TrafficPSSF: A Fast and An Effective Malware Detection Under Online and Offline
2018 International Conference on Security, Pattern Analysis, and Cybernetics (SPAC)(2018)
摘要
The use of Android phones is becoming more and more widespread, and Android malware is also entering everyone's field of vision. In this paper, we propose TrafficPSSF as a fast and an effective method for traffic detection and classification under online and offline detection. The traffic collection platform collects traffic data of application. Especially, we design an online detection and offline detection. One of the features of TCP session is the packet size, which is used for online detection. We can detect malicious traffic without waiting for all traffic packets to arrive, which can improve efficiency. What's more, we use combination classifier model for our server to increase the accuracy of malicious detection. In the offline detection, we use seven statistical features of TCP as our model input and random forest algorithm for model training. The experiment shows that the online detection has 98.35% of the malicious detection rate, and the offline detection and classification accuracy rate reach 99.98%.
更多查看译文
关键词
random forest,packet size,statistic feature,malware detection,combination classifier
AI 理解论文
溯源树
样例
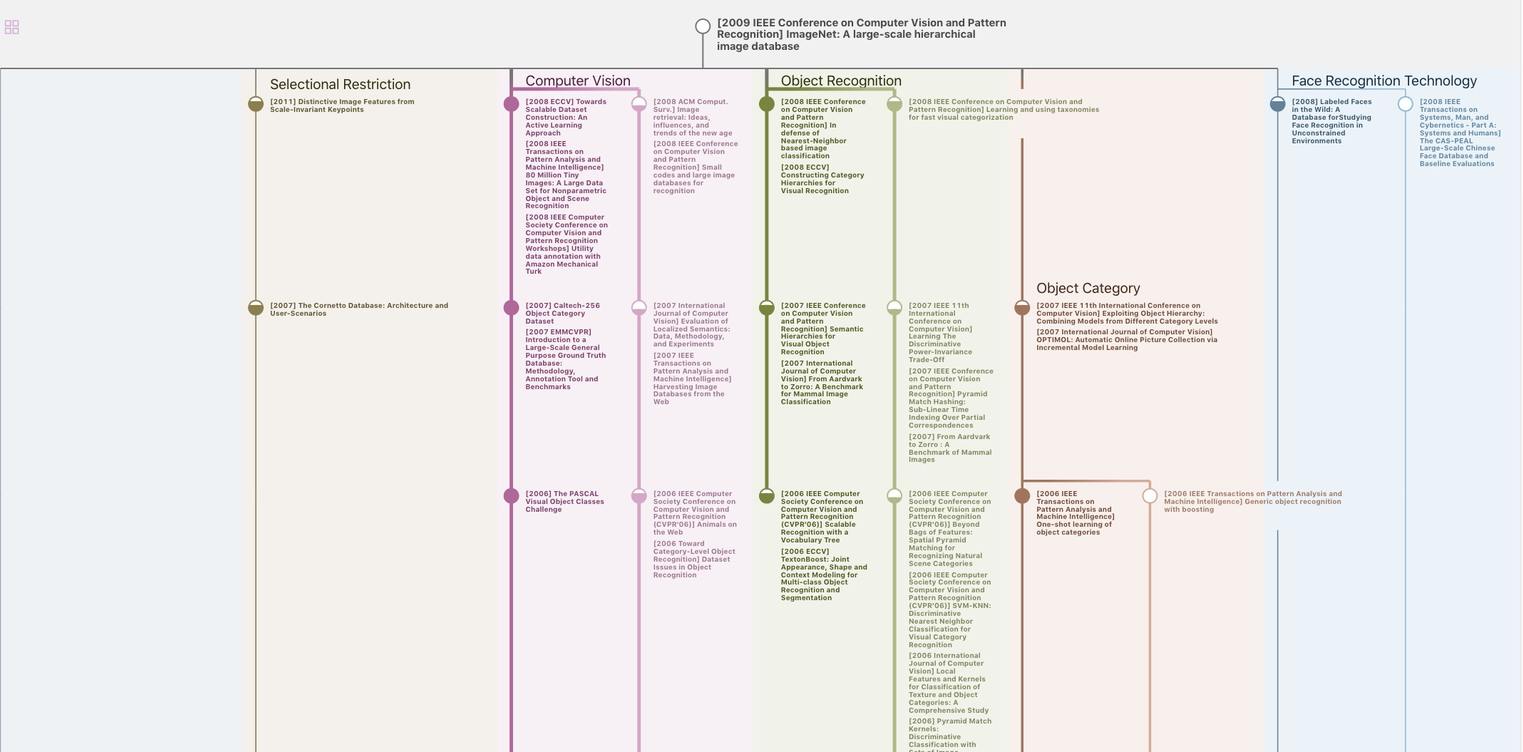
生成溯源树,研究论文发展脉络
Chat Paper
正在生成论文摘要