Topologically Densified Distributions
ICML(2020)
摘要
We study regularization in the context of small sample-size learning with over-parameterized neural networks. Specifically, we shift focus from architectural properties, such as norms on the network weights, to properties of the internal representations before a linear classifier. Specifically, we impose a topological constraint on samples drawn from the probability measure induced in that space. This provably leads to mass concentration effects around the representations of training instances, i.e., a property beneficial for generalization. By leveraging previous work to impose topological constraints in a neural network setting, we provide empirical evidence (across various vision benchmarks) to support our claim for better generalization.
更多查看译文
关键词
distributions
AI 理解论文
溯源树
样例
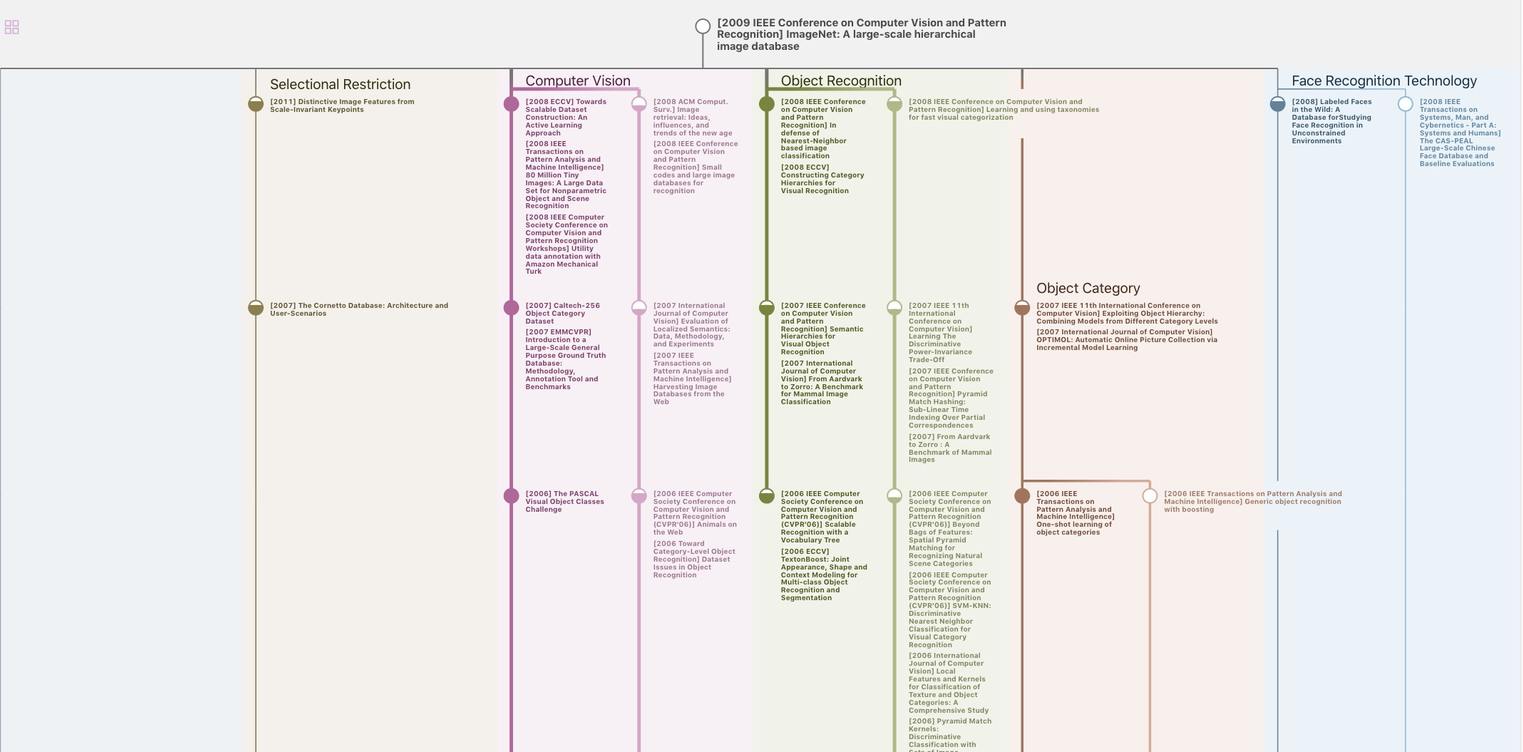
生成溯源树,研究论文发展脉络
Chat Paper
正在生成论文摘要