A deep learning model to predict lower temperatures in agriculture.
JOURNAL OF AMBIENT INTELLIGENCE AND SMART ENVIRONMENTS(2020)
摘要
Deep learning techniques provide a novel framework for prediction and classification in decision-making procedures that are widely applied in different fields. Precision agriculture is one of these fields where the use of decision-making technologies provides better production with better costs and a greater benefit for farmers. This paper develops an intelligent framework based on a deep learning model for early prediction of crop frost to help farmers activate anti-frost techniques to save the crop. This model is based on a long short-term memory (LSTM) model and it is designed to predict low temperatures. The model is based on information from an IoT infrastructure deployed on two plots in Murcia (Southeast of Spain). Three experiments are performed; a cross validation to validate the model from the most pessimistic point of view, a validation of 24 consecutive hours of temperatures, in order to know 24 hours before the possible temperature drop and a comparison with two traditional time series prediction techniques, namely Auto Regressive Integrated Moving Average and the Gaussian process. The results obtained are satisfactory, being better the results of the LSTM, obtaining an average quadratic error of less than a Celsius degree and a determination coefficient R-2 greater than 0.95.
更多查看译文
关键词
Deep Learning,LSTM,precision agriculture,IoT
AI 理解论文
溯源树
样例
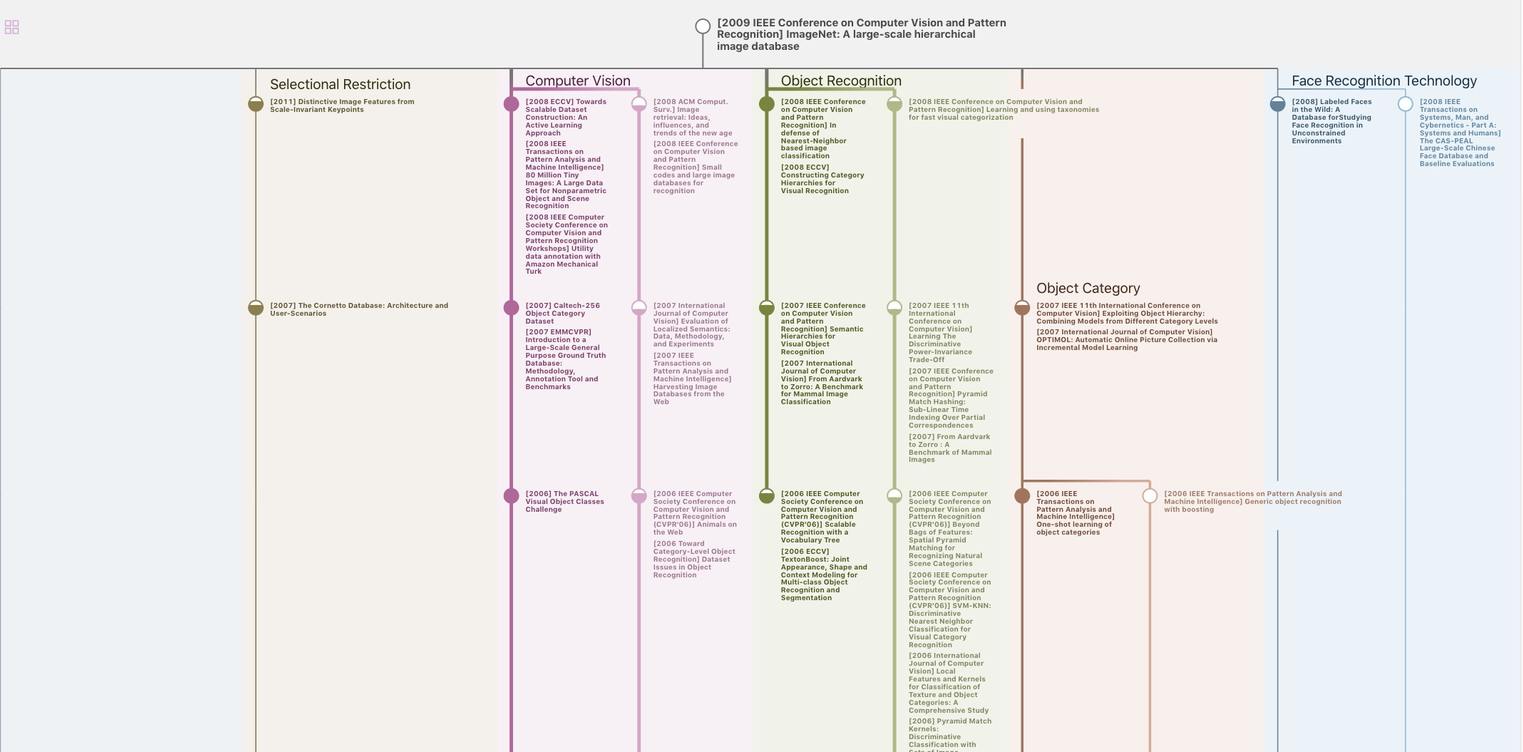
生成溯源树,研究论文发展脉络
Chat Paper
正在生成论文摘要