Representation Learning on Variable Length and Incomplete Wearable-Sensory Time Series
ACM Transactions on Intelligent Systems and Technology(2022)
摘要
AbstractThe prevalence of wearable sensors (e.g., smart wristband) is creating unprecedented opportunities to not only inform health and wellness states of individuals, but also assess and infer personal attributes, including demographic and personality attributes. However, the data captured from wearables, such as heart rate or number of steps, present two key challenges: (1) the time series is often of variable length and incomplete due to different data collection periods (e.g., wearing behavior varies by person); and (2) there is inter-individual variability to external factors like stress and environment. This article addresses these challenges and brings us closer to the potential of personalized insights about an individual, taking the leap from quantified self to qualified self. Specifically, HeartSpace proposed in this article learns embedding of the time-series data with variable length and missing values via the integration of a time-series encoding module and a pattern aggregation network. Additionally, HeartSpace implements a Siamese-triplet network to optimize representations by jointly capturing intra- and inter-series correlations during the embedding learning process. The empirical evaluation over two different real-world data presents significant performance gains over state-of-the-art baselines in a variety of applications, including user identification, personality prediction, demographics inference, job performance prediction, and sleep duration estimation.
更多查看译文
关键词
Representation learning,wearable-sensory time series
AI 理解论文
溯源树
样例
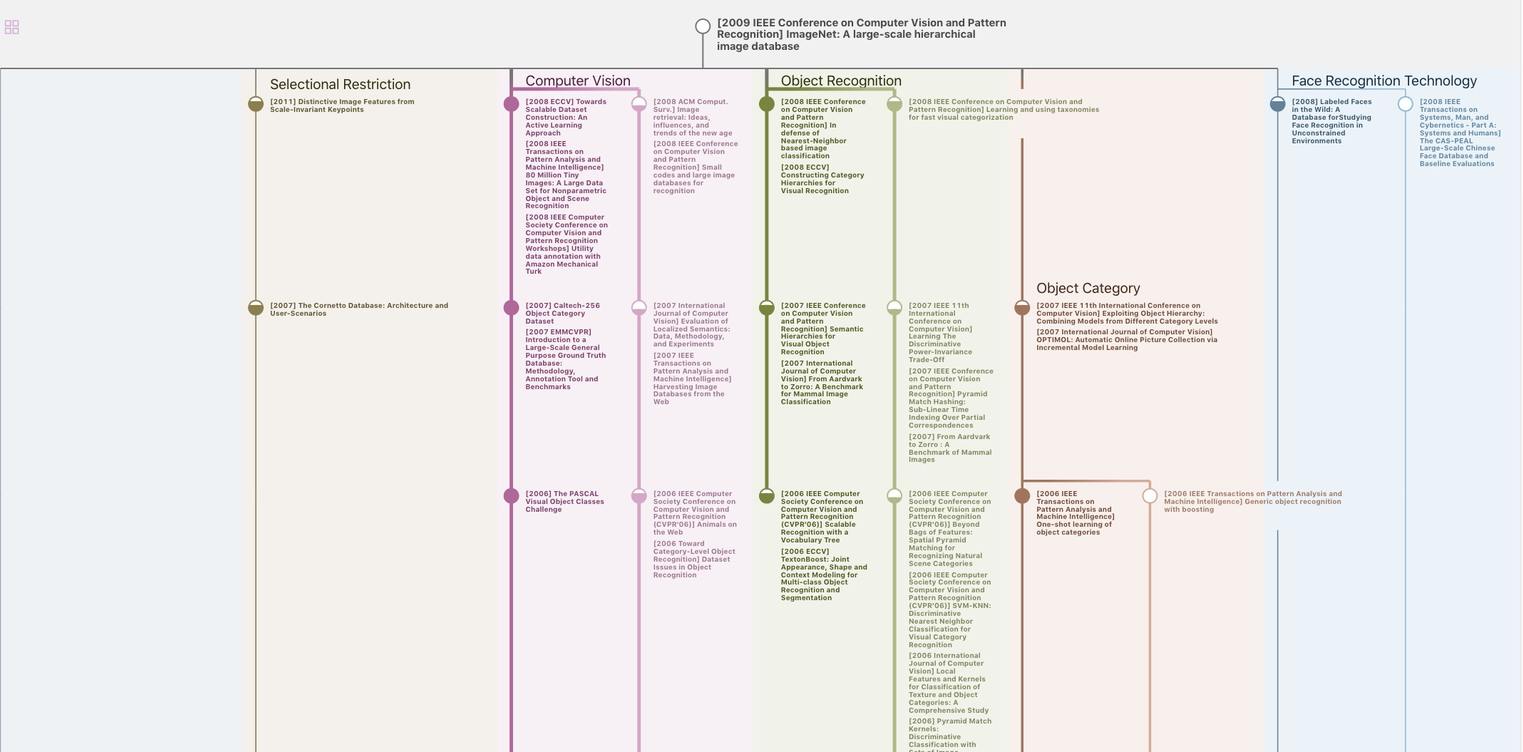
生成溯源树,研究论文发展脉络
Chat Paper
正在生成论文摘要