Deep Multi-task Augmented Feature Learning via Hierarchical Graph Neural Network
MACHINE LEARNING AND KNOWLEDGE DISCOVERY IN DATABASES(2021)
摘要
Deep multi-task learning attracts much attention in recent years as it achieves good performance in many applications. Feature learning is important to deep multi-task learning for sharing common information among tasks. In this paper, we propose a Hierarchical Graph Neural Network (HGNN) to learn augmented features for deep multi-task learning. The HGNN consists of two-level graph neural networks. In the low level, an intra-task graph neural network is responsible of learning a powerful representation for each data point in a task by aggregating its neighbors. Based on the learned representation, a task embedding can be generated for each task in a similar way to max pooling. In the second level, an inter-task graph neural network updates task embeddings of all the tasks based on the attention mechanism to model task relations. Then the task embedding of one task is used to augment the feature representation of data points in this task. Moreover, for classification tasks, an inter-class graph neural network is introduced to conduct similar operations on a finer granularity, i.e., the class level, to generate class embeddings for each class in all the tasks using class embeddings to augment the feature representation. The proposed feature augmentation strategy can be used in many deep multi-task learning models. Experiments on real-world datasets show the significant performance improvement when using this strategy.
更多查看译文
关键词
Multi-task learning, Feature learning, Graph neural network
AI 理解论文
溯源树
样例
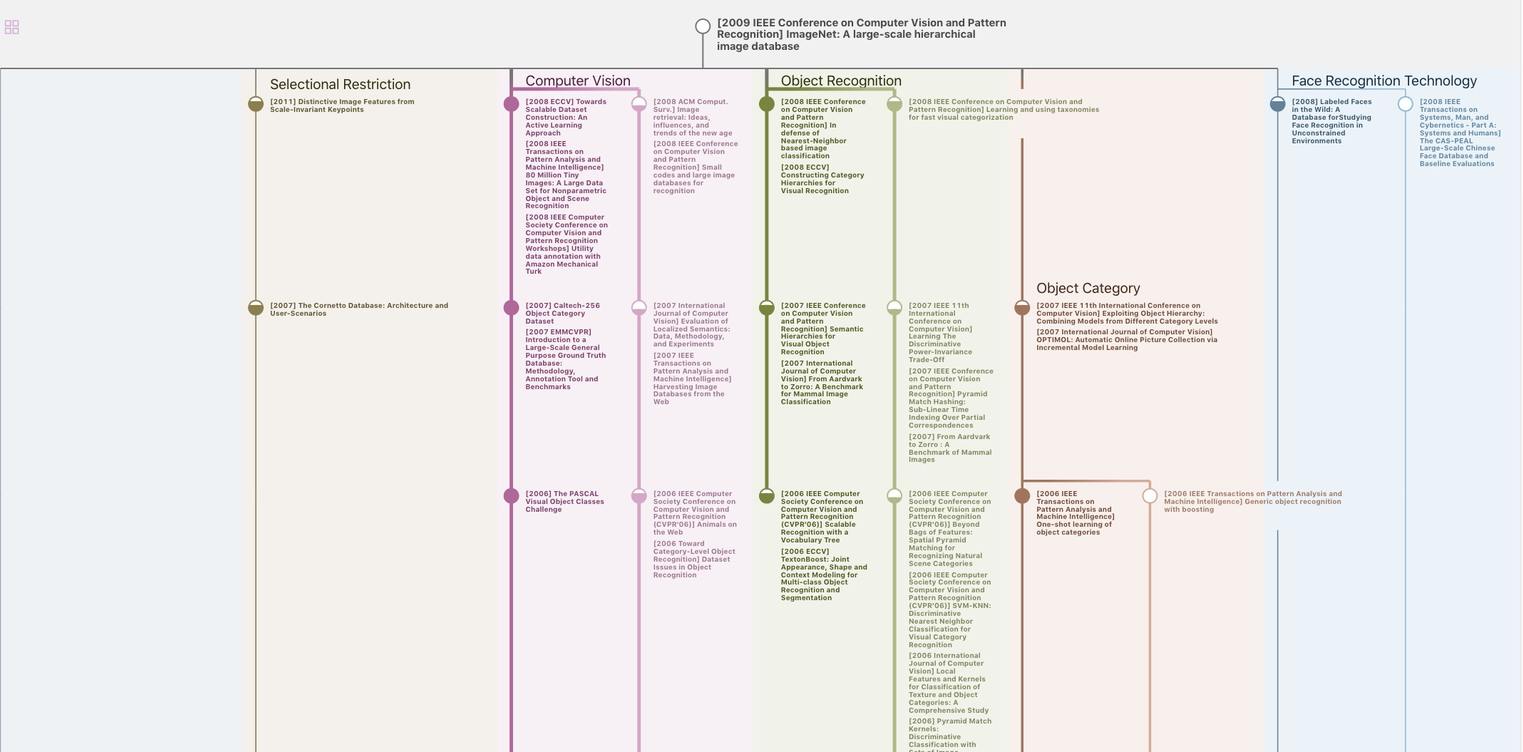
生成溯源树,研究论文发展脉络
Chat Paper
正在生成论文摘要