Prediction Of All-Cause Mortality In Haemodialysis Patients Using A Bayesian Network
NEPHROLOGY DIALYSIS TRANSPLANTATION(2020)
摘要
Background. All-cause mortality in haemodialysis (HD) is high, reaching 15.6% in the first year according to the European Renal Association.Methods. A new clinical tool to predict all-cause mortality in HD patients is proposed. It uses a post hoc analysis of data from the prospective cohort study Photo-Graph V3. A total of 35 variables related to patient characteristics, laboratory values and treatments were used as predictors of all-cause mortality. The first step was to compare the results obtained using a logistic regression to those obtained by a Bayesian network. The second step aimed to increase the performance of the best prediction model using synthetic data. Finally, a compromise between performance and ergonomics was proposed by reducing the number of variables to be entered in the prediction tool.Results. Among the 9010 HD patients included in the PhotoGraph V3 study, 4915 incident patients with known medical status at 2 years were analysed. All-cause mortality at 2 years was 34.1%. The Bayesian network provided the most reliable prediction. The final optimized models that used 14 variables had areas under the receiver operating characteristic curves of 0.78 +/- 0.01, sensitivity of 72 +/- 2%, specificity of 69 +/- 2%, predictive positive value of 70 +/- 1% and negative predictive value of 71 +/- 2% for the prediction of all-cause mortality.Conclusions. Using artificial intelligence methods, a new clinical tool to predict all-cause mortality in incident HD patients is proposed. The latter can be used for research purposes before its external validation at: https://www.hed.cc/? a=twoyearsallcausemortalityhemod8m=2-years%20All-cause %20Mortality9620Hemodialysis.neta.
更多查看译文
关键词
Bayesian network, epidemiology, haemodialysis, mortality, risk prediction
AI 理解论文
溯源树
样例
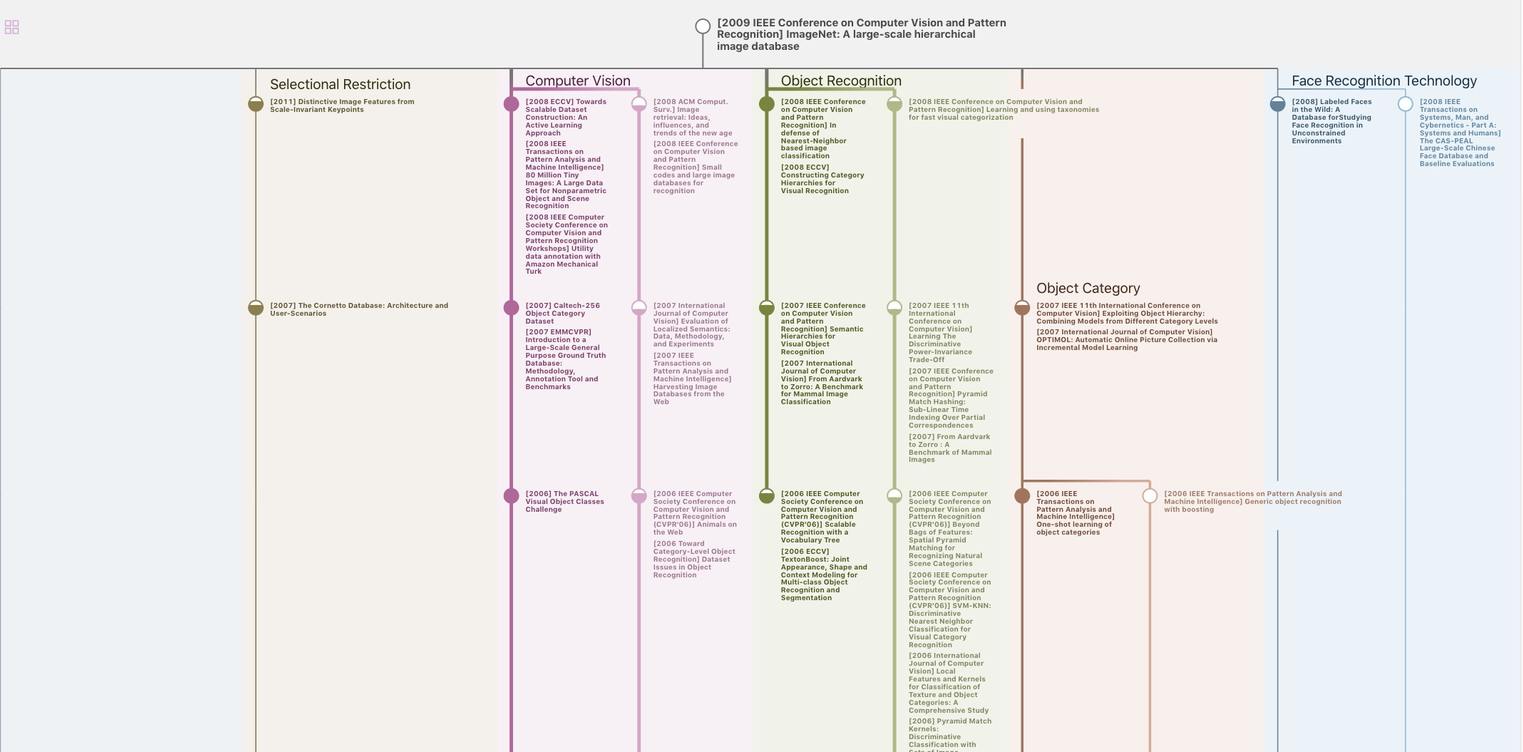
生成溯源树,研究论文发展脉络
Chat Paper
正在生成论文摘要