LRP-Based path relevances for global explanation of deep architectures.
Neurocomputing(2020)
摘要
Understanding what Machine Learning models are doing is not always trivial. This is especially true for complex models such as Deep Neural Networks (DNN), which are the best-suited algorithms for modeling very complex and nonlinear relationships. But this need to understand has become a must since privacy regulations are hardening the industrial use of these models. There are different techniques to address the interpretability issues that Machine Learning models arises. This paper is focused on opening the so-called Deep Neural architectures black-box. This research extends the technique called Layer-wise Relevant Propagation (LRP) enhancing its properties to compute the most critical paths in different deep neural architectures using multicriteria analysis. We call this technique Ranked-LRP and it was tested on four different datasets and tasks, including classification and regression. The results show the worth of our proposal.
更多查看译文
关键词
Explainable AI,Deep learning,Interpretable machine learning,Layer-wise relevant propagation
AI 理解论文
溯源树
样例
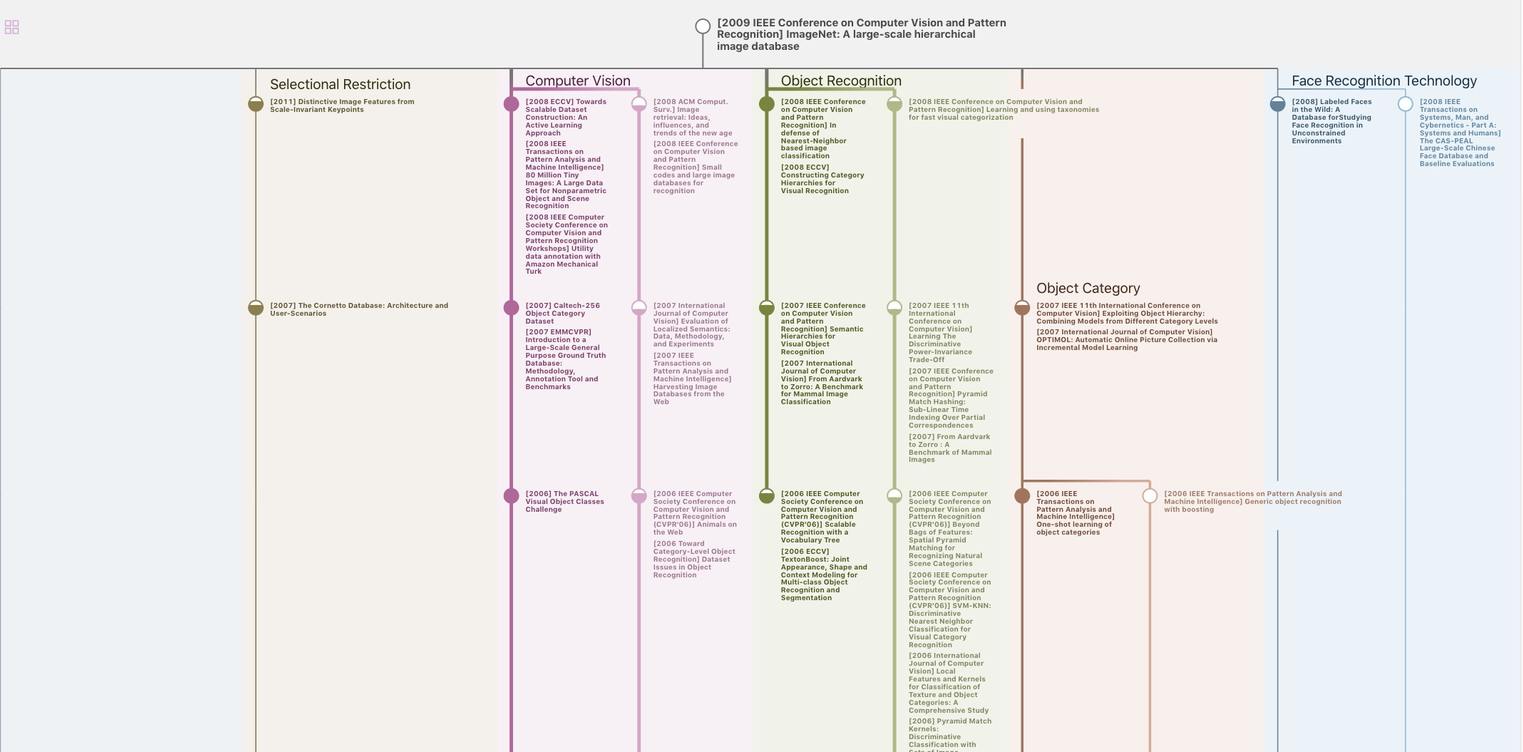
生成溯源树,研究论文发展脉络
Chat Paper
正在生成论文摘要