Block-Structured Optimization For Anomalous Pattern Detection In Interdependent Networks
2019 19TH IEEE INTERNATIONAL CONFERENCE ON DATA MINING (ICDM 2019)(2019)
摘要
We propose a generalized optimization framework for detecting anomalous patterns (subgraphs that are interesting or unexpected) in interdependent networks, such as multi-layer networks, temporal networks, networks of networks, and many others. We frame the problem as a non-convex optimization that has a general nonlinear score function and a set of block-structured and non-convex constraints. We develop an effective, efficient, and parallelizable projection-based algorithm, namely Graph Block-structured Gradient Projection (GBGP), to solve the problem. It is proved that our algorithm 1) runs in nearly-linear time on the network size, and 2) enjoys a theoretical approximation guarantee. Moreover, we demonstrate how our framework can be applied to two very practical applications, and we conduct comprehensive experiments to show the effectiveness and efficiency of our proposed algorithm.
更多查看译文
关键词
subgraph detection, sparse optimization, interdependent networks
AI 理解论文
溯源树
样例
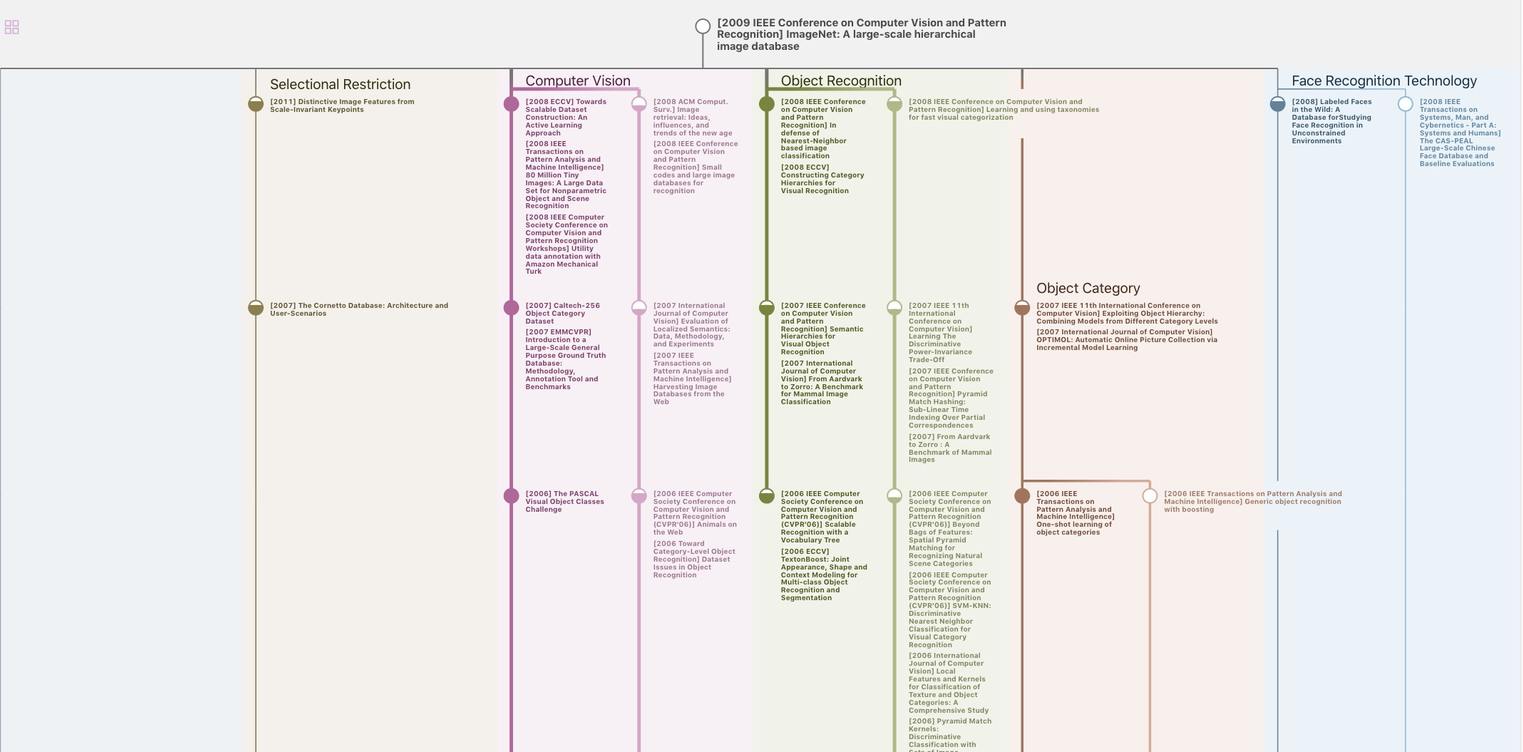
生成溯源树,研究论文发展脉络
Chat Paper
正在生成论文摘要