Temporal-adaptive Hierarchical Reinforcement Learning
arxiv(2020)
摘要
Hierarchical reinforcement learning (HRL) helps address large-scale and sparse reward issues in reinforcement learning. In HRL, the policy model has an inner representation structured in levels. With this structure, the reinforcement learning task is expected to be decomposed into corresponding levels with sub-tasks, and thus the learning can be more efficient. In HRL, although it is intuitive that a high-level policy only needs to make macro decisions in a low frequency, the exact frequency is hard to be simply determined. Previous HRL approaches often employed a fixed-time skip strategy or learn a terminal condition without taking account of the context, which, however, not only requires manual adjustments but also sacrifices some decision granularity. In this paper, we propose the \emph{temporal-adaptive hierarchical policy learning} (TEMPLE) structure, which uses a temporal gate to adaptively control the high-level policy decision frequency. We train the TEMPLE structure with PPO and test its performance in a range of environments including 2-D rooms, Mujoco tasks, and Atari games. The results show that the TEMPLE structure can lead to improved performance in these environments with a sequential adaptive high-level control.
更多查看译文
关键词
reinforcement,hierarchical,learning,temporal-adaptive
AI 理解论文
溯源树
样例
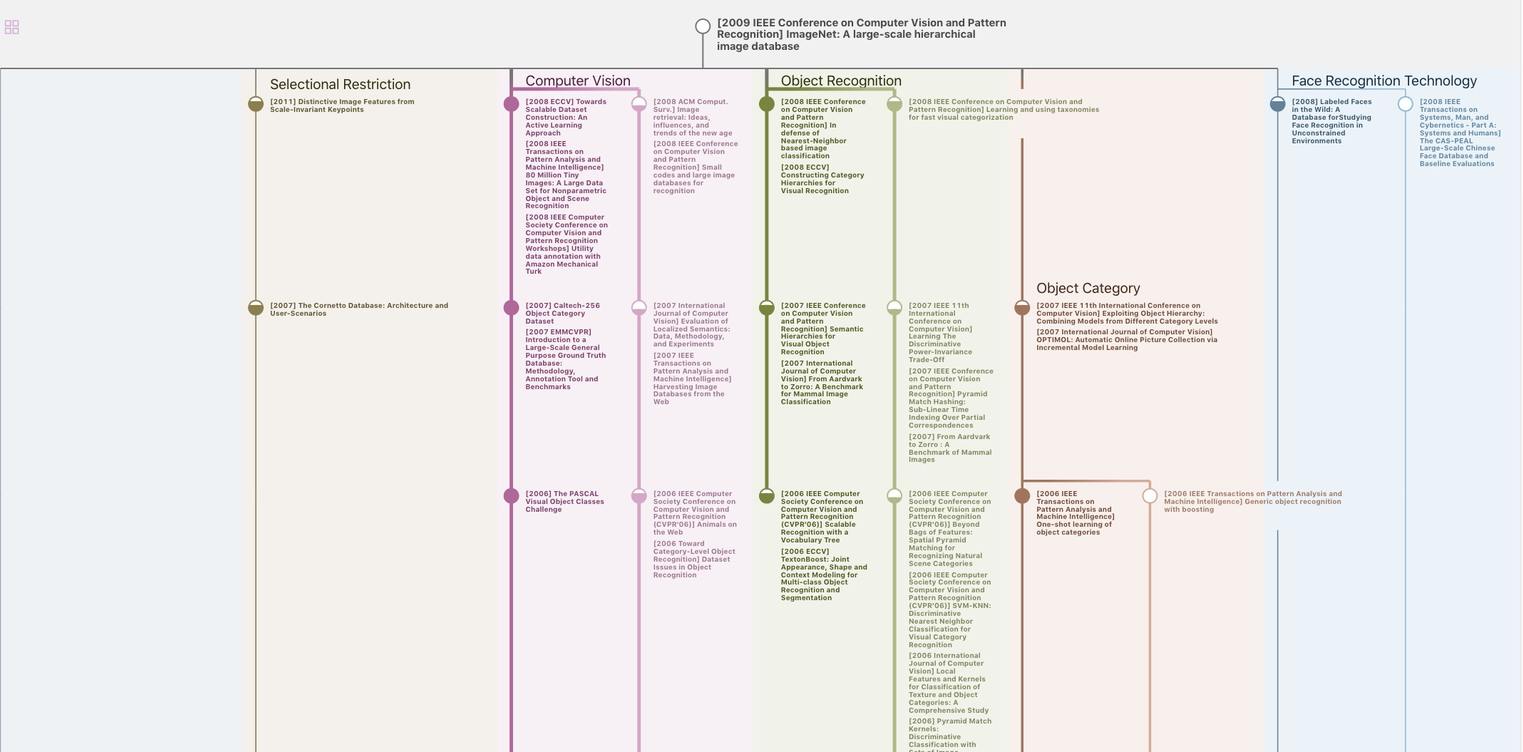
生成溯源树,研究论文发展脉络
Chat Paper
正在生成论文摘要