MEGA: multi-view semi-supervised clustering of hypergraphs
Hosted Content(2020)
摘要
AbstractComplex relationships among entities can be modeled very effectively using hypergraphs. Hypergraphs model real-world data by allowing a hyperedge to include two or more entities. Clustering of hypergraphs enables us to group the similar entities together. While most existing algorithms solely consider the connection structure of a hypergraph to solve the clustering problem, we can boost the clustering performance by considering various features associated with the entities as well as auxiliary relationships among the entities. Also, we can further improve the clustering performance if some of the labels are known and we incorporate them into a clustering model. In this paper, we propose a semi-supervised clustering framework for hypergraphs that is able to easily incorporate not only multiple relationships among the entities but also multiple attributes and content of the entities from diverse sources. Furthermore, by showing the close relationship between the hypergraph normalized cut and the weighted kernel K-Means, we also develop an efficient multilevel hypergraph clustering method which provides a good initialization with our semi-supervised multi-view clustering algorithm. Experimental results show that our algorithm is effective in detecting the ground-truth clusters and significantly outperforms other state-of-the-art methods.
更多查看译文
AI 理解论文
溯源树
样例
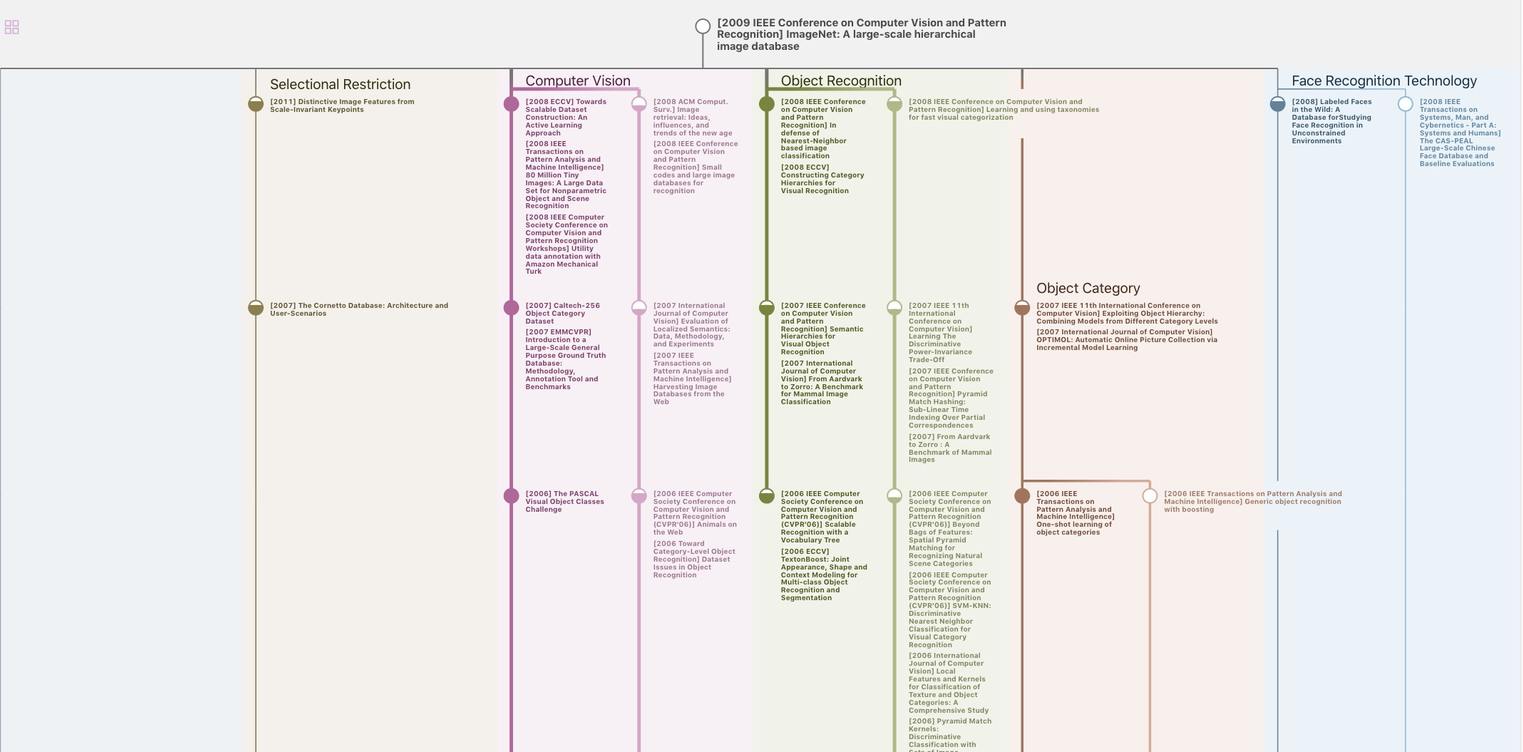
生成溯源树,研究论文发展脉络
Chat Paper
正在生成论文摘要