Action Graphs: Weakly-supervised Action Localization with Graph Convolution Networks
2020 IEEE Winter Conference on Applications of Computer Vision (WACV)(2020)
摘要
We present a method for weakly-supervised action localization based on graph convolutions. In order to find and classify video time segments that correspond to relevant action classes, a system must be able to both identify discriminative time segments in each video, and identify the full extent of each action. Achieving this with weak video level labels requires the system to use similarity and dissimilarity between moments across videos in the training data to understand both how an action appears, as well as the subactions that comprise the action's full extent. However, current methods do not make explicit use of similarity between video moments to inform the localization and classification predictions. We present a novel method that uses graph convolutions to explicitly model similarity between video moments. Our method utilizes similarity graphs that encode appearance and motion, and pushes the state of the art on THUMOS'14, ActivityNet 1.2, and Charades for weakly- supervised action localization.
更多查看译文
关键词
video moments,graph convolutions,similarity graphs,action graphs,weakly-supervised action localization,graph convolution networks,weak video level labels,discriminative time segment identification,localization prediction,video time segment classification,classification prediction,ActivityNet 1.2,Charades,THUMOS'14
AI 理解论文
溯源树
样例
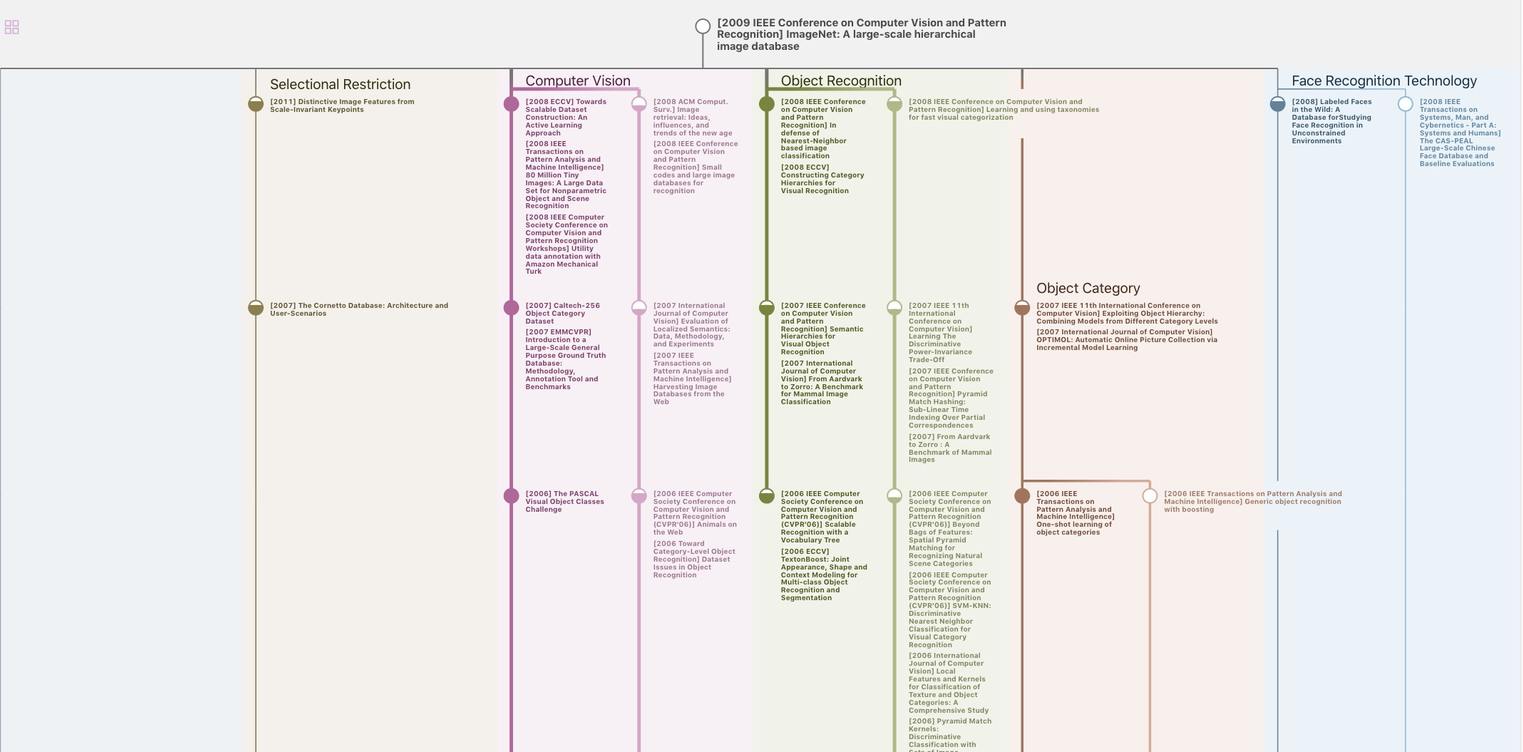
生成溯源树,研究论文发展脉络
Chat Paper
正在生成论文摘要