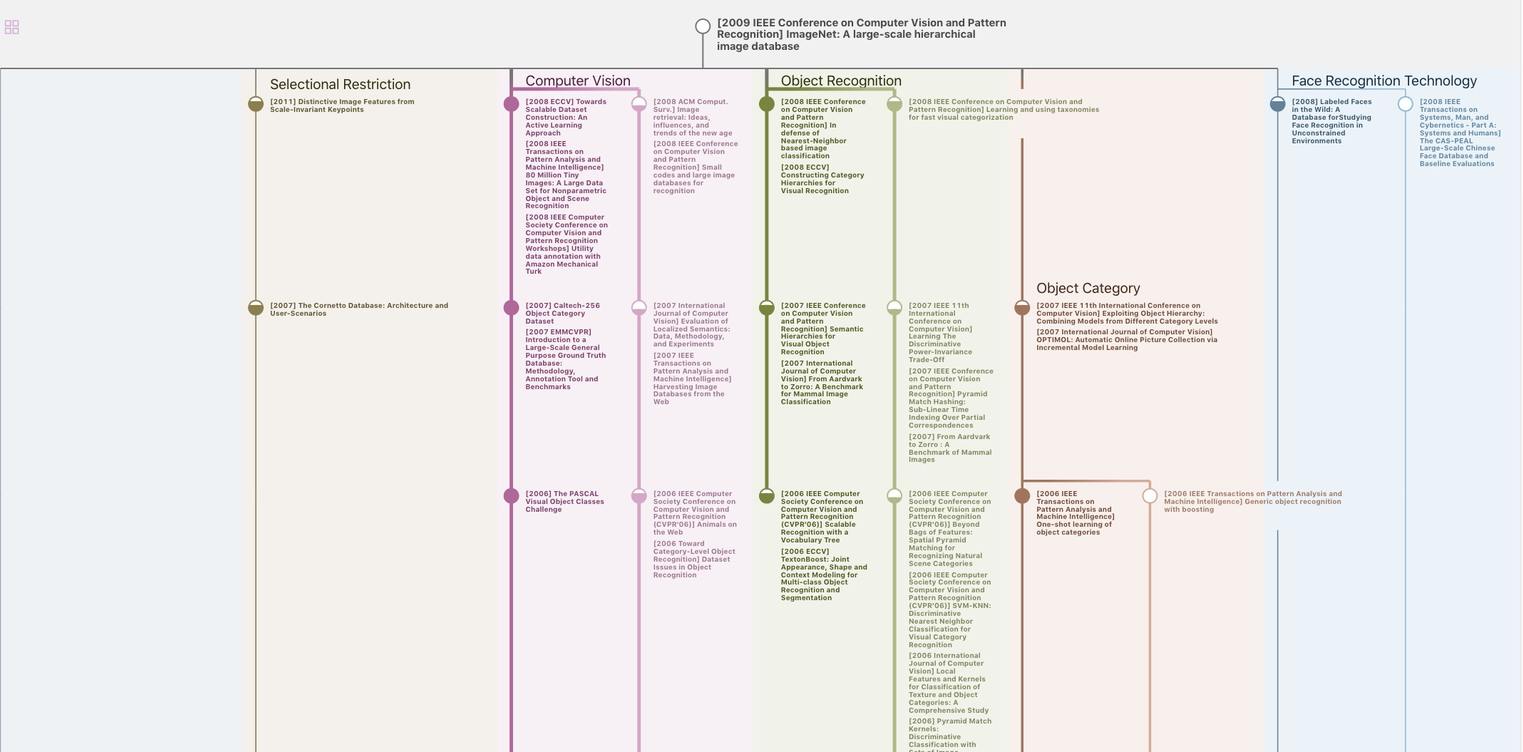
生成溯源树,研究论文发展脉络
Chat Paper
正在生成论文摘要