Direct Estimation of Forest Leaf Area Index based on Spectrally Corrected Airborne LiDAR Pulse Penetration Ratio.
REMOTE SENSING(2020)
摘要
The leaf area index (LAI) is a crucial structural parameter of forest canopies. Light Detection and Ranging (LiDAR) provides an alternative to passive optical sensors in the estimation of LAI from remotely sensed data. However, LiDAR-based LAI estimation typically relies on empirical models, and such methods can only be applied when the field-based LAI data are available. Compared with an empirical model, a physically-based model-e.g., the Beer-Lambert law based light extinction model-is more attractive due to its independent dataset with training. However, two challenges are encountered when applying the physically-based model to estimate LAI from discrete LiDAR data: i.e., deriving the gap fraction and the extinction coefficient from the LiDAR data. We solved the first problem by integrating LiDAR and hyperspectral data to transfer the LiDAR penetration ratio to the forest gap fraction. For the second problem, the extinction coefficient was estimated from tiled (1 km x 1 km) LiDAR data by nonlinearly optimizing the cost function of the angular LiDAR gap fraction and simulated gap fraction from the Beer-Lambert law model. A validation against LAI-2000 measurements showed that the estimates were significantly correlated to the reference LAI with an R-2 of 0.66, a root mean square error (RMSE) of 0.60 and a relative RMSE of 0.15. We conclude that forest LAI can be directly estimated by the nonlinear optimization method utilizing the Beer-Lambert model and a spectrally corrected LiDAR penetration ratio. The significance of the proposed method is that it can produce reliable remotely sensed forest LAI from discrete LiDAR and spectral data when field-measured LAI are unavailable.
更多查看译文
关键词
leaf area index,Light Detection and Ranging (LiDAR),gap fraction,extinction coefficient,spectral correction
AI 理解论文
溯源树
样例
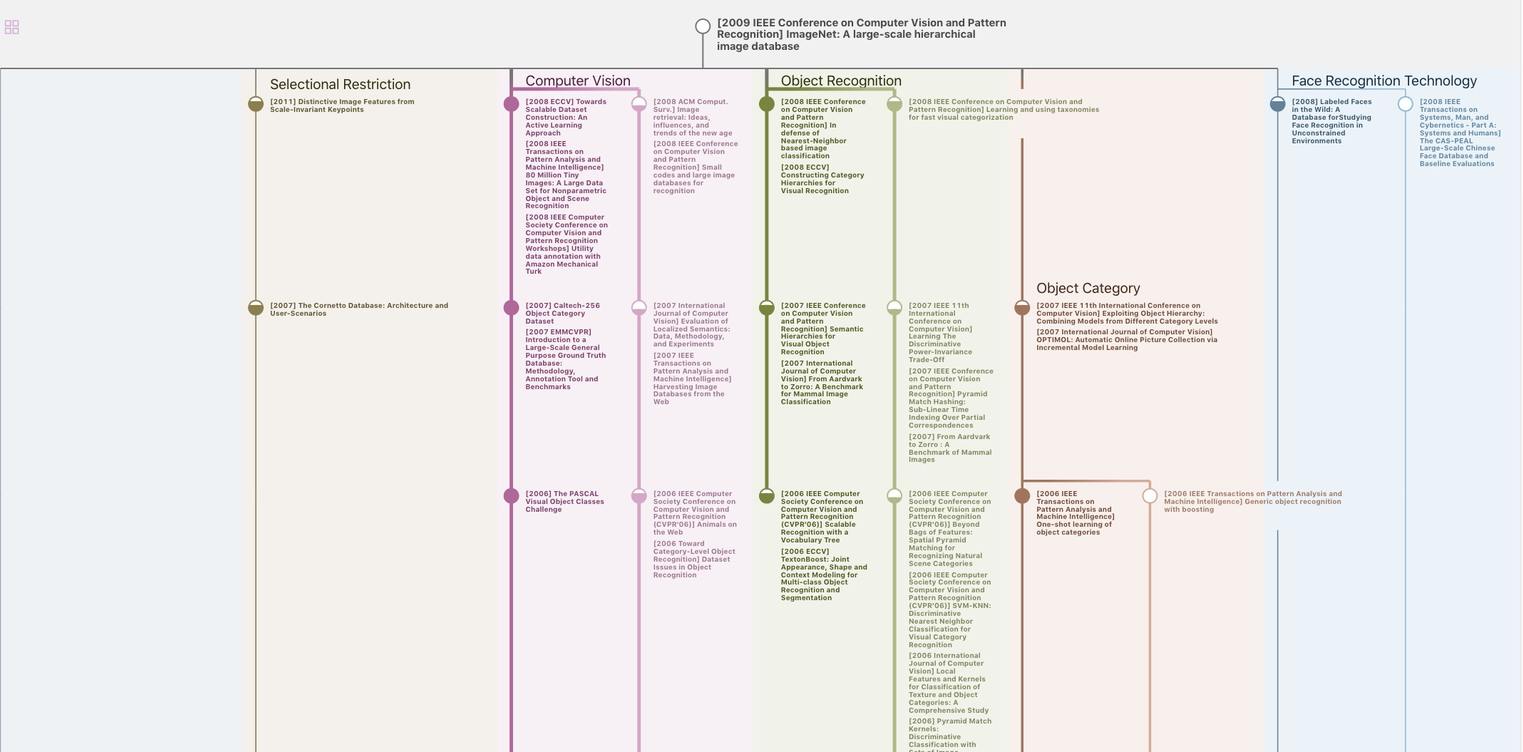
生成溯源树,研究论文发展脉络
Chat Paper
正在生成论文摘要