Temporal Segmentation of Surgical Sub-tasks through Deep Learning with Multiple Data Sources
2020 IEEE International Conference on Robotics and Automation (ICRA)(2020)
摘要
Many tasks in robot-assisted surgeries (RAS) can be represented by finite-state machines (FSMs), where each state represents either an action (such as picking up a needle) or an observation (such as bleeding). A crucial step towards the automation of such surgical tasks is the temporal perception of the current surgical scene, which requires a real-time estimation of the states in the FSMs. The objective of this work is to estimate the current state of the surgical task based on the actions performed or events occurred as the task progresses. We propose Fusion-KVE, a unified surgical state estimation model that incorporates multiple data sources including the Kinematics, Vision, and system Events. Additionally, we examine the strengths and weaknesses of different state estimation models in segmenting states with different representative features or levels of granularity. We evaluate our model on the JHU-ISI Gesture and Skill Assessment Working Set (JIGSAWS), as well as a more complex dataset involving robotic intra-operative ultrasound (RIOUS) imaging, created using the da Vinci® Xi surgical system. Our model achieves a superior frame-wise state estimation accuracy up to 89.4%, which improves the state-of-the-art surgical state estimation models in both JIGSAWS suturing dataset and our RIOUS dataset.
更多查看译文
关键词
Skill Assessment Working Set,robotic intra-operative ultrasound imaging,da Vinci® Xi surgical system,superior frame-wise state estimation accuracy,temporal segmentation,deep learning,data sources,robot-assisted surgeries,finite-state machines,surgical task,temporal perception,current surgical scene,real-time estimation,task progresses,state estimation models,surgical state estimation models
AI 理解论文
溯源树
样例
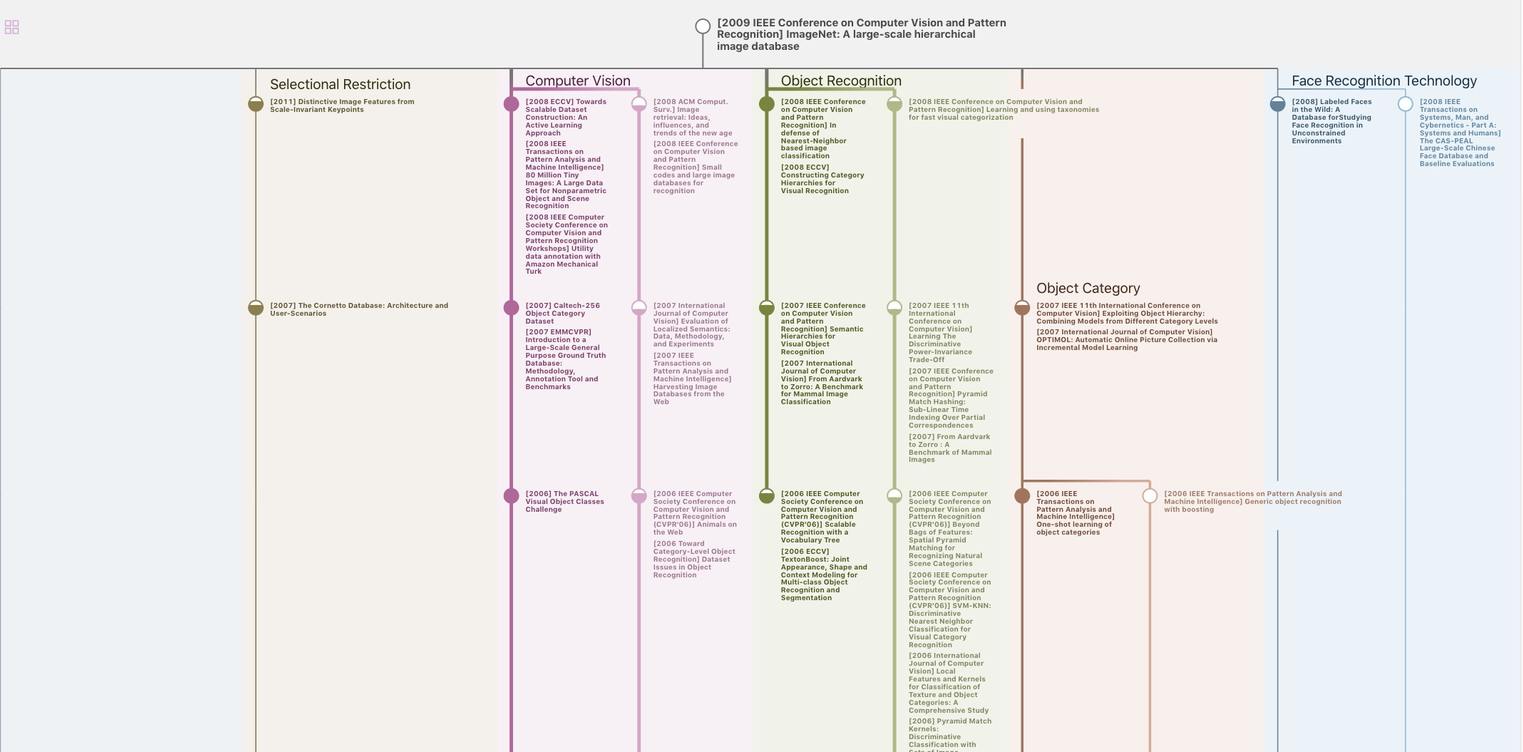
生成溯源树,研究论文发展脉络
Chat Paper
正在生成论文摘要