Decoding Movement Imagination and Execution from EEG Signals using BCI-Transfer Learning Method based on Relation Network
2020 IEEE INTERNATIONAL CONFERENCE ON ACOUSTICS, SPEECH, AND SIGNAL PROCESSING(2020)
摘要
A brain-computer interface (BCI) is used not only to control external devices for healthy people but also to rehabilitate motor functions for motor-disabled patients. Decoding movement intention is one of the most significant aspects for performing arm movement tasks using brain signals. Decoding movement execution (ME) from electroencephalogram (EEG) signals have shown high performance in previous works, however movement imagination (MI) paradigm-based intention decoding has so far failed to achieve sufficient accuracy. In this study, we focused on a robust MI decoding method with transfer learning for the ME and MI paradigm. We acquired EEG data related to arm reaching for 3D directions. We proposed a BCI-transfer learning method based on a Relation network (BTRN) architecture. Decoding performances showed the highest performance compared to conventional works. We confirmed the possibility of the BTRN architecture to contribute to continuous decoding of MI using ME datasets.
更多查看译文
关键词
Brain-computer interface (BCI), Electroencephalogram (EEG), Transfer learning, Movement imagination and execution
AI 理解论文
溯源树
样例
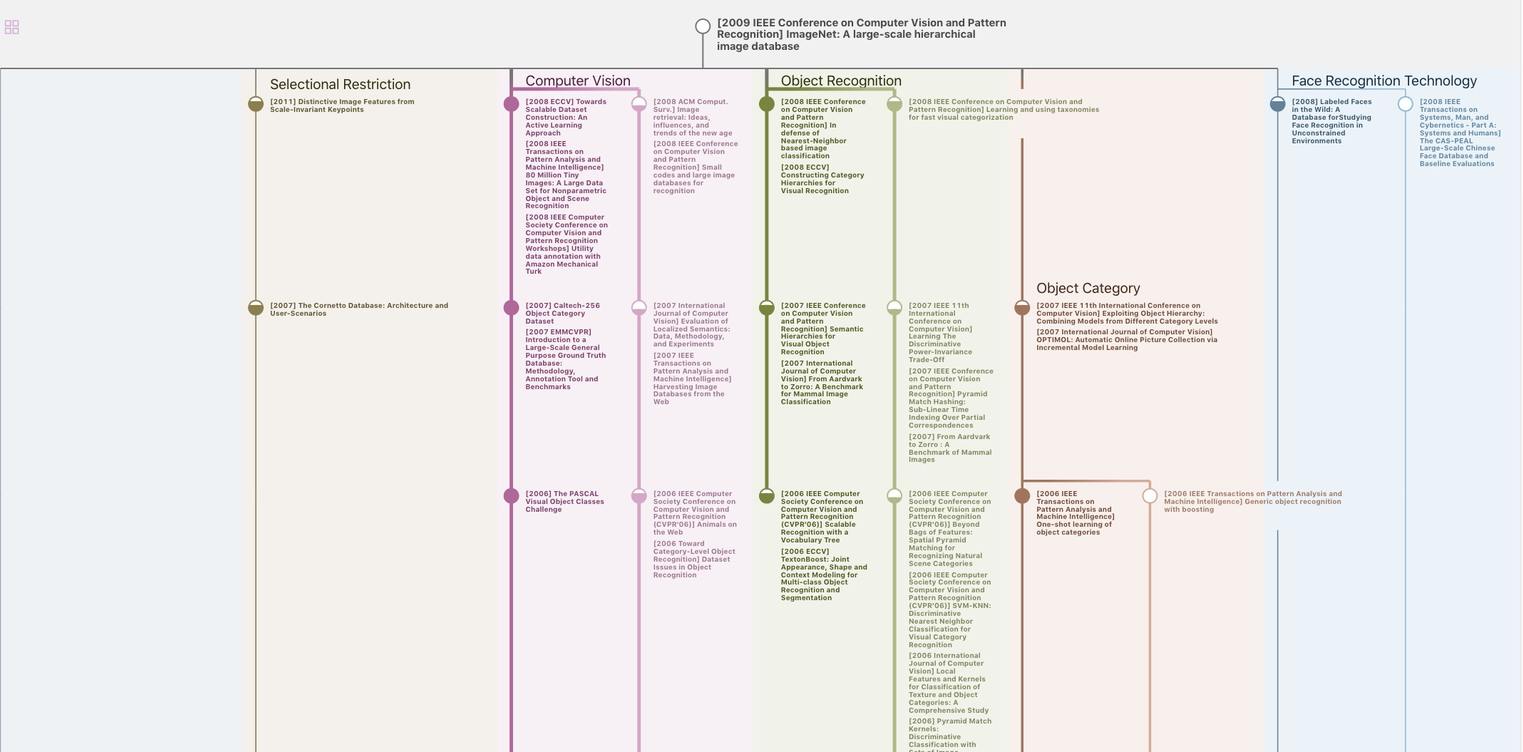
生成溯源树,研究论文发展脉络
Chat Paper
正在生成论文摘要