Optimal Timelines For Network Processes
2019 19TH IEEE INTERNATIONAL CONFERENCE ON DATA MINING (ICDM 2019)(2019)
摘要
Structural models for network dynamics typically assume a discrete timeline of network events (node activation or link creation) and a stochastic generative process giving rise to new events based on the event history and the network structure. In order to employ these models for prediction, observational data is often aggregated at a fixed temporal resolution (e.g., minutes or days). However, the underlying network processes may "speed up" or "slow down" at different points in time, rendering observations unlikely and predictions incorrect. The challenge is to optimize the timescale for the analysis of network event data, which in turn is based on structural models of the underlying network processes.We introduce the general problem of inferring the optimal temporal resolution for network event data. The goal is to map observed network events to discrete time steps by aggregation and/or disaggregation of their original timeline such that they are collectively well-explained by structural dynamics models. We unify network growth and information diffusion models and differentiate between short- and long-memory processes. We demonstrate that while optimal temporal aggregation can be performed in polynomial time, disaggregation-and thus, the general timescale inference problem-is NP-hard. We propose scalable heuristics for the problem, some with approximation guarantees, and employ them for missing event recovery and temporal link prediction, demonstrating significant improvements (absolute increase of 10% in F-1 measure for event recovery and of 5% in AUC for link prediction) compared to employing the same algorithms on the default timescale of data collection.
更多查看译文
关键词
Temporal networks, Network clock, Timeline reconstruction, Structural network models
AI 理解论文
溯源树
样例
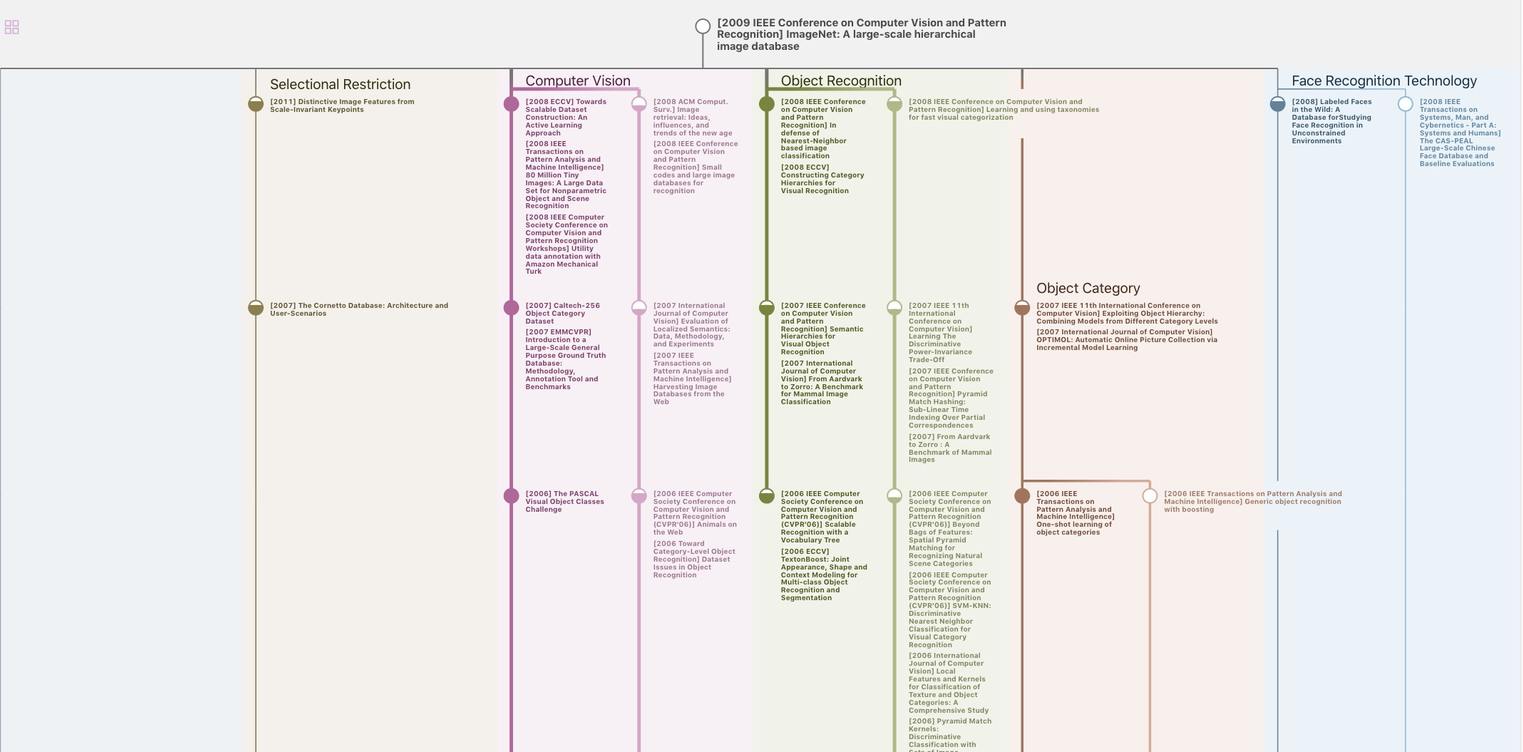
生成溯源树,研究论文发展脉络
Chat Paper
正在生成论文摘要