Mean-Field Analysis of Two-Layer Neural Networks: Non-Asymptotic Rates and Generalization Bounds
arxiv(2020)
摘要
A recent line of work in deep learning theory has utilized the mean-field analysis to demonstrate the global convergence of noisy (stochastic) gradient descent for training over-parameterized two-layer neural networks. However, existing results in the mean-field setting do not provide the convergence rate of neural network training, and the generalization error bound is largely missing. In this paper, we provide a mean-field analysis in a generalized neural tangent kernel regime, and show that noisy gradient descent with weight decay can still exhibit a "kernel-like" behavior. This implies that the training loss converges linearly up to a certain accuracy in such regime. We also establish a generalization error bound for two-layer neural networks trained by noisy gradient descent with weight decay. Our results shed light on the connection between mean field analysis and the neural tangent kernel based analysis.
更多查看译文
关键词
neural networks,generalization bounds,mean-field,two-layer,non-asymptotic
AI 理解论文
溯源树
样例
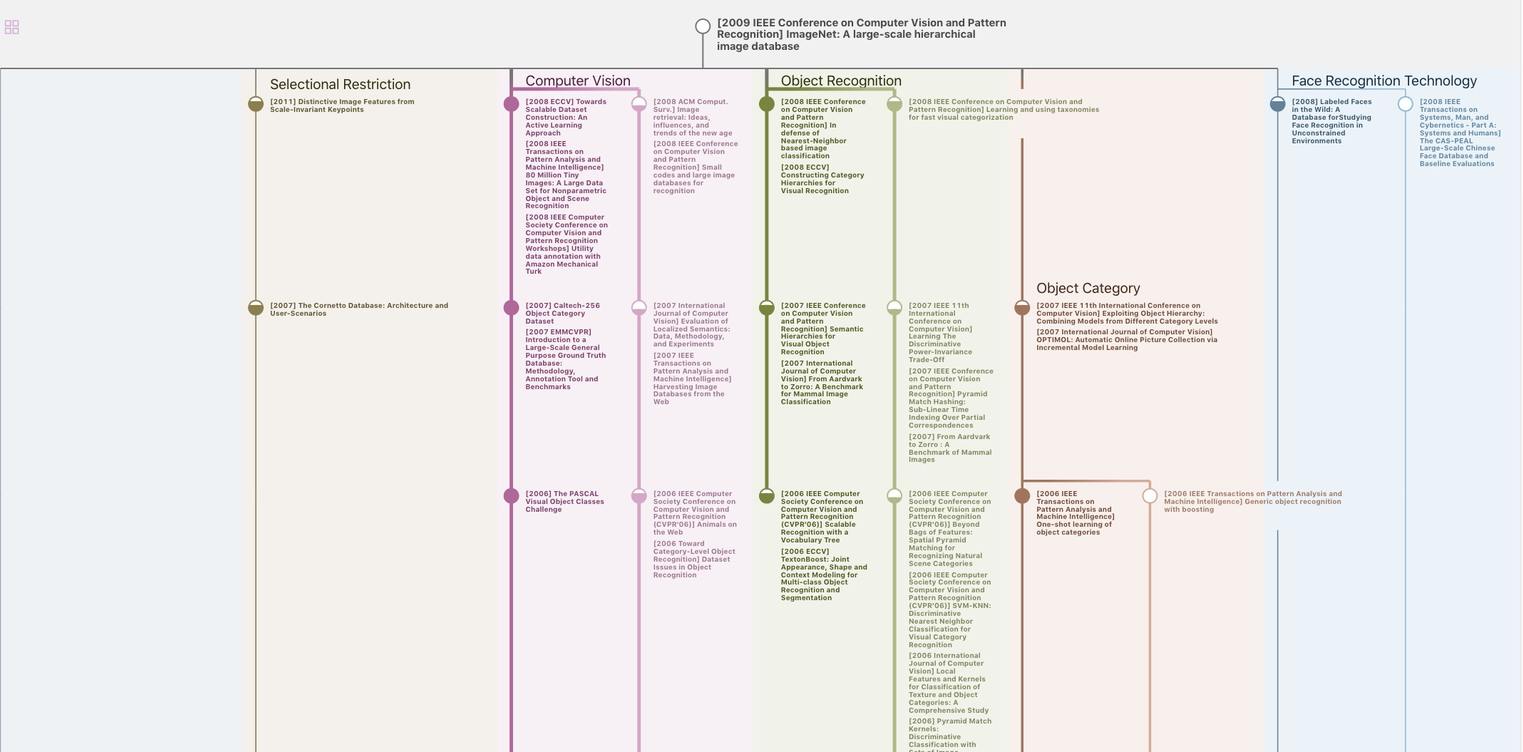
生成溯源树,研究论文发展脉络
Chat Paper
正在生成论文摘要