A PRECISE HIGH-DIMENSIONAL ASYMPTOTIC THEORY FOR BOOSTING AND MINIMUM-l(1)-NORM INTERPOLATED CLASSIFIERS
arxiv(2022)
摘要
This paper establishes a precise high-dimensional asymptotic theory for boosting on separable data, taking statistical and computational perspectives. We consider a high-dimensional setting where the number of features (weak learners) p scales with the sample size n, in an overparametrized regime. Under a class of statistical models, we provide an exact analysis of the generalization error of boosting when the algorithm interpolates the training data and maximizes the empirical l(1)-margin. Further, we explicitly pin down the relation between the boosting test error and the optimal Bayes error, as well as the proportion of active features at interpolation (with zero initialization). In turn, these precise characterizations answer certain questions raised in (Neural Comput. 11 (1999) 1493-1517; Ann. Statist. 26 (1998) 1651-1686) surrounding boosting, under assumed data generating processes. At the heart of our theory lies an in-depth study of the maximum-l(1)-margin, which can be accurately described by a new system of nonlinear equations; to analyze this margin, we rely on Gaussian comparison techniques and develop a novel uniform deviation argument. Our statistical and computational arguments can handle (1) any finite-rank spiked covariance model for the feature distribution and (2) variants of boosting corresponding to general l(q)-geometry, q is an element of [1, 2]. As a final component, via the Lindeberg principle, we establish a universality result showcasing that the scaled l(1)-margin (asymptotically) remains the same, whether the covariates used for boosting arise from a nonlinear random feature model or an appropriately linearized model with matching moments.
更多查看译文
关键词
Boosting, high-dimensional asymptotics, minimum-norm interpolation, over-parametrization, margin theory
AI 理解论文
溯源树
样例
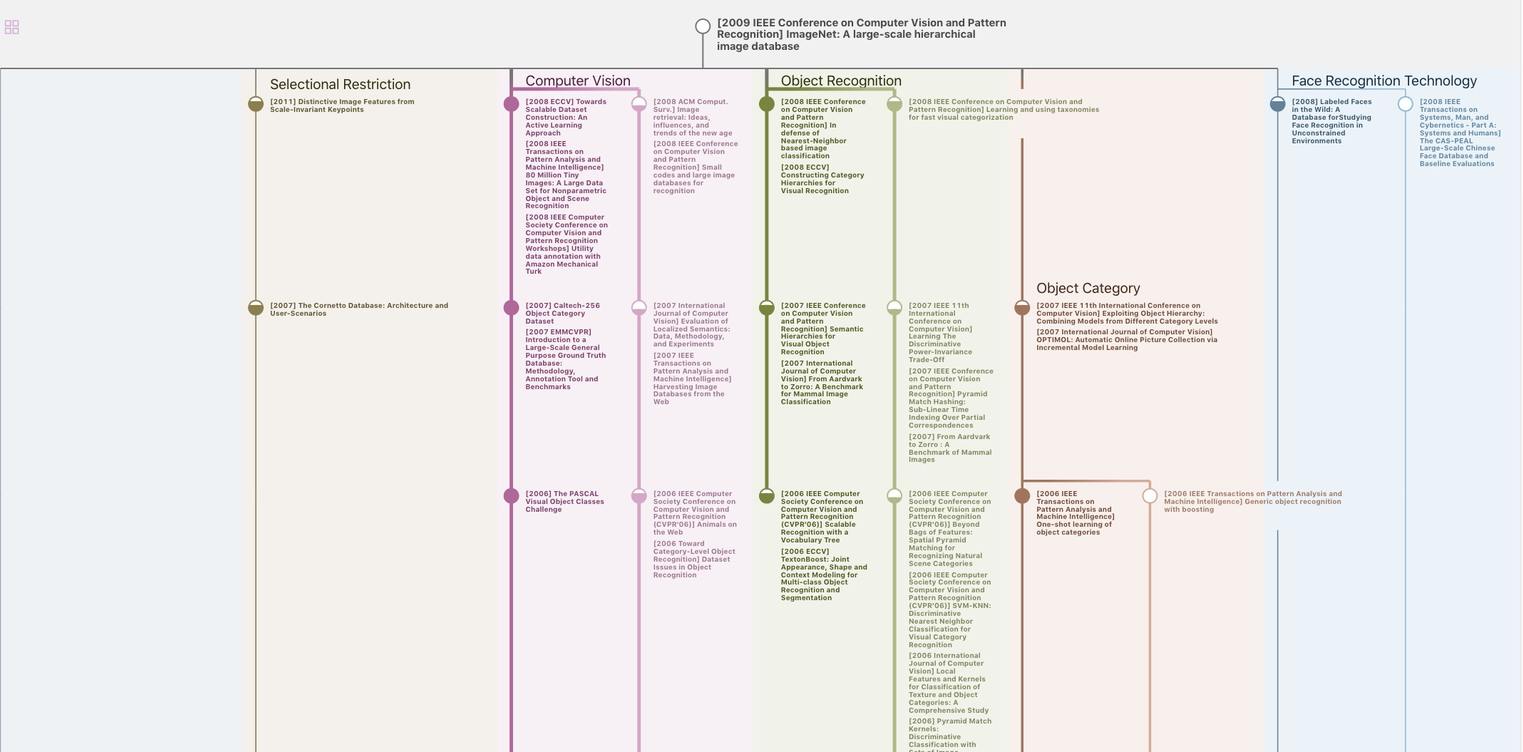
生成溯源树,研究论文发展脉络
Chat Paper
正在生成论文摘要