Bi-directional Causal Graph Learning through Weight-Sharing and Low-Rank Neural Network
2019 IEEE International Conference on Data Mining (ICDM)(2019)
摘要
Discovering the causal graph in multivariate time series data is of great importance for industrial society, yet challenging due to the unknown nonlinearity in the data. Existing works only explore the data in chronological order, and rely on pre-assumed kernels or certain distribution assumption. In this paper, we present a Bi-directional neural network for Causal Graph Learning (Bi-CGL) through weight-sharing and low-rank neural network. It discovers the causal graph by simultaneously exploring input in forward and reverse chronological order. Both directions approach the same causal graph with shared low-rank approximation, which provides robustness and better accuracy against data noise. Experiments on synthetic and real world datasets prove our Bi-CGL's outperformance over existing baselines.
更多查看译文
关键词
causal graph learning,bi-directional neural network,weight-sharing
AI 理解论文
溯源树
样例
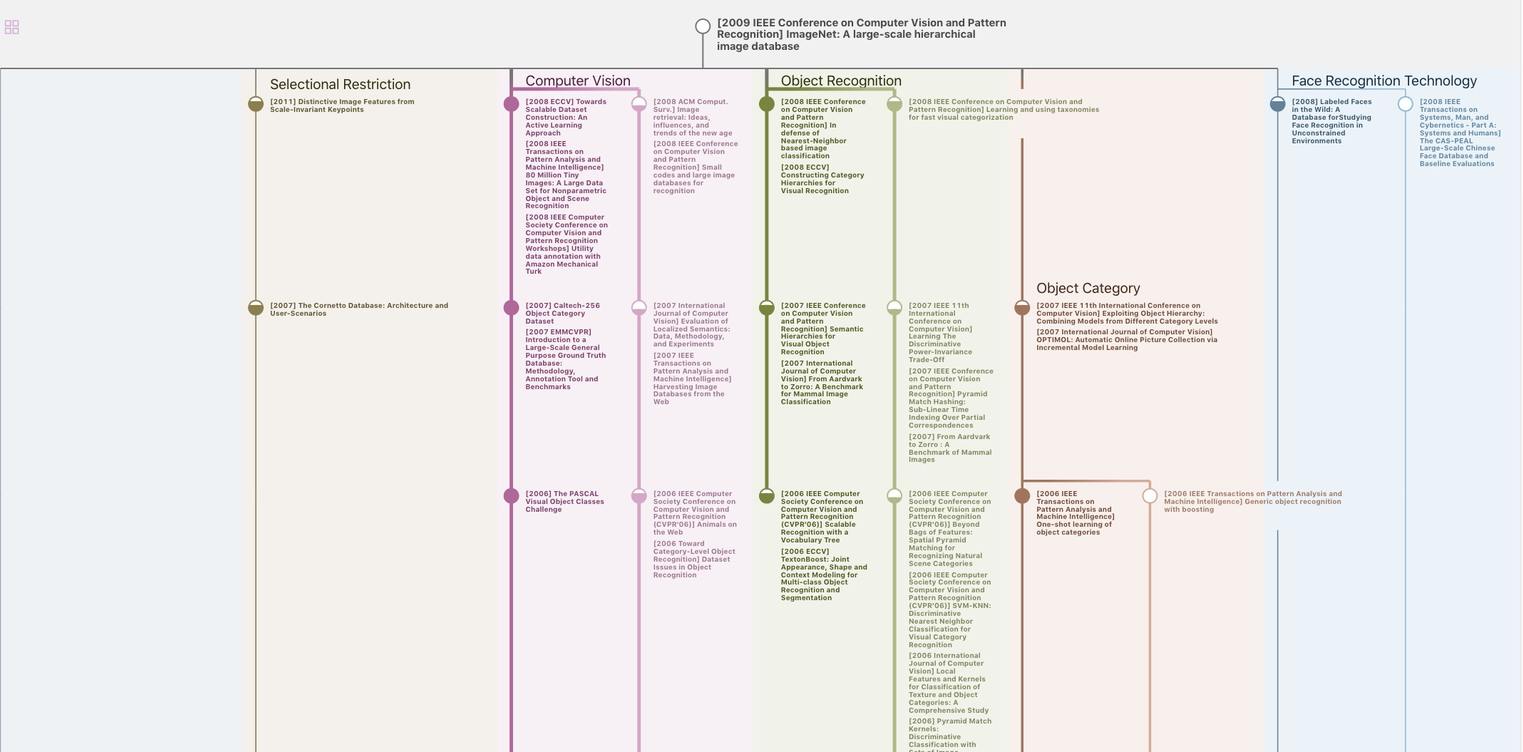
生成溯源树,研究论文发展脉络
Chat Paper
正在生成论文摘要