Improving The Background Of Gravitational-Wave Searches For Core Collapse Supernovae: A Machine Learning Approach
MACHINE LEARNING-SCIENCE AND TECHNOLOGY(2020)
摘要
Based on the prior O1-O2 observing runs, about 30% of the data collected by Advanced LIGO and Virgo in the next observing runs are expected to be single-interferometer data, i.e. they will be collected at times when only one detector in the network is operating in observing mode. Searches for gravitational-wave signals from supernova events do not rely on matched filtering techniques because of the stochastic nature of the signals. If a Galactic supernova occurs during single-interferometer times, separation of its unmodelled gravitational-wave signal from noise will be even more difficult due to lack of coherence between detectors. We present a novel machine learning method to perform single-interferometer supernova searches based on the standard LIGO-Virgo coherent WaveBurst pipeline. We show that the method may be used to discriminate Galactic gravitational-wave supernova signals from noise transients, decrease the false alarm rate of the search, and improve the supernova detection reach of the detectors.
更多查看译文
关键词
gravitational waves, machine learning, genetic programming
AI 理解论文
溯源树
样例
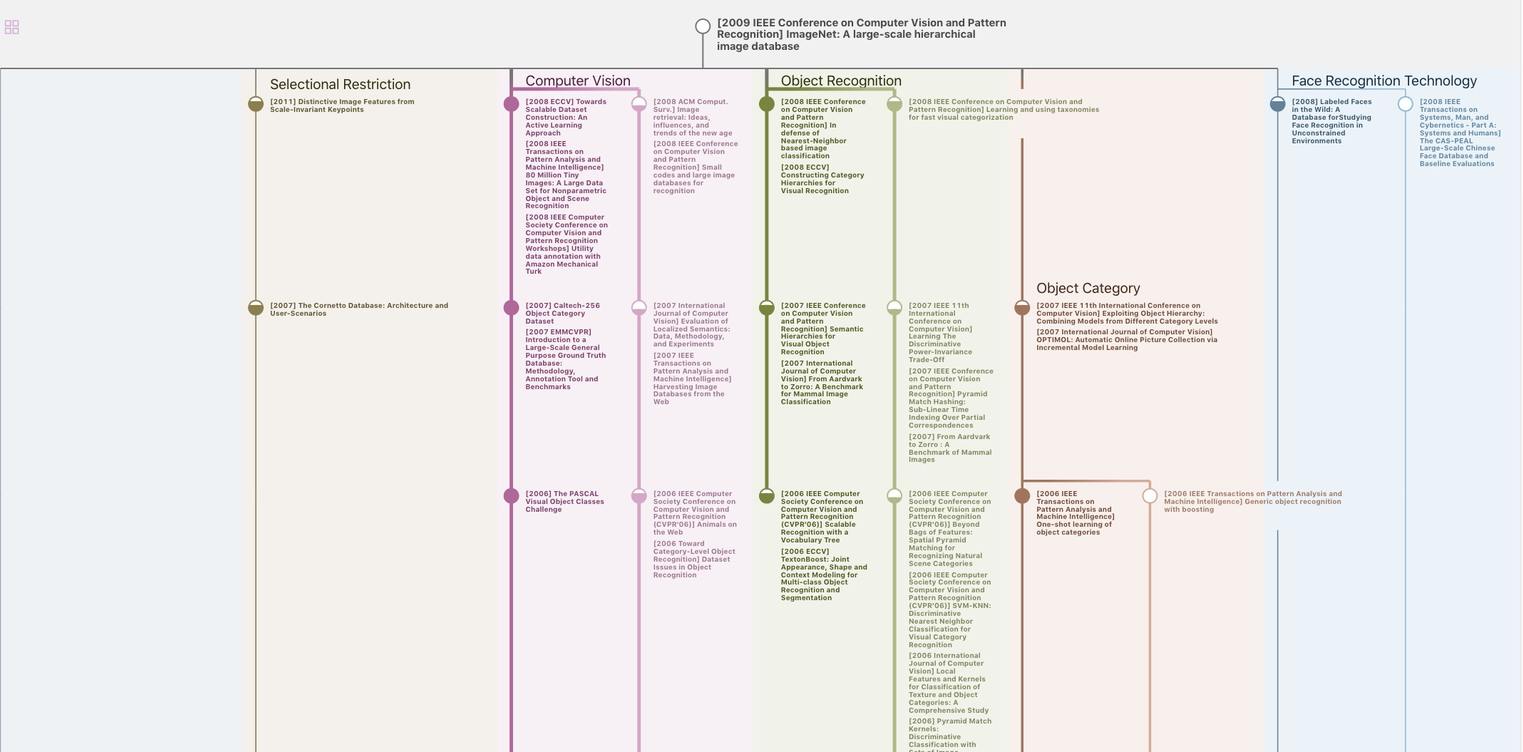
生成溯源树,研究论文发展脉络
Chat Paper
正在生成论文摘要