A Unified Framework For Generalizable Style Transfer: Style And Content Separation
IEEE TRANSACTIONS ON IMAGE PROCESSING(2020)
摘要
Image style transfer has drawn broad attention recently. However, most existing methods aim to explicitly model the transformation between different styles, and the learned model is often not generalizable to new styles. Based on the idea of style and content separation, we here propose a unified style transfer framework that consists of style encoder, content encoder, mixer and decoder. The style encoder and the content encoder are used to extract the style and content representations from the corresponding reference images. The two representations are integrated by the mixer and fed to the decoder, which generates images with the target style and content. Assuming the same encoder could be shared among different styles/contents, the style/content encoder explores a generalizable way to represent style/content information, i.e. the encoders are expected to capture the underlying representation for different styles/contents and generalize to new styles/contents. Training simultaneously with a number of styles and contents, the framework enables building one single transfer network for multiple styles and further leads to a key merit of the framework, i.e. its generalizability to new styles and contents. To evaluate the proposed framework, we apply it to both supervised and unsupervised style transfer, using character typeface transfer and neural style transfer as respective examples. For character typeface transfer, to separate the style features and content features, we leverage the conditional dependence of styles and contents given an image. For neural style transfer, we leverage the statistical information of feature maps in certain layers to represent style. Extensive experimental results have demonstrated the effectiveness and robustness of the proposed methods. Furthermore, models learned under the proposed framework are shown to be better generalizable to new styles and contents.
更多查看译文
关键词
Style and content separation, character typeface, transfer neural style transfer
AI 理解论文
溯源树
样例
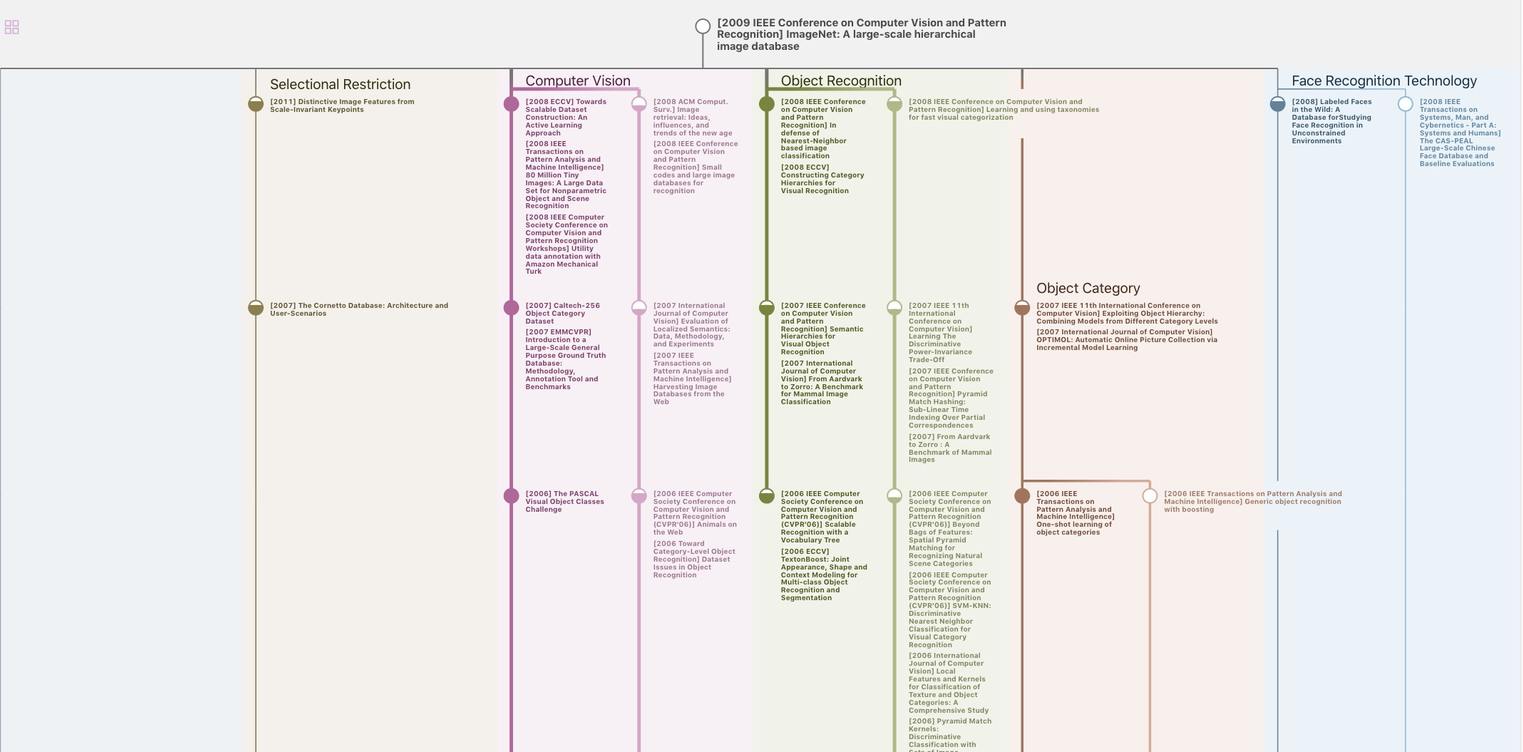
生成溯源树,研究论文发展脉络
Chat Paper
正在生成论文摘要