Multistage Model for Robust Face Alignment Using Deep Neural Networks
Cognitive Computation(2021)
摘要
The ability to generalize unconstrained conditions such as severe occlusions and large pose variations remains a challenging goal to achieve in face alignment. In this paper, a multistage model based on deep neural networks is proposed which takes advantage of spatial transformer networks, hourglass networks and exemplar-based shape constraints. First, a spatial transformer-generative adversarial network which consists of convolutional layers and residual units is utilized to solve the initialization issues caused by face detectors, such as rotation and scale variations, to obtain improved face bounding boxes for face alignment. Then, stacked hourglass network is employed to obtain preliminary locations of landmarks as well as their corresponding scores. In addition, an exemplar-based shape dictionary is designed to determine landmarks with low scores based on those with high scores. By incorporating face shape constraints, misaligned landmarks caused by occlusions or cluttered backgrounds can be considerably improved. Extensive experiments based on challenging benchmark datasets are performed to demonstrate the superior performance of the proposed method over other state-of-the-art methods.
更多查看译文
关键词
Face alignment,Facial landmark detection,Multistage model,Spatial transformer generative adversarial networks,Stacked hourglass networks,Exemplar-based shape constraints
AI 理解论文
溯源树
样例
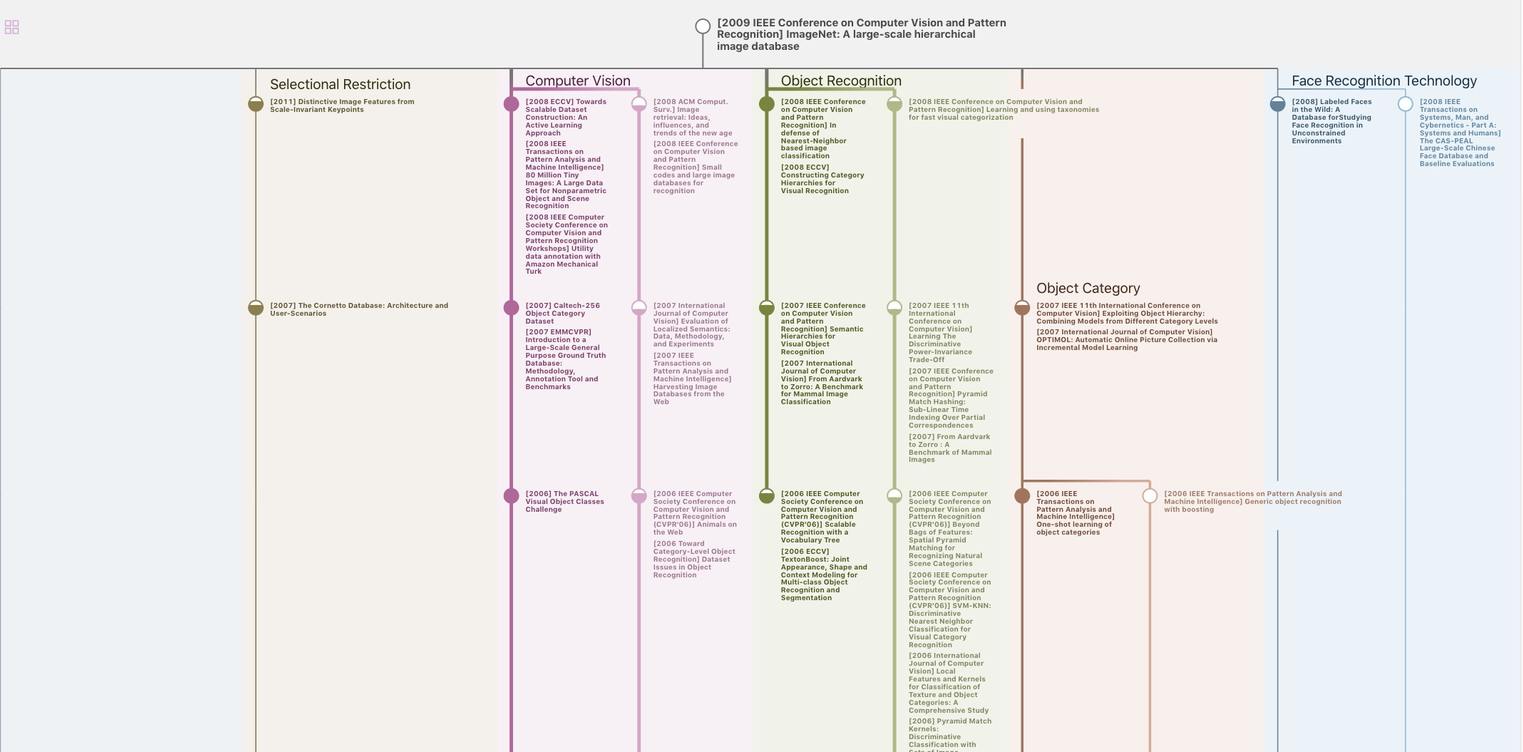
生成溯源树,研究论文发展脉络
Chat Paper
正在生成论文摘要