A Deep ConvNet-Based Countermeasure to Mitigate Link Flooding Attacks Using Software-Defined Networks.
ISCC(2019)
摘要
Recently, link flooding attacks (LFA) have been observed as a serious threat for cutting off the Internet connectivity through congesting critical links. A LFA typically utilizes legitimate and low-rate flows, which makes it extremely hard to be detected and, subsequently, to be mitigated. In this paper, we present LF-Shield, that is a deep convolutional neural network (ConvNet) based countermeasure to accurately detect and efficiently mitigate LFAs using software-defined network (SDN) paradigm. LF-Shield can identify malicious bots that launch LFA flows by extracting end-hosts’ traffic features and afterwards, classifying the type of end-hosts based on deep ConvNet. Then, LF-Shield mitigates LFAs without affecting legitimate end-hosts through blocking the classified malicious bots and limiting the bandwidths of inactive or newly-accessed end-hosts. A LF-Shield prototype is implemented for evaluating its performance by several experiments. The experimental results demonstrate that LF-Shield can identify malicious bots with an accuracy of 96.4% and mitigate LFAs with the 93.1% reduction in link degradation ratio, with negligible impact on legitimate end-hosts.
更多查看译文
关键词
Software-defined network,link flooding attack,deep convolutional neural network.
AI 理解论文
溯源树
样例
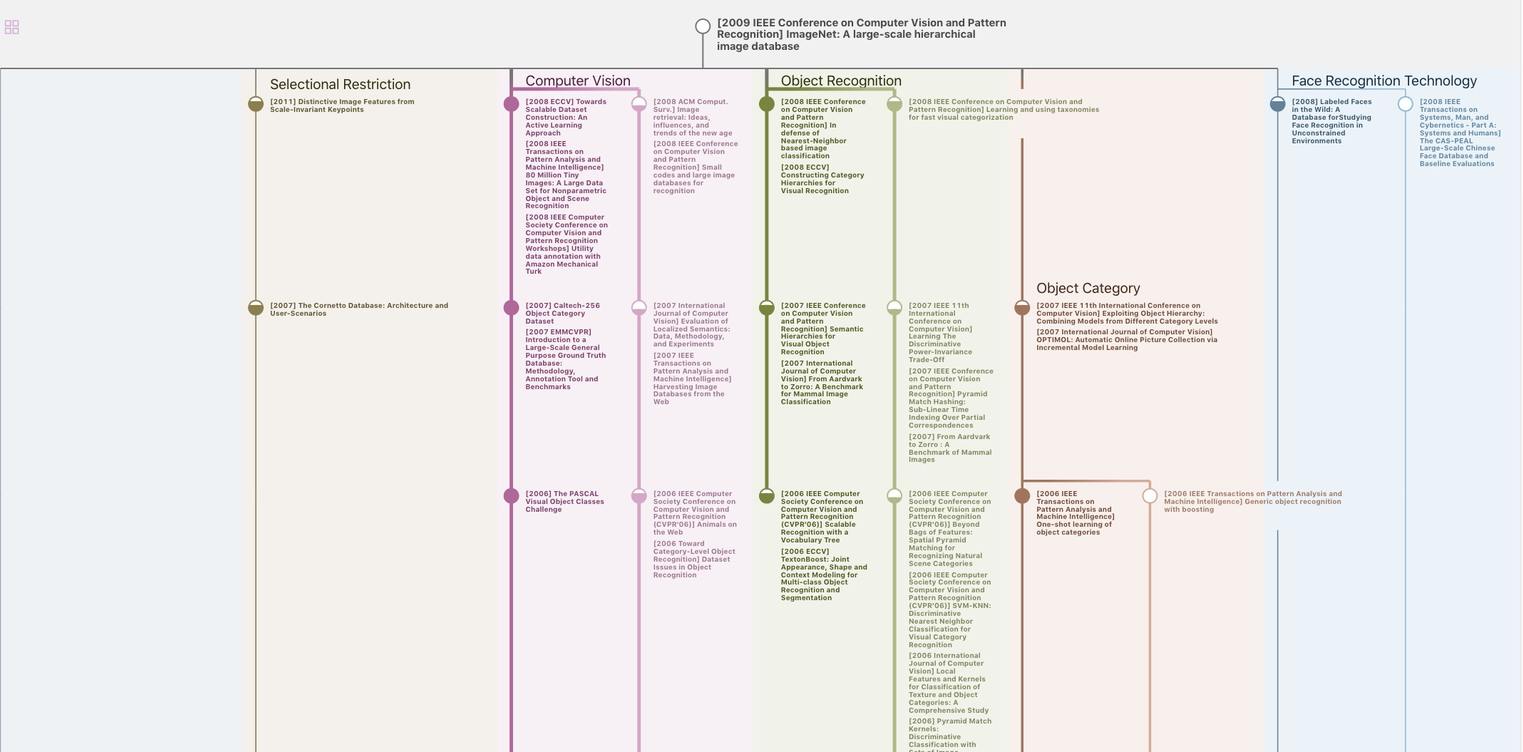
生成溯源树,研究论文发展脉络
Chat Paper
正在生成论文摘要