Dual Adversarial Learning Based Network Alignment
2019 19TH IEEE INTERNATIONAL CONFERENCE ON DATA MINING (ICDM 2019)(2019)
摘要
Network alignment, which aims to learn a matching between the same entities across multiple information networks, often suffers challenges from feature inconsistency, high-dimensional features, to unstable alignment results. This paper presents a novel network alignment framework, RANA, that combines dual generative adversarial network (GAN) techniques to match the distributions of two networks based on two dimensions of distance and shape. First, we propose an adversarial network distribution matching model to perform the bidirectional cross-network alignment translations between two networks, such that the cross-network transformed distributions of two networks move closer to each other and finally meet with each other halfwayc In addition, a homophily consistency loss is introduced to maintain the vertex homophily consistency between pairwise vertices on two networks in both the embedding space. Second, in order to address the feature inconsistency issue, we integrate a dual adversarial autoencoder module with an adversarial two-class classification model together to twist the cross-network transformed distributions of two networks, such that two distributions could have the same shape. This facilitates the translations of the distributions of two networks in the adversarial network distribution matching model. Moreover, a semantic preservation loss is introduced to preserve the original embedding semantics of one network when this network is translated to another network and returned to itself. Third but last, the competition game by integrating the above two adversarial models together can help project two copies of the same vertices with high-dimensional inconsistent features into the same low-dimensional embedding space, and thus guarantee the distribution consistency between two networks in terms of both distance and shape.
更多查看译文
关键词
Network alignment, high dimensional inconsistent features, adversarial matching, adversarial classification
AI 理解论文
溯源树
样例
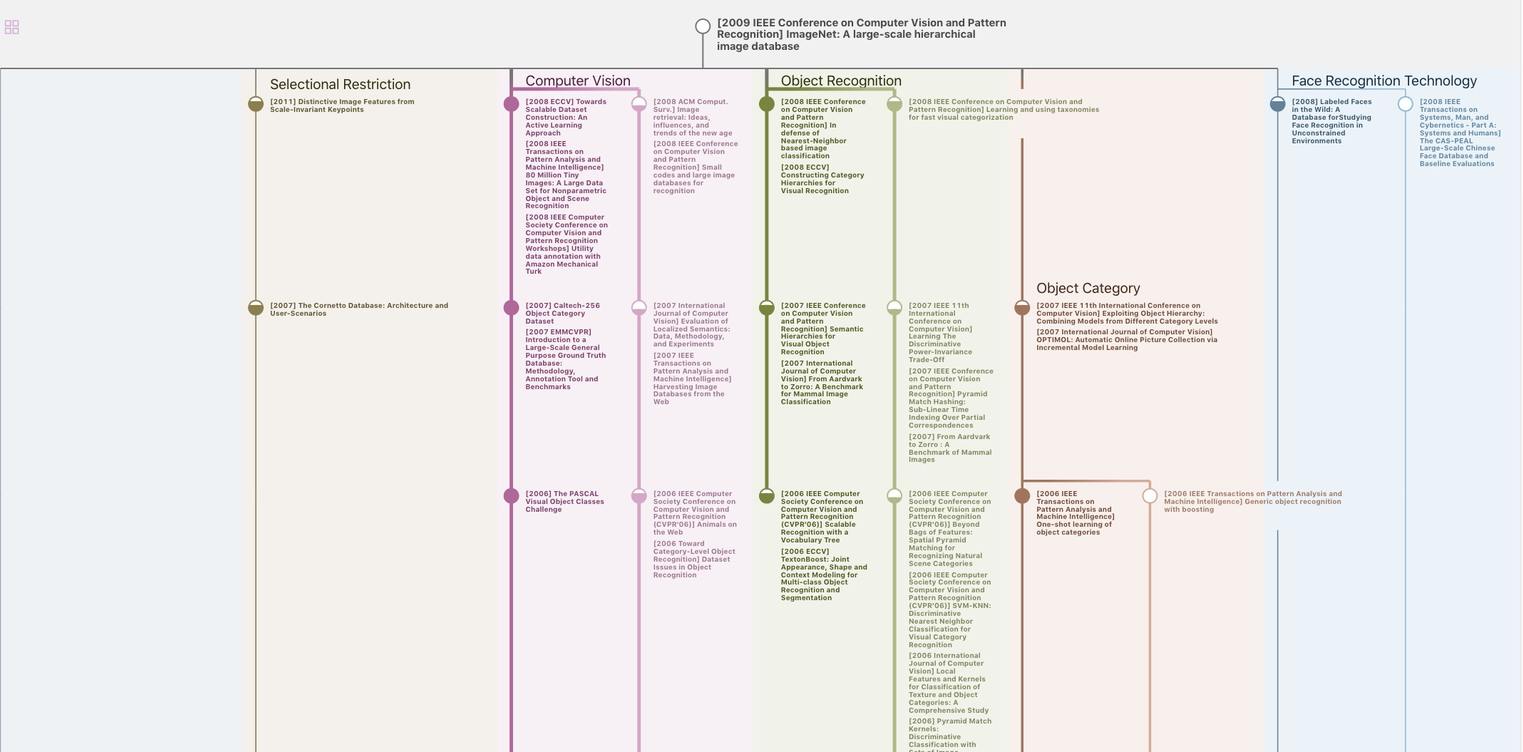
生成溯源树,研究论文发展脉络
Chat Paper
正在生成论文摘要