Knowrisk: An Interpretable Knowledge-Guided Model For Disease Risk Prediction
2019 19TH IEEE INTERNATIONAL CONFERENCE ON DATA MINING (ICDM 2019)(2019)
摘要
Thanks to the widespread adoption of Electronic Health Record (EHR) systems, a variety of data-driven clinical risk prediction approaches have been spawned in recent years. However, there remain three challenges, which if addressed would improve the performance and applicability of such models. (i) Due to the limited data sharing between different health care institutions, the EHR data collected by a single institution is often inadequate or missing some visits records. The limited number of data cannot meet the large sample required of recent approaches especially deep learning models. In addition, the missing records (due to visiting different institution) may contain important health condition of the patient, which if ignored would cause prediction bias. (ii) Few existing approaches take clinical knowledge into account. The auxiliary knowledge if included can greatly reduce the data dependency of many modern learning algorithms. (iii) Most existing deep learning based methods are unable to identify the contribution of each medical event to the final results, which prohibits such models from being widely accepted in practical clinical applications. In this paper, we propose an interpretable and knowledge-guided deep model to address these challenges. Specifically, we distill knowledge from a clinical knowledge graph both explicitly and implicitly, which can not only supplement inadequate patient records but also guide the predicting process of the model. Furthermore, skip-connections and attention mechanisms are adopted to improve the interpretability of our model. In the context of heart failure prediction task, our model outperforms several state-of-the-art methods. Finally, a series of case studies are presented to prove the interpretability of our model.
更多查看译文
关键词
Risk Prediction, Deep Learning, Interpretability, Knowledge Graph
AI 理解论文
溯源树
样例
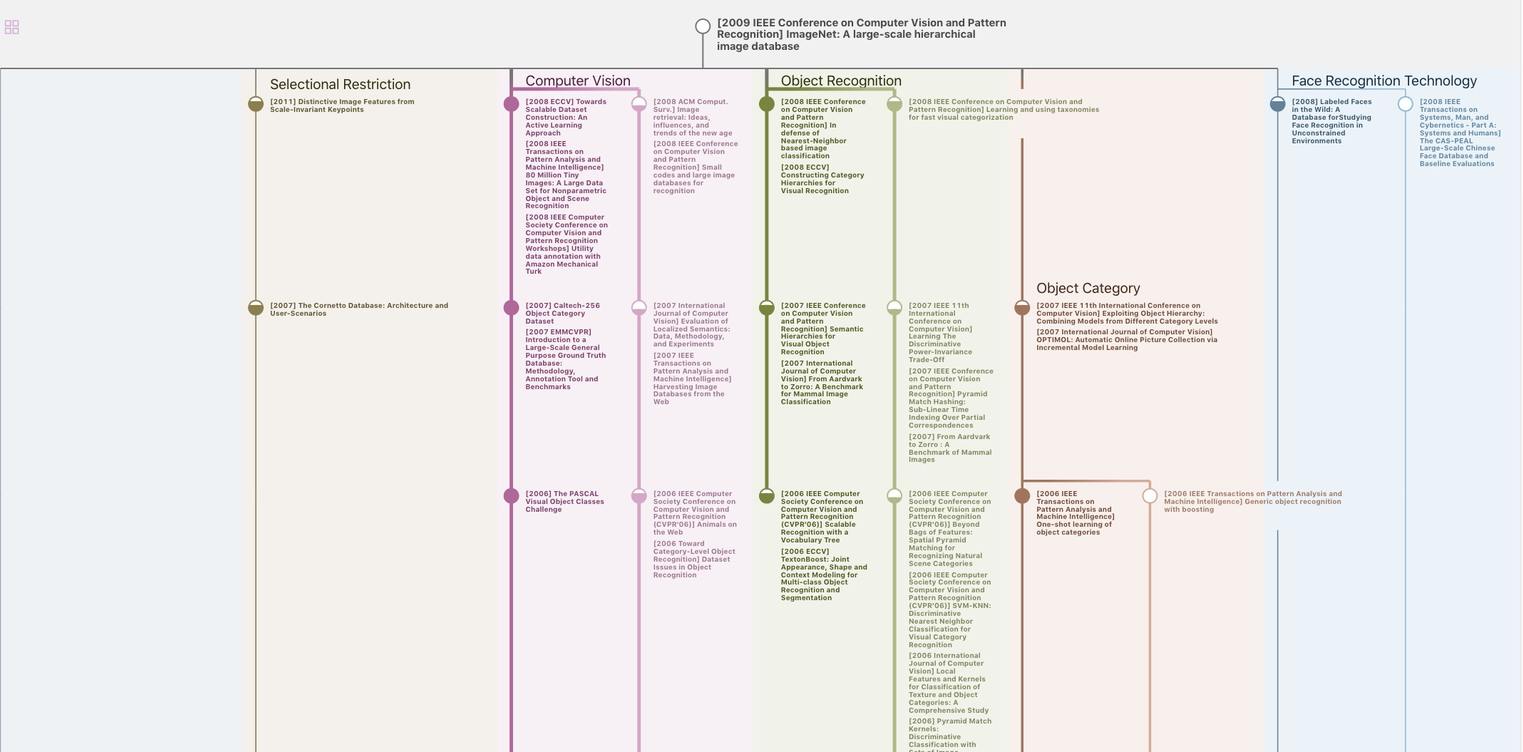
生成溯源树,研究论文发展脉络
Chat Paper
正在生成论文摘要