Online Budgeted Least Squares with Unlabeled Data
2019 IEEE International Conference on Data Mining (ICDM)(2019)
摘要
The scarcity of labeled data in real streaming environments has boosted the study of online semi-supervised learning (SSL). However, existing online SSL models often rely on some specific assumptions (e.g., manifold assumption) and need to maintain some extra constraints (e.g., the Laplacian matrix) on the fly, which is usually time and resource consuming. In this paper, we propose an efficient and effective online semi-supervised learning approach via Budgeted Least Square (BLS). Specifically, we first derive both closed-form transductive and inductive solutions for kernel least squares classification in the semi-supervised setting. Then, together with online kernel learning, BLS allows a concise online update. Besides, the theoretical regret bound of BLS is analysed, and empirical experiments on both static and streaming data further demonstrate its superiority over state-of-the-art algorithms.
更多查看译文
关键词
semi-supervised learning,online Learning,classification
AI 理解论文
溯源树
样例
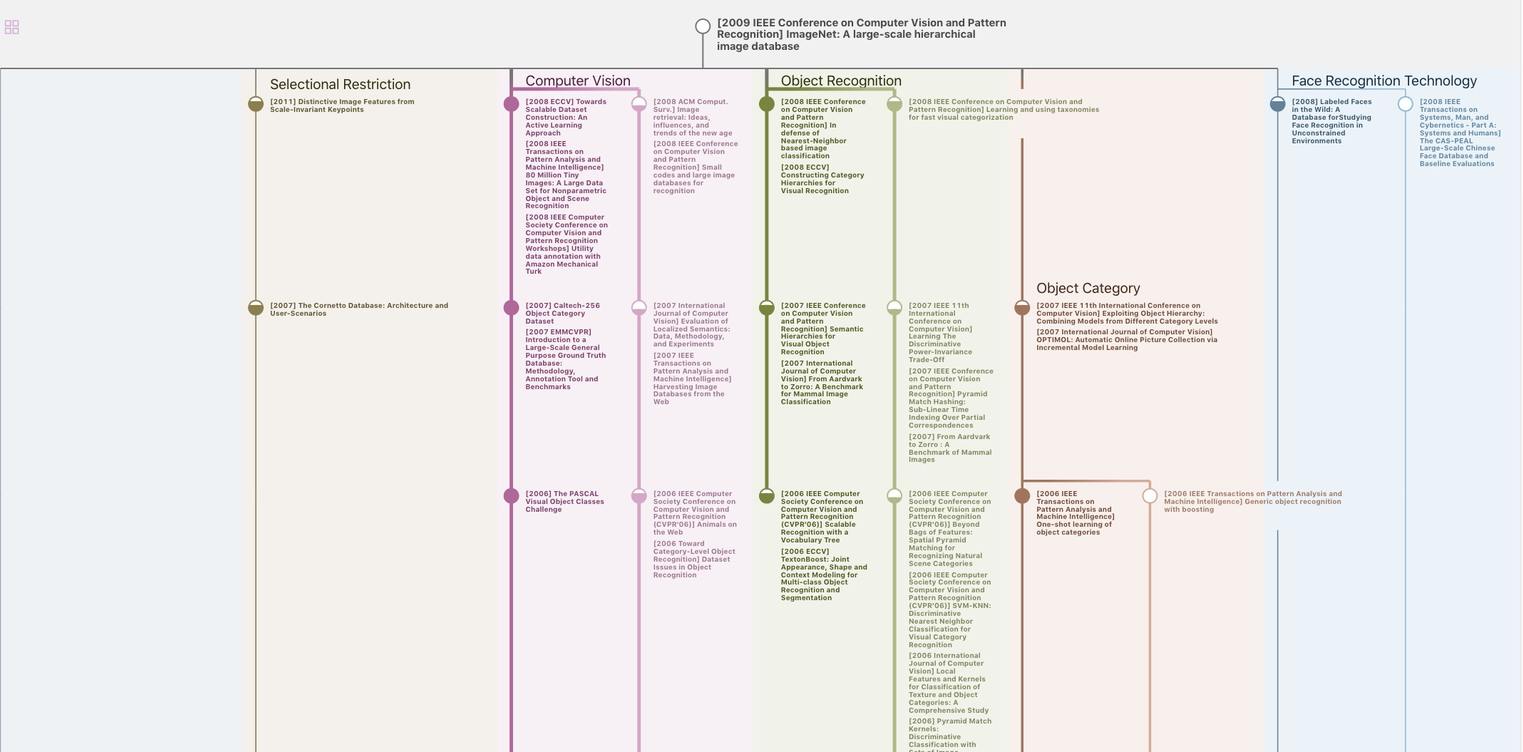
生成溯源树,研究论文发展脉络
Chat Paper
正在生成论文摘要