Temporal-Clustering Based Technique for Identifying Thermal Regions in Buildings.
ACIVS(2020)
摘要
Nowadays, moistures and thermal leaks in buildings are manually detected by an operator, who roughly delimits those critical regions in thermal images. Nevertheless, the use of artificial intelligence (AI) techniques can greatly improve the manual thermal analysis, providing automatically more precise and objective results. This paper presents a temporal-clustering based technique that carries out the segmentation of a set of thermal orthoimages (STO) of a wall, which have been taken at different times. The algorithm has two stages: region labelling and consensus. In order to delimit regions with similar temporal temperature variation, three clustering algorithms are applied on STO, obtaining the respective three labelled images. In the second stage, a consensus algorithm between the labelled images is applied. The method thus delimitates regions with different thermal evolutions over time, each characterized by a temperature consensus vector. The approach has been tested in real scenes by using a 3D thermal scanner. A case study, composed of 48 thermal orthoimages at 30 min-intervals over 24 h, are presented.
更多查看译文
关键词
3D data processing, 3D thermal models, Thermal data analysis
AI 理解论文
溯源树
样例
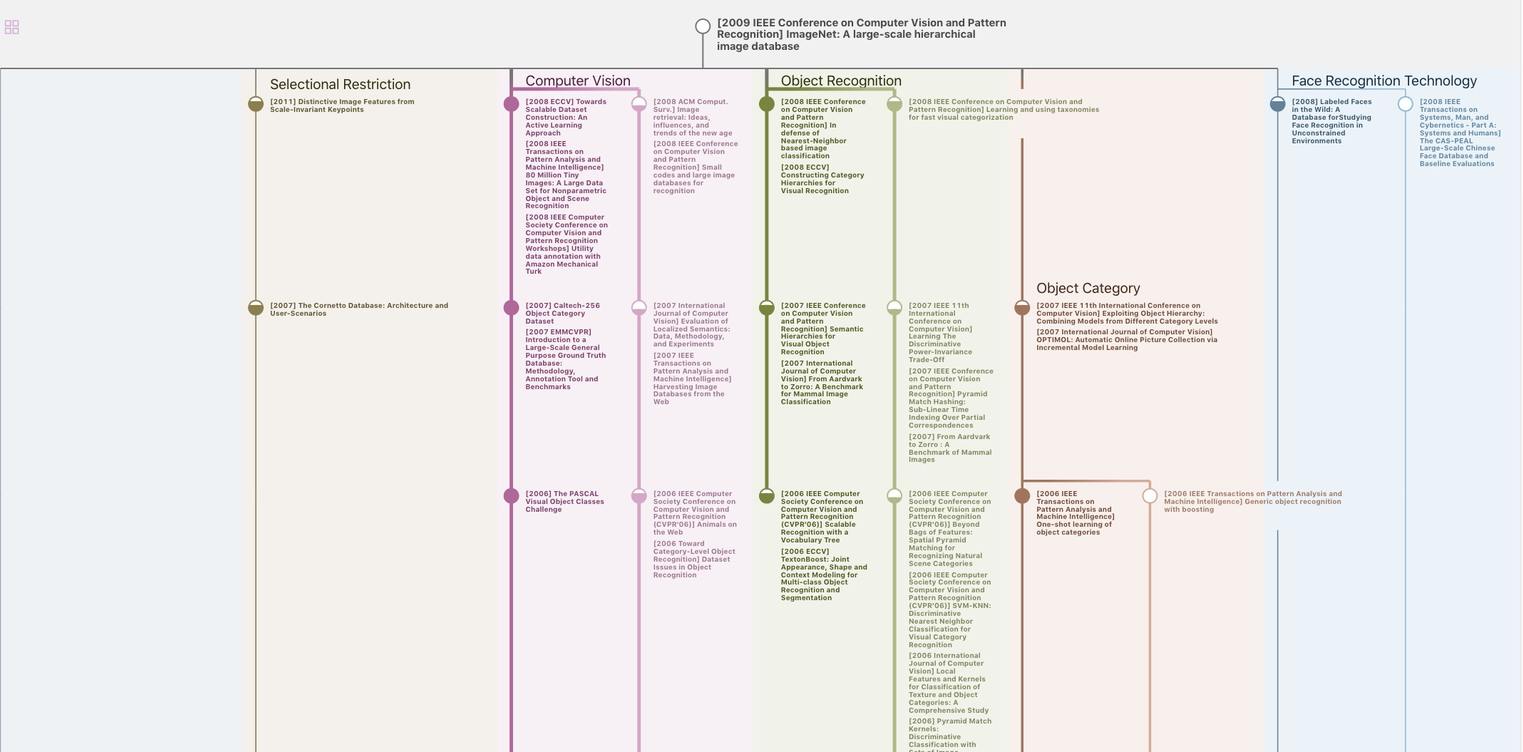
生成溯源树,研究论文发展脉络
Chat Paper
正在生成论文摘要