Contrast Feature Dependency Pattern Mining For Controlled Experiments With Application To Driving Behavior
2019 19TH IEEE INTERNATIONAL CONFERENCE ON DATA MINING (ICDM 2019)(2019)
摘要
A controlled experiment is an empirical interventional study method to evaluate the causal impact of an intervention, by identifying the dynamic feature dependency patterns in the contrast multivariate time series (CMTS) collected from the control and experimental groups. Manually labeling or interpreting the effects caused by the intervention from the CMTS data has become an infeasible task even for domain experts. Thus, it is imperative to develop an integrated technique, preferably in an unsupervised manner, that can simultaneously identify and characterize feature dynamic dependencies and their contrast patterns in CMTS, which we call the contrast dynamic feature dependency (CDFD) patterns. In this paper, we propose a generative model with partial correlation-based feature dependency regularization to help analysts understand the CMTS data by jointly 1) characterizing a set of comparable multivariate Gaussian distributions from CMTS, and 2) determining whether the intervention causes the changes between two comparable distributions. Extensive experiments demonstrate the effectiveness and scalability of the proposed method. The proposed method applied to a driving behavior application demonstrates its utility and interpretability.
更多查看译文
关键词
Contrast Pattern,Time Series,Markov Random Fields,Feature Dependency,Driving Behavior,Controlled Experiment
AI 理解论文
溯源树
样例
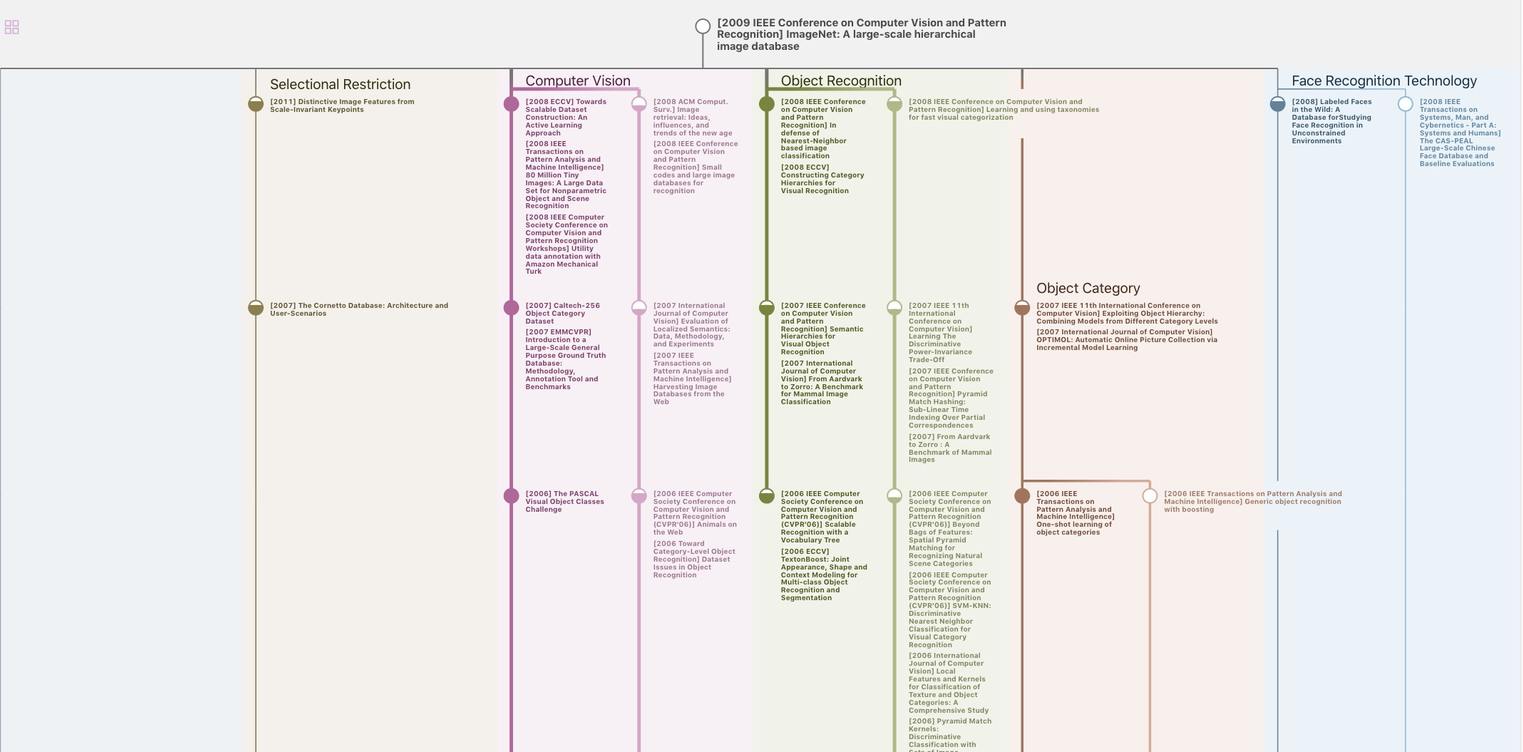
生成溯源树,研究论文发展脉络
Chat Paper
正在生成论文摘要