A Survey on Knowledge Graphs: Representation, Acquisition, and Applications.
IEEE Transactions on Neural Networks and Learning Systems(2022)
摘要
Human knowledge provides a formal understanding of the world. Knowledge graphs that represent structural relations between entities have become an increasingly popular research direction toward cognition and human-level intelligence. In this survey, we provide a comprehensive review of the knowledge graph covering overall research topics about: 1) knowledge graph representation learning; 2) knowledge acquisition and completion; 3) temporal knowledge graph; and 4) knowledge-aware applications and summarize recent breakthroughs and perspective directions to facilitate future research. We propose a full-view categorization and new taxonomies on these topics. Knowledge graph embedding is organized from four aspects of representation space, scoring function, encoding models, and auxiliary information. For knowledge acquisition, especially knowledge graph completion, embedding methods, path inference, and logical rule reasoning are reviewed. We further explore several emerging topics, including metarelational learning, commonsense reasoning, and temporal knowledge graphs. To facilitate future research on knowledge graphs, we also provide a curated collection of data sets and open-source libraries on different tasks. In the end, we have a thorough outlook on several promising research directions.
更多查看译文
关键词
Deep learning,knowledge graph completion (KGC),knowledge graph,reasoning,relation extraction,representation learning
AI 理解论文
溯源树
样例
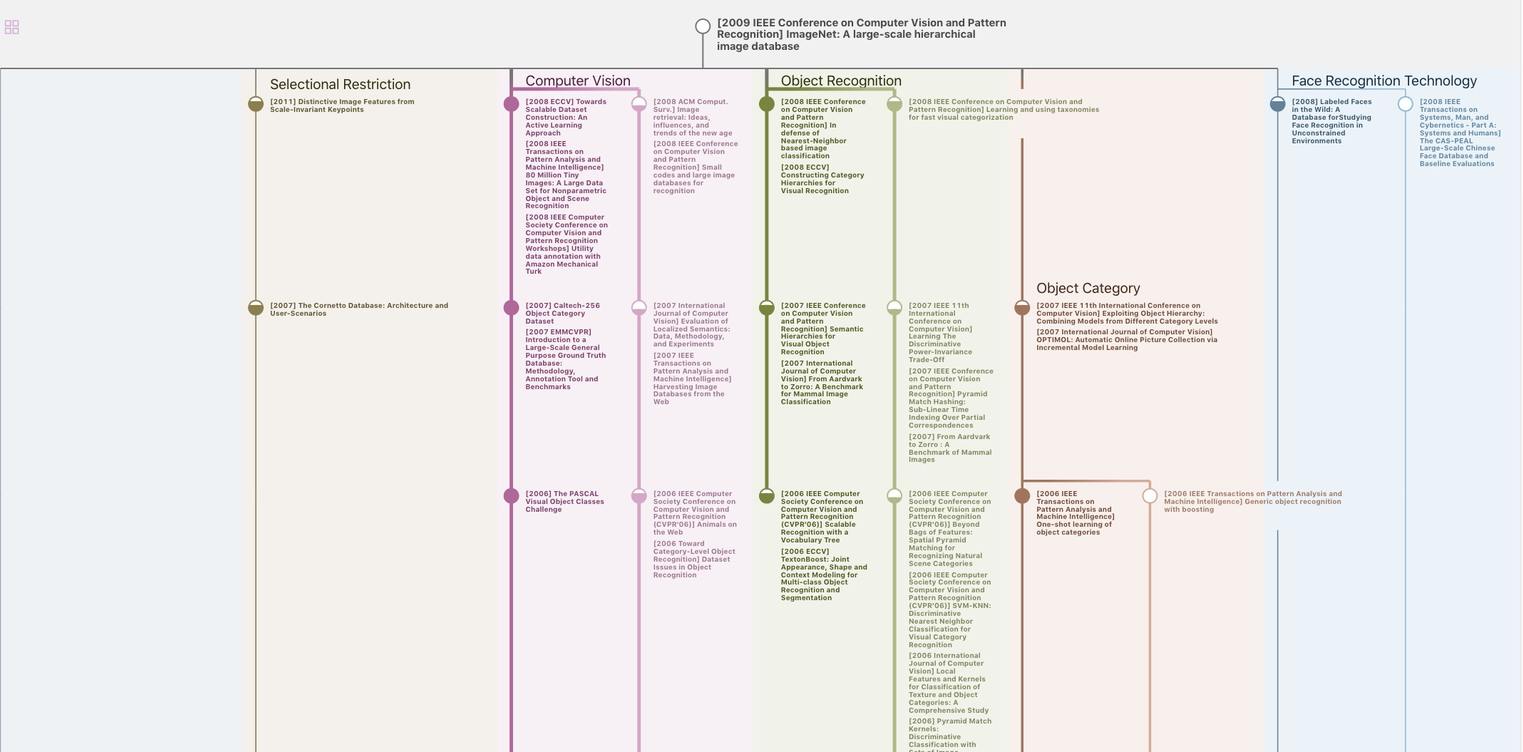
生成溯源树,研究论文发展脉络
Chat Paper
正在生成论文摘要