Dynamic News Recommendation With Hierarchical Attention Network
2019 19TH IEEE INTERNATIONAL CONFERENCE ON DATA MINING (ICDM 2019)(2019)
摘要
News recommendation is an effective information dissemination solution in modern society. In general, news articles can be modeled from multiple granularities: sentence-, element- and news-level. However, the first two levels have been largely ignored in existing methods and it is also unclear how such multi-granularity modeling can enhance news recommendation. In this paper, we propose a novel dynamic model for news recommendation. A unique perspective of our model is to discriminate the contributions of previously interacted contents for triggering the next news-reading, in sentence-, element- and news-level simultaneously. To this end, we design a hierarchical attention network of which the lower layer learns the impacts of sentences and elements, while the upper layer captures disparity of news. Moreover, we incorporate a time-decaying factor to reflect the dynamism, as well as convolution neural networks for learning sequential influence. Using three real-world datasets, we conduct extensive experiments to verify the superiority of our model, compared with several state-of-the-art approaches.
更多查看译文
关键词
news recommendation, attention model, dynamic model, convolutional neural networks
AI 理解论文
溯源树
样例
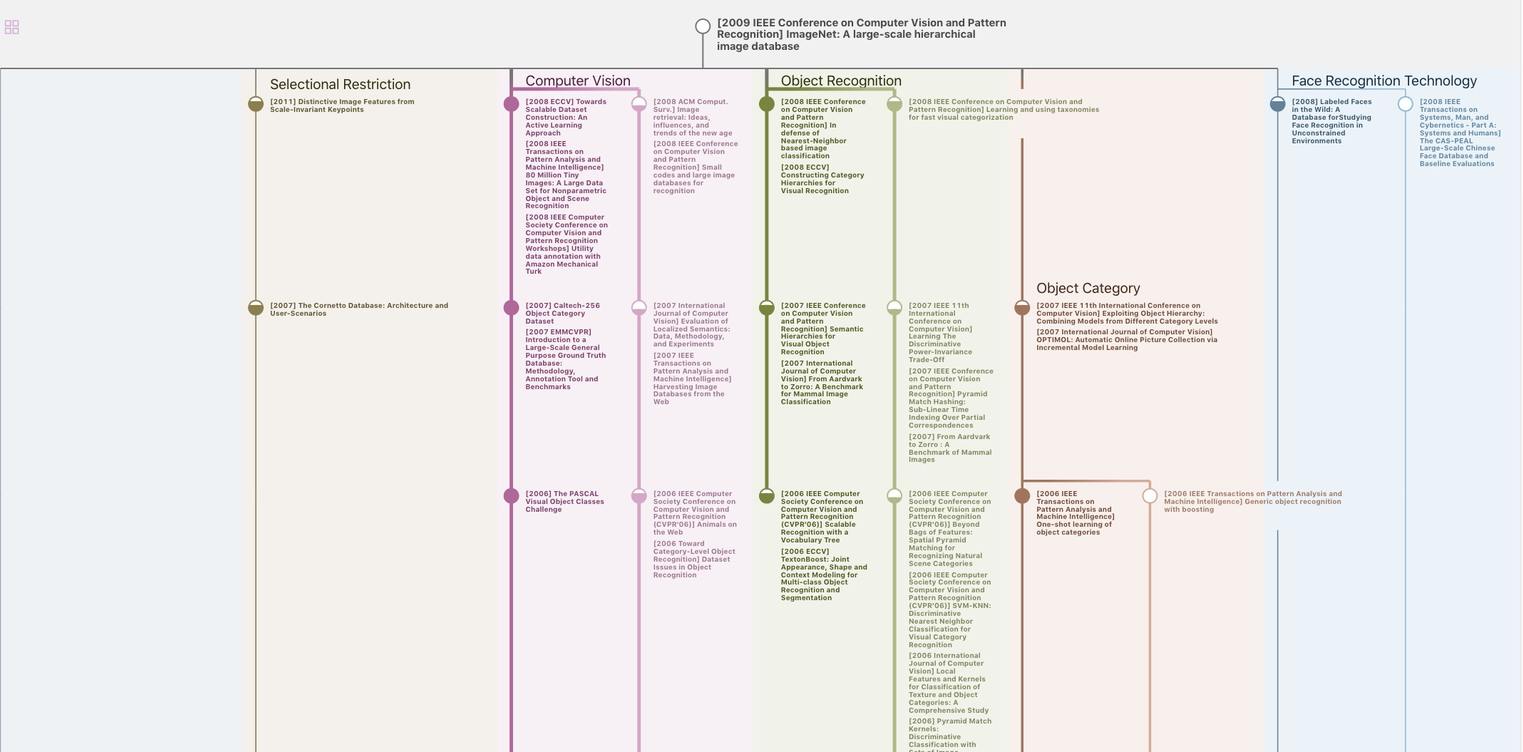
生成溯源树,研究论文发展脉络
Chat Paper
正在生成论文摘要