Differentiation of non-small cell lung cancer and histoplasmosis pulmonary nodules: insights from radiomics model performance compared with clinician observers.
TRANSLATIONAL LUNG CANCER RESEARCH(2019)
摘要
Background: Histoplasmosis pulmonary nodules often present in computed tomography (CT) imaging with characteristics suspicious for lung cancer. This presents a work-up decision issue for clinicians in regions where histoplasmosis is an endemic fungal infection, when a nodule suspicious for lung cancer is detected. We hypothesize the application of radiomic features extracted from pulmonary nodules and perinodular parenchyma could accurately distinguish between suspicious histoplasmosis lung nodules and non-small cell lung cancer (NSCLC). Methods: A retrospective clinical cohort of pulmonary nodules with a confirmed diagnosis of histoplasmosis or NSCLC was collected from the University of Iowa Hospitals and Clines. Radiomic features were extracted describing characteristics of the nodule and perinodular parenchyma regions and used to build a machine learning tool. These cases were assessed by four expert clinicians who gave a blinded risk prediction for NSCLC. Tool and observer performance were assessed by calculating the area under the curve for the receiver operating characteristic (AUC-ROC) and interdass correlation coefficient (ICC). Results: A cohort of 71 subjects with confirmed histopathology (40 NSCLC, 31 histoplasmosis) were case-matched based on age, sex, and smoking history. Superior performance (AUC-ROC =0.89) was demonstrated using leave-one-subject out validation in the tool that incorporated radiomics from the nodule and perinodular parenchyma region extended to 100% nodule diameter. Observers had perfect intrarepeatability (ICC =1.0) and demonstrated fair inter-reader variability (ICC =0.52). Conclusions: Radiomics have potential utility in the challenging task of differentiation between lung cancer and histoplasmosis. Expert clinician readers have high intra-repeatability but demonstrated interreader variability which could provide context for a supplemental radiomics-based tool.
更多查看译文
关键词
Granuloma,machine learning,perinodular,risk assessment
AI 理解论文
溯源树
样例
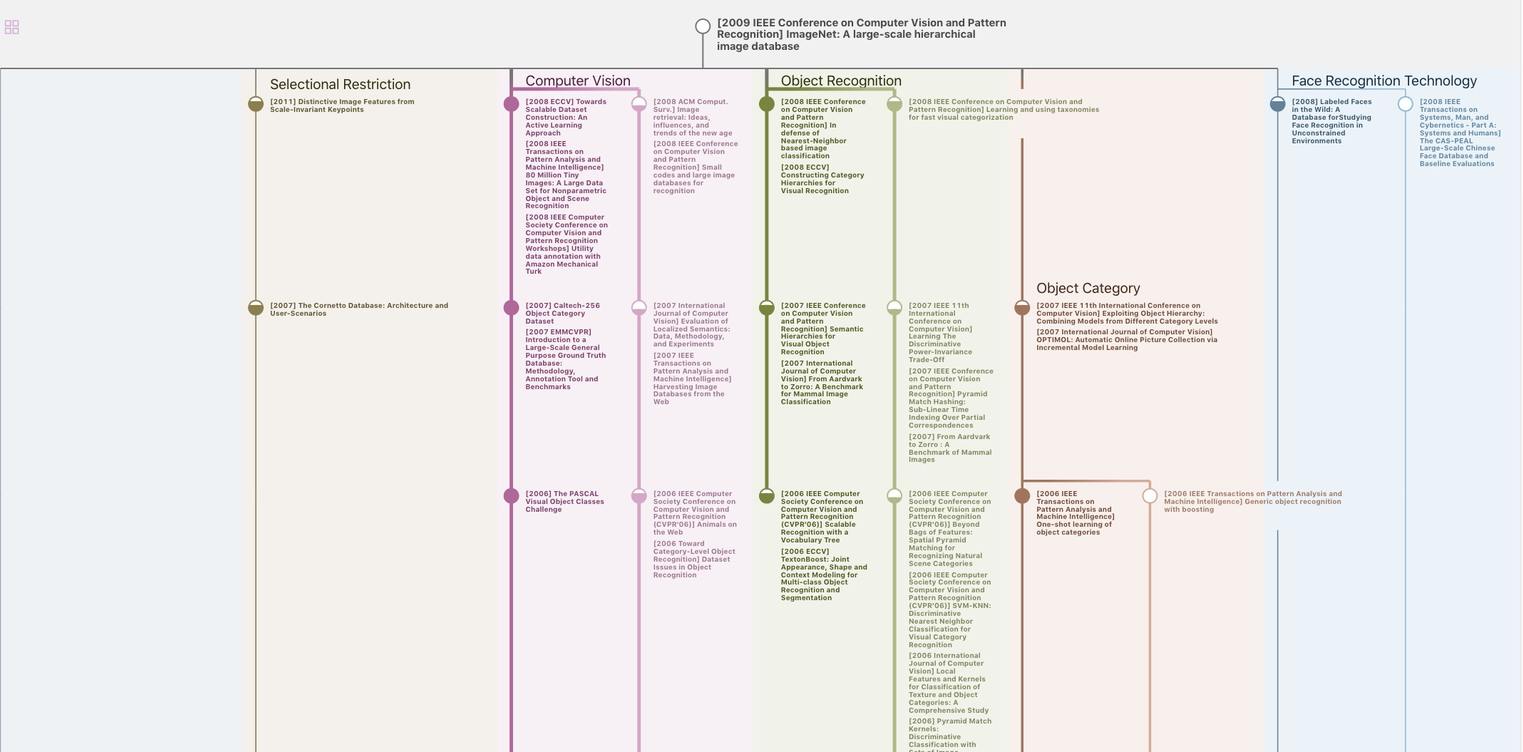
生成溯源树,研究论文发展脉络
Chat Paper
正在生成论文摘要