Bayesian Learning for Classification using a Uniform Dirichlet Prior
2019 IEEE Global Conference on Signal and Information Processing (GlobalSIP)(2019)
摘要
In Bayesian learning, designs based on noninformative priors are appropriate when the user cannot confidently identify the data-generating distribution. While such learners cannot achieve the performance of those based on a well-matched subjective prior, they impart a robustness against poor prior selection. The uniform Dirichlet distribution is the true non-informative prior as it has full support over the space of candidate distributions; additionally, it leads to closed-form posteriors. This work applies such a prior to classification using the 0-1 loss, determines the optimal Bayes classifier and the corresponding minimum probability of error, and analyzes the results.
更多查看译文
关键词
Bayesian learning,machine learning,classification,Dirichlet distribution,predictive distribution
AI 理解论文
溯源树
样例
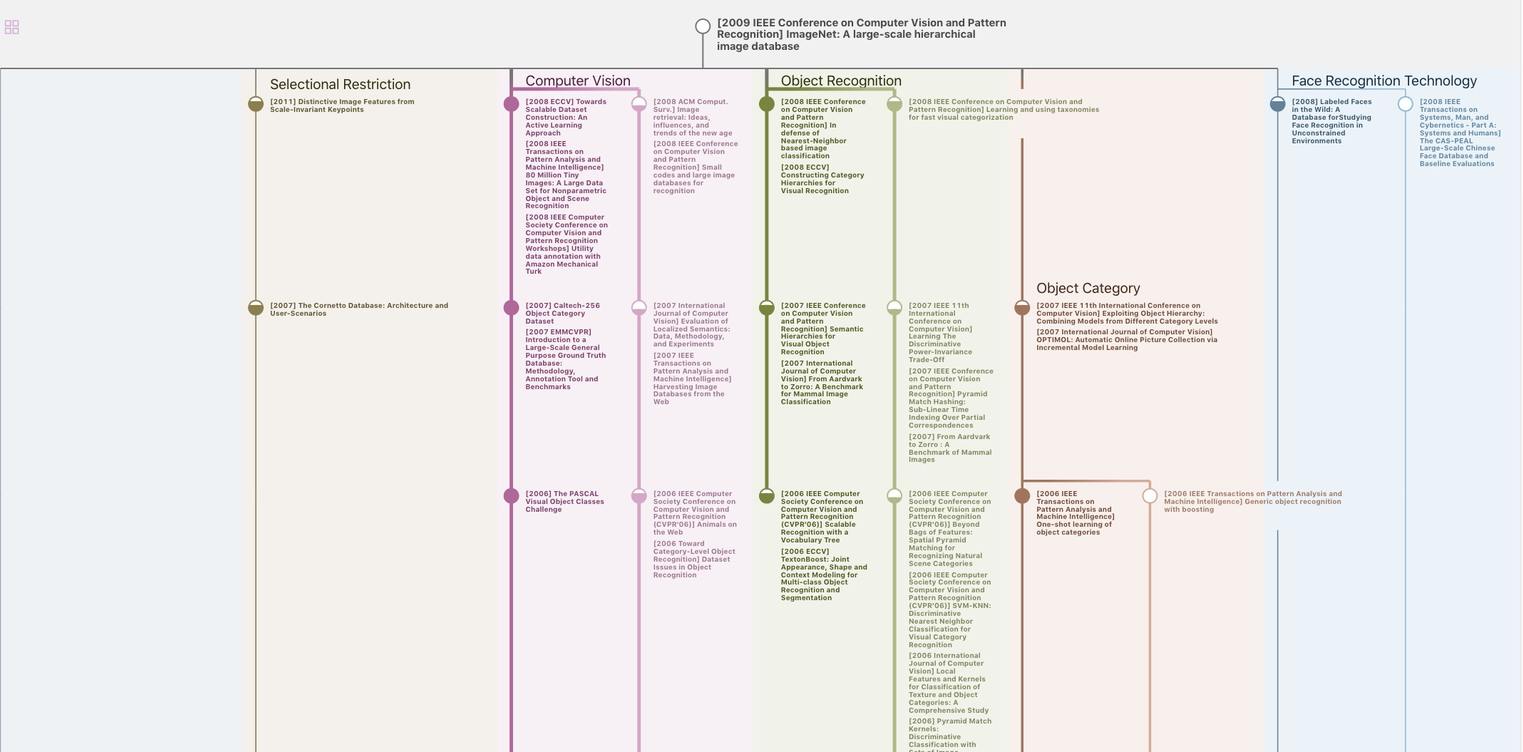
生成溯源树,研究论文发展脉络
Chat Paper
正在生成论文摘要