Modeling ASR Ambiguity for Dialogue State Tracking Using Word Confusion Networks
arxiv(2020)
摘要
Spoken dialogue systems typically use a list of top-N ASR hypotheses for inferring the semantic meaning and tracking the state of the dialogue. However ASR graphs, such as confusion networks (confnets), provide a compact representation of a richer hypothesis space than a top-N ASR list. In this paper, we study the benefits of using confusion networks with a state-of-the-art neural dialogue state tracker (DST). We encode the 2-dimensional confnet into a 1-dimensional sequence of embeddings using an attentional confusion network encoder which can be used with any DST system. Our confnet encoder is plugged into the state-of-the-art 'Global-locally Self-Attentive Dialogue State Tacker' (GLAD) model for DST and obtains significant improvements in both accuracy and inference time compared to using top-N ASR hypotheses.
更多查看译文
关键词
dialogue state tracking,word confusion networks,asr ambiguity
AI 理解论文
溯源树
样例
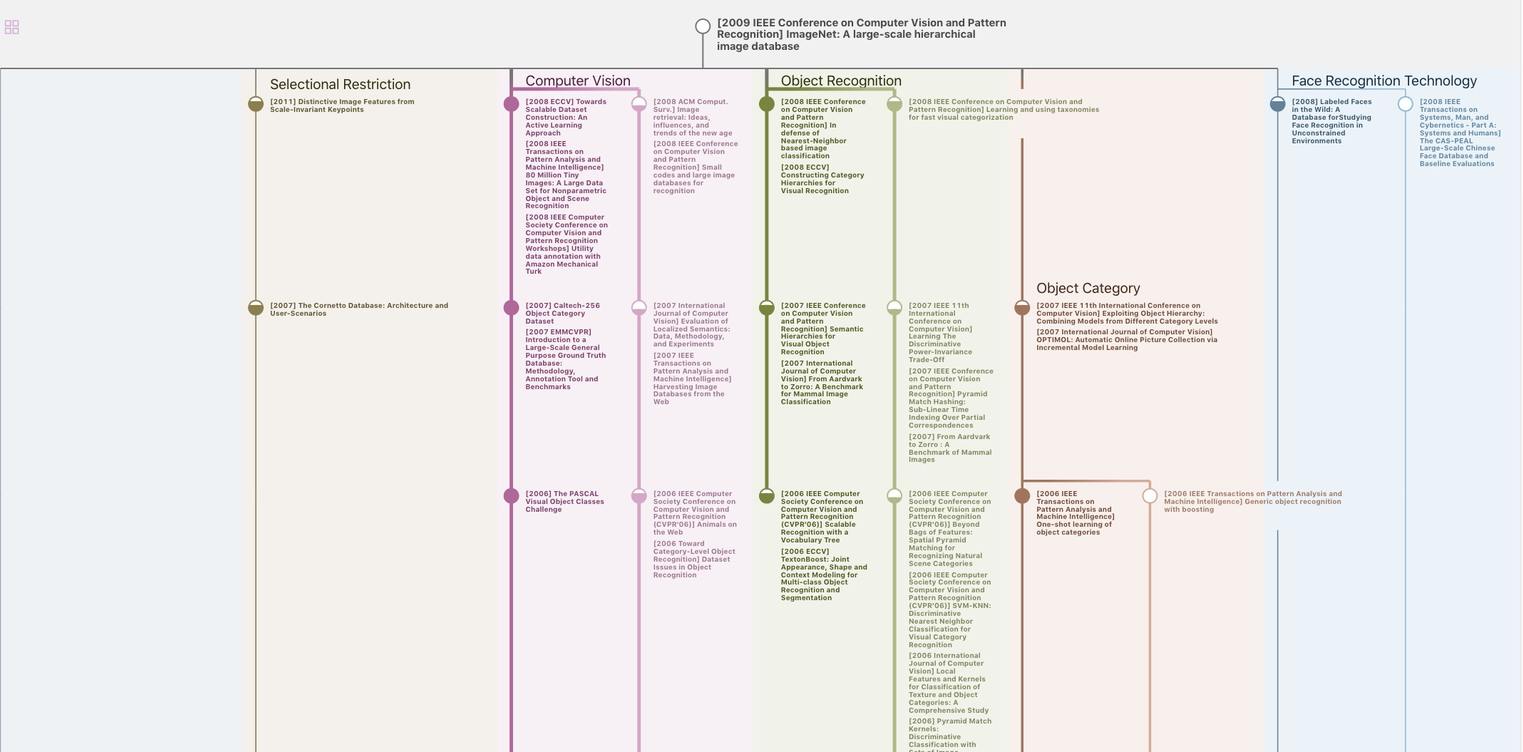
生成溯源树,研究论文发展脉络
Chat Paper
正在生成论文摘要