Contextual Stroke Classification in Online Handwritten Documents with Graph Attention Networks.
ICDAR(2019)
摘要
Classifying strokes into different categories is an essential preprocessing step in the automatic document understanding process. To tackle this task, it is crucial to integrate different types of contextual information. Previous methods which are based on conditional random fields or recurrent neural networks have some limitations in model capacity or computational cost. In this paper, we propose a novel framework based on graph attention networks to solve this problem, which casts the stroke classification problem into the node classification problem in a document graph. In the graph, each node represents a stroke and the edges are built from temporal and spatial interactions between strokes. Combined graph convolution with attention mechanisms to dynamically aggregate features from the neighborhood, our model is very flexible to control the message passing routine between different nodes and therefore has strong capability learning context-aware features. We perform comparison experiments on the IAMonDo dataset and experimental results demonstrate the superiority of our approach.
更多查看译文
关键词
online handwritten document, stroke classification, graph attention networks, structured prediction
AI 理解论文
溯源树
样例
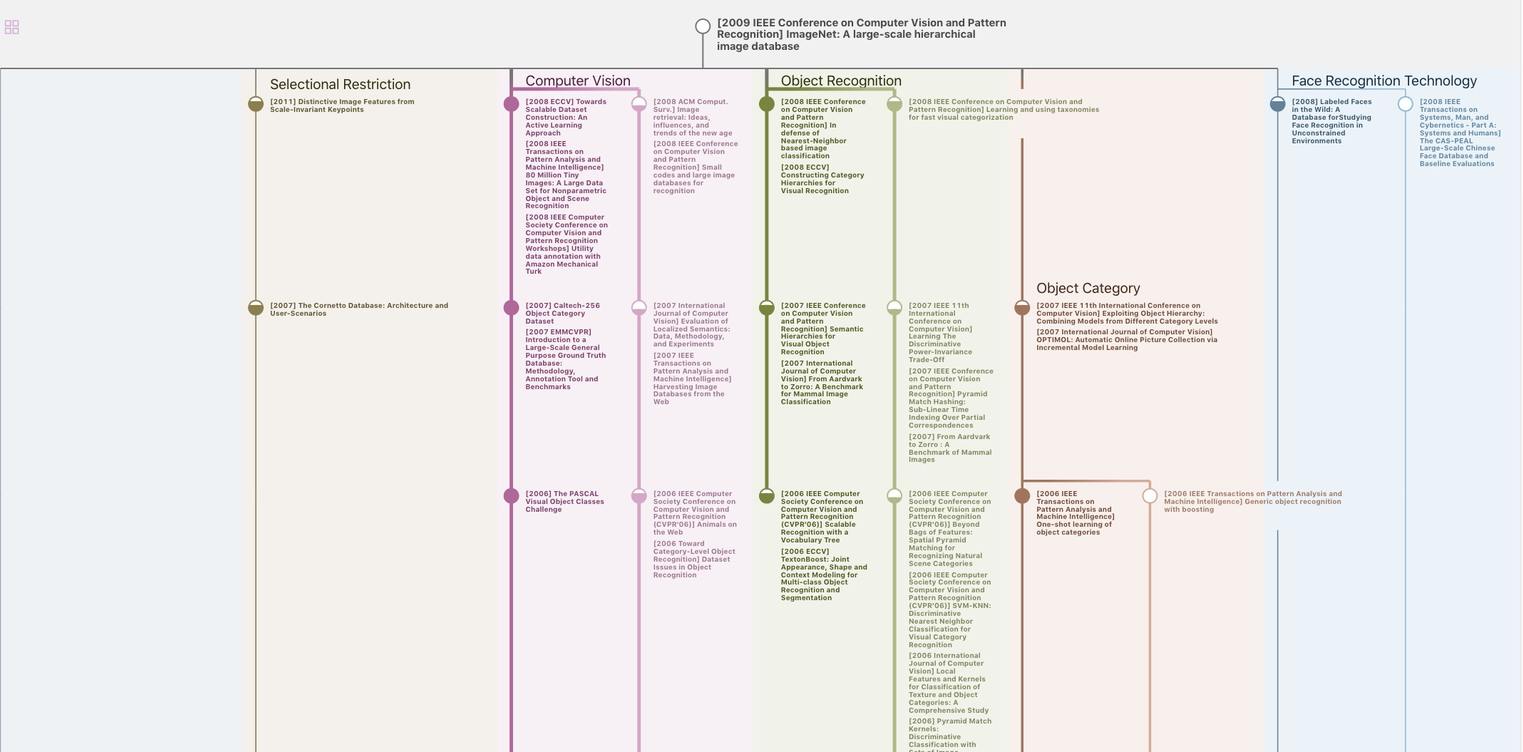
生成溯源树,研究论文发展脉络
Chat Paper
正在生成论文摘要