Provable Noisy Sparse Subspace Clustering using Greedy Neighbor Selection: A Coherence-Based Perspective
arxiv(2020)
摘要
Sparse subspace clustering (SSC) using greedy-based neighbor selection, such as matching pursuit (MP) and orthogonal matching pursuit (OMP), has been known as a popular computationally-efficient alternative to the conventional L1-minimization based methods. Under deterministic bounded noise corruption, in this paper we derive coherence-based sufficient conditions guaranteeing correct neighbor identification using MP/OMP. Our analyses exploit the maximum/minimum inner product between two noisy data points subject to a known upper bound on the noise level. The obtained sufficient condition clearly reveals the impact of noise on greedy-based neighbor recovery. Specifically, it asserts that, as long as noise is sufficiently small so that the resultant perturbed residual vectors stay close to the desired subspace, both MP and OMP succeed in returning a correct neighbor subset. A striking finding is that, when the ground truth subspaces are well-separated from each other and noise is not large, MP-based iterations, while enjoying lower algorithmic complexity, yield smaller perturbation of residuals, thereby better able to identify correct neighbors and, in turn, achieving higher global data clustering accuracy. Extensive numerical experiments are used to corroborate our theoretical study.
更多查看译文
关键词
greedy neighbor selection,clustering,sparse,coherence-based
AI 理解论文
溯源树
样例
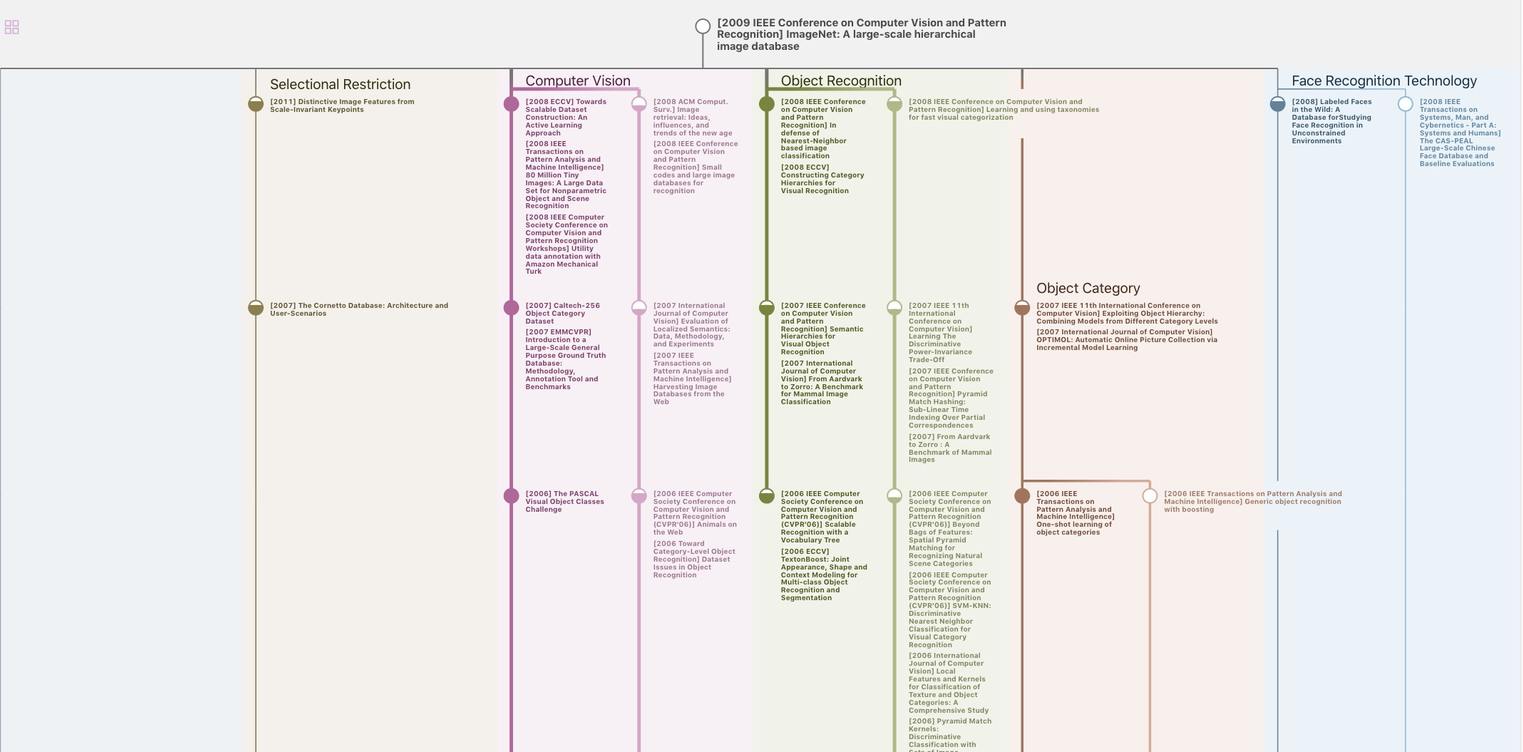
生成溯源树,研究论文发展脉络
Chat Paper
正在生成论文摘要