{P}Net: Privacy-Preserving Personalization Of Ai-Based Models By Anonymous Inter-Person Similarity Networks
PROCEEDINGS OF THE 16TH EAI INTERNATIONAL CONFERENCE ON MOBILE AND UBIQUITOUS SYSTEMS: COMPUTING, NETWORKING AND SERVICES (MOBIQUITOUS'19)(2019)
摘要
Emerging proactive applications need user data to power their underlying AI algorithms. However, both the training and inference tasks are typically performed in the provider's cloud, leading to multiple privacy issues to the data subject. Current privacy-preserving concepts are neither practicable for AI algorithms nor promise mutual benefits for both parties. To address these issues, we propose {P}Net-a novel two-level approach for privacy-preserving personalization, which exploits 'divisible' AI algorithms and anonymous inter-person similarity measurements. In short, {P}Net splits the training task into a cloud-based general model learning process and 'local' personalization steps in which the general 'black-box' model is subsequently adapted to individuals (level 1). Based on anonymously-contributed model modifications (patches) resulting from the first level, {P}Net further allows new users to request a community model-representing the general model personalized by crowd-sourced patches from other similar users (level 2). Our experiments show that {P}Net outperforms existing techniques especially when only a few user data is labeled. With {P}Net, new users can now directly benefit from personalized applications in a practical and privacy-preserving way with reduced labeling effort.
更多查看译文
关键词
Divisible AI Algorithms, Patching, Community Learning, Personal Model, Privacy, Anonymous Communication
AI 理解论文
溯源树
样例
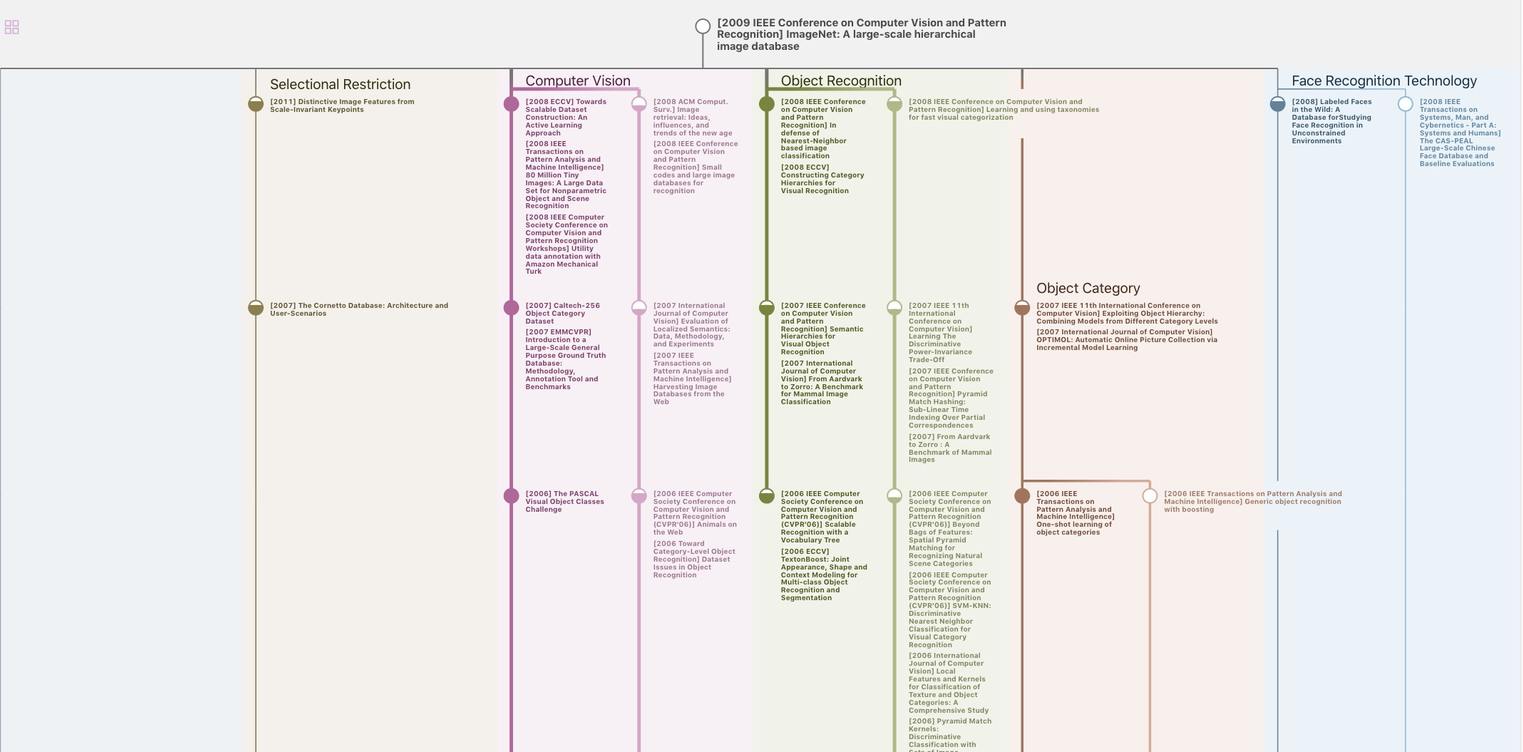
生成溯源树,研究论文发展脉络
Chat Paper
正在生成论文摘要