When Less Is More: Systematic Analysis of Cascade-Based Community Detection
ACM Transactions on Knowledge Discovery from Data(2022)
摘要
AbstractInformation diffusion, spreading of infectious diseases, and spreading of rumors are fundamental processes occurring in real-life networks. In many practical cases, one can observe when nodes become infected, but the underlying network, over which a contagion or information propagates, is hidden. Inferring properties of the underlying network is important since these properties can be used for constraining infections, forecasting, viral marketing, and so on. Moreover, for many applications, it is sufficient to recover only coarse high-level properties of this network rather than all its edges. This article conducts a systematic and extensive analysis of the following problem: Given only the infection times, find communities of highly interconnected nodes. This task significantly differs from the well-studied community detection problem since we do not observe a graph to be clustered. We carry out a thorough comparison between existing and new approaches on several large datasets and cover methodological challenges specific to this problem. One of the main conclusions is that the most stable performance and the most significant improvement on the current state-of-the-art are achieved by our proposed simple heuristic approaches agnostic to a particular graph structure and epidemic model. We also show that some well-known community detection algorithms can be enhanced by including edge weights based on the cascade data.
更多查看译文
关键词
Community detection, information propagation, epidemic cascades, diffusion, network inference, likelihood optimization
AI 理解论文
溯源树
样例
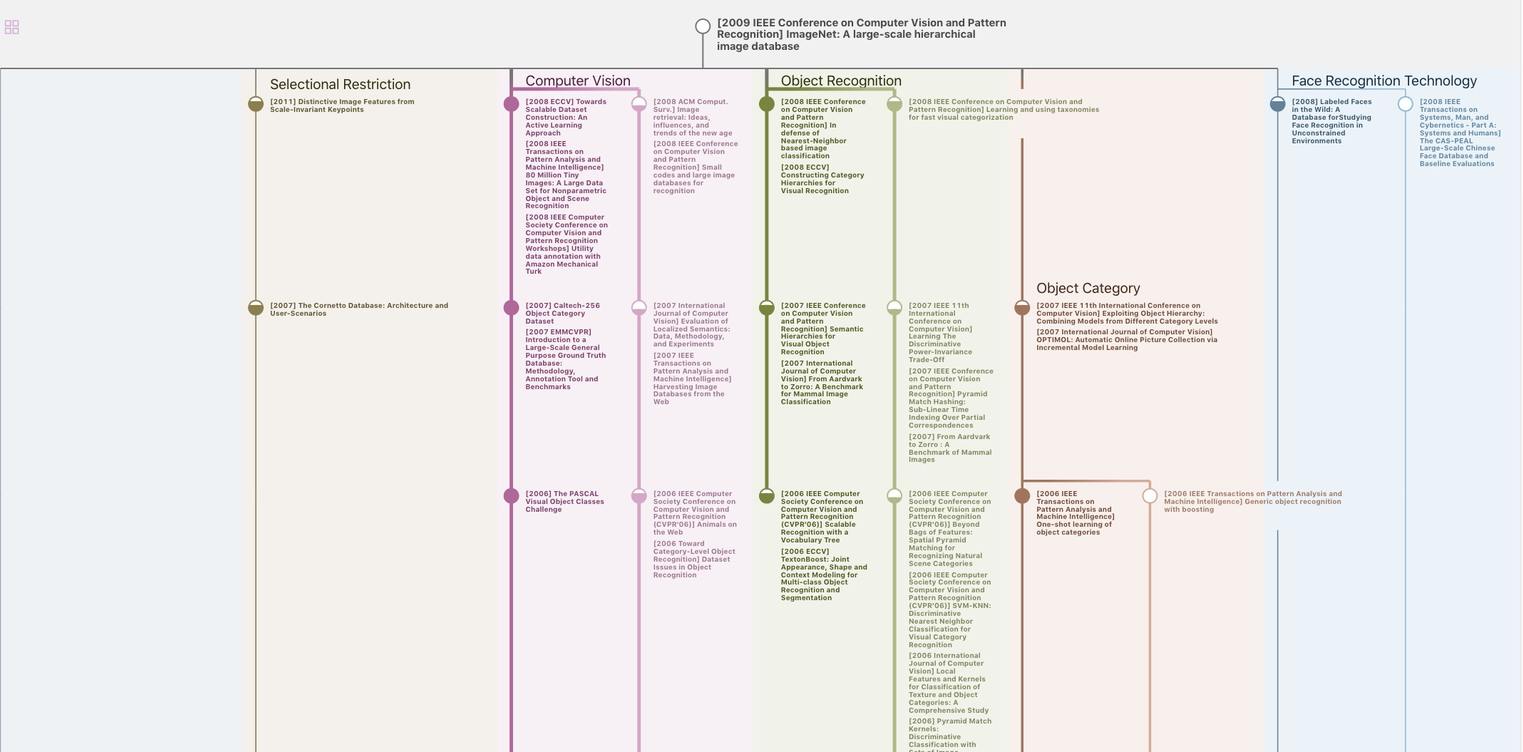
生成溯源树,研究论文发展脉络
Chat Paper
正在生成论文摘要