Speeding up training of automated bird recognizers by data reduction of audio features.
PEERJ(2020)
摘要
Automated acoustic recognition of birds is considered an important technology in support of biodiversity monitoring and biodiversity conservation activities. These activities require processing large amounts of soundscape recordings. Typically, recordings are transformed to a number of acoustic features, and a machine learning method is used to build models and recognize the sound events of interest. The main problem is the scalability of data processing, either for developing models or for processing recordings made over long time periods. In those cases, the processing time and resources required might become prohibitive for the average user. To address this problem, we evaluated the applicability of three data reduction methods. These methods were applied to a series of acoustic feature vectors as an additional postprocessing step, which aims to reduce the computational demand during training. The experimental results obtained using Mel-frequency cepstral coefficients (MFCCs) and hidden Markov models (HMMs) support the finding that a reduction in training data by a factor of 10 does not significantly affect the recognition performance.
更多查看译文
关键词
Data representation,Data reduction,Random sampling,Uniform sampling,Piecewise aggregate approximation
AI 理解论文
溯源树
样例
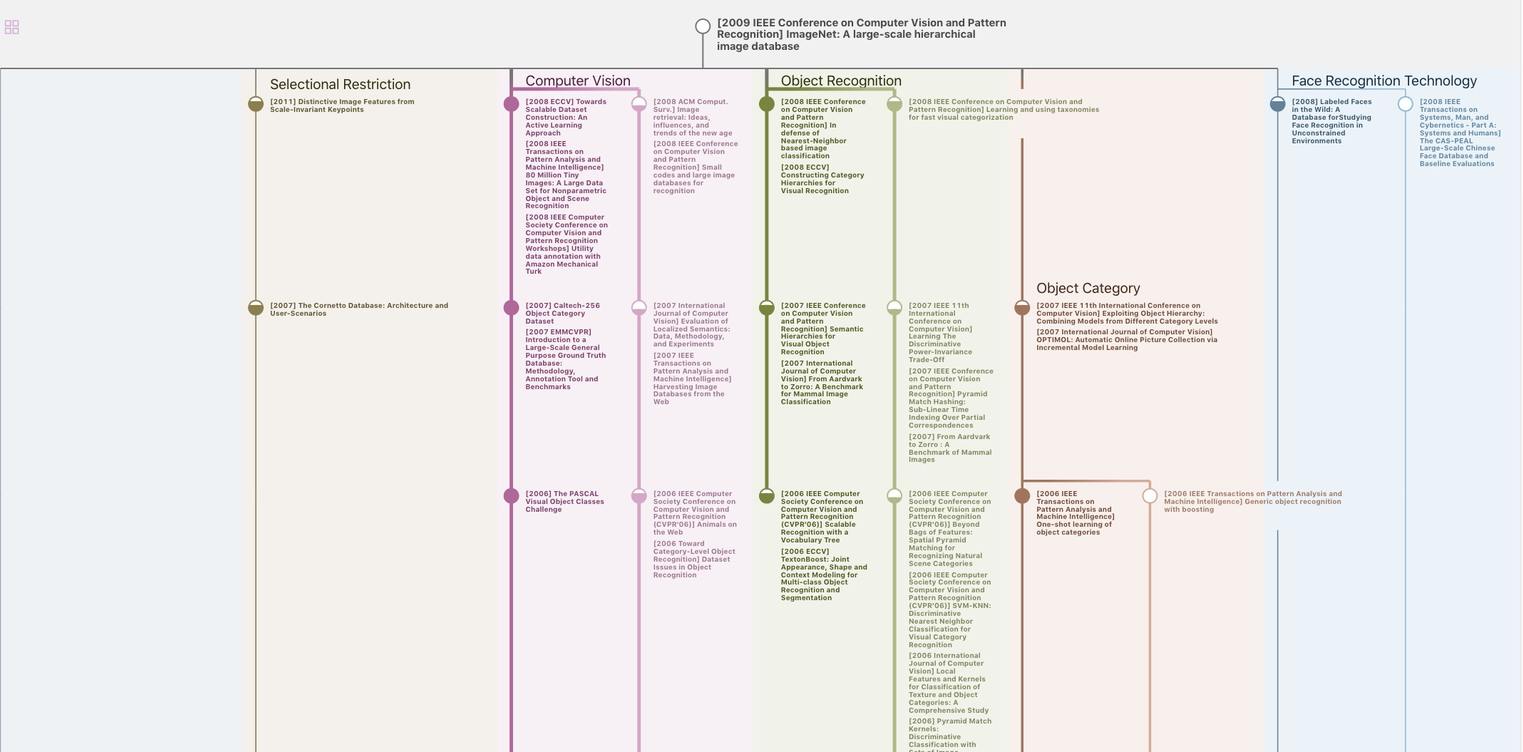
生成溯源树,研究论文发展脉络
Chat Paper
正在生成论文摘要