Tuning of multivariable model predictive controllers through expert bandit feedback
INTERNATIONAL JOURNAL OF CONTROL(2021)
摘要
For certain industrial control applications an explicit function capturing the non-trivial trade-off between competing objectives in closed loop performance is not available. In such scenarios it is common practice to use the human innate ability to implicitly learn such a relationship and manually tune the corresponding controller to achieve the desirable closed loop performance. This approach has its deficiencies because of individual variations due to experience levels and preferences in the absence of an explicit calibration metric. Moreover, as the complexity of the underlying system and/or the controller increase, in the effort to achieve better performance, so does the tuning time and the associated tuning cost. To reduce the overall tuning cost, a tuning framework is proposed herein, whereby a supervised machine learning is used to extract the human-learned cost function and an optimisation algorithm that can efficiently deal with a large number of variables, is used for optimising the extracted cost function. Given the interest in the implementation across many industrial domains and the associated high degree of freedom present in the corresponding tuning process, a Model Predictive Controller applied to air path control in a diesel engine is tuned for the purpose of demonstrating the potential of the framework.
更多查看译文
关键词
Model predictive control tuning,machine learning,automotive control
AI 理解论文
溯源树
样例
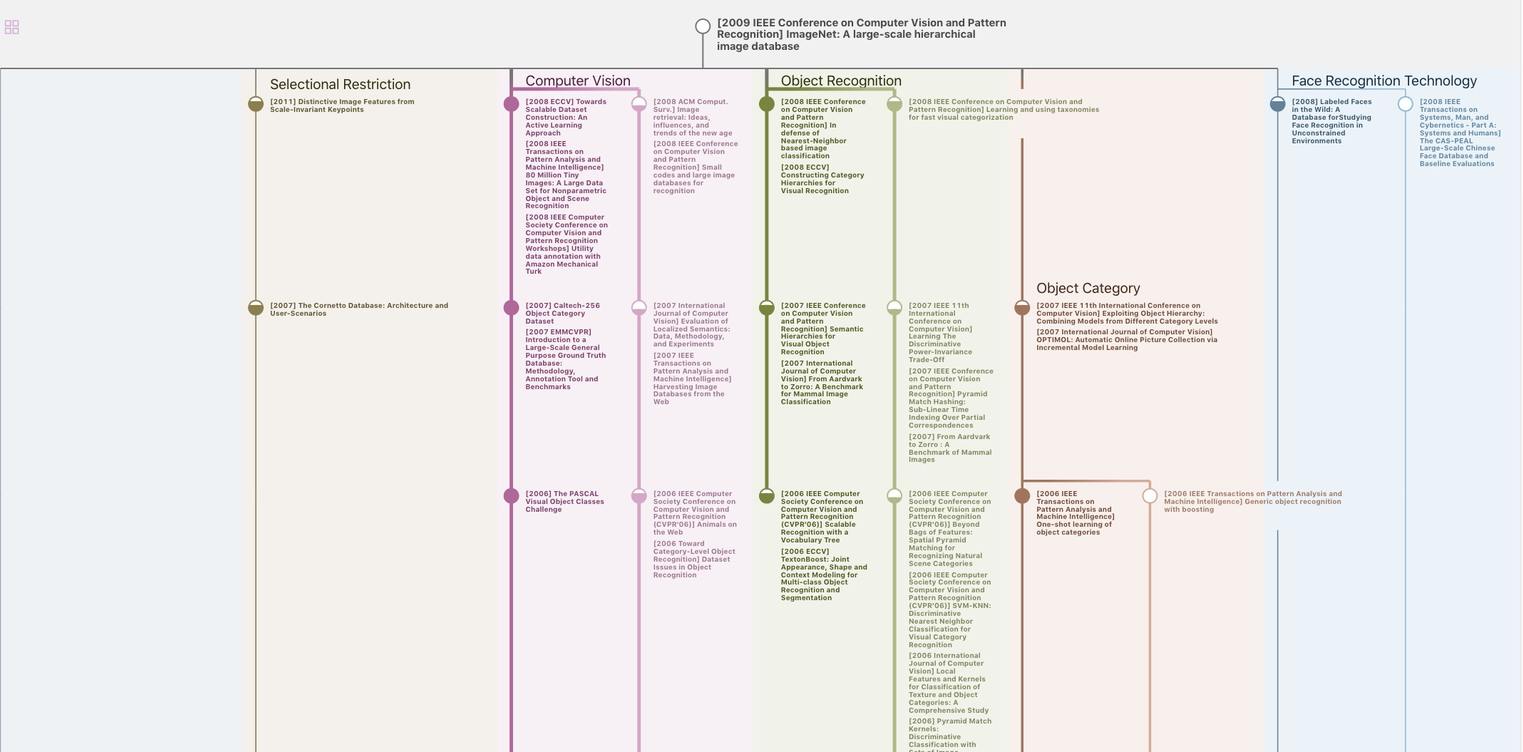
生成溯源树,研究论文发展脉络
Chat Paper
正在生成论文摘要