Semantic Discord: Finding Unusual Local Patterns for Time Series
PROCEEDINGS OF THE 2020 SIAM INTERNATIONAL CONFERENCE ON DATA MINING (SDM)(2020)
摘要
Finding anomalous subsequence in a long time series is a very important but difficult problem. Existing state-of-the-art methods have been focusing on searching for the subsequence that is the most dissimilar to the rest of the subsequences; however, they do not take into account the background patterns that contain the anomalous candidates. As a result, such approaches are likely to miss local anomalies. We introduce a new definition named \textit{semantic discord}, which incorporates the context information from larger subsequences containing the anomaly candidates. We propose an efficient algorithm with a derived lower bound that is up to 3 orders of magnitude faster than the brute force algorithm in real world data. We demonstrate that our method significantly outperforms the state-of-the-art methods in locating anomalies by extensive experiments. We further explain the interpretability of semantic discord.
更多查看译文
关键词
unusual local patterns,time series
AI 理解论文
溯源树
样例
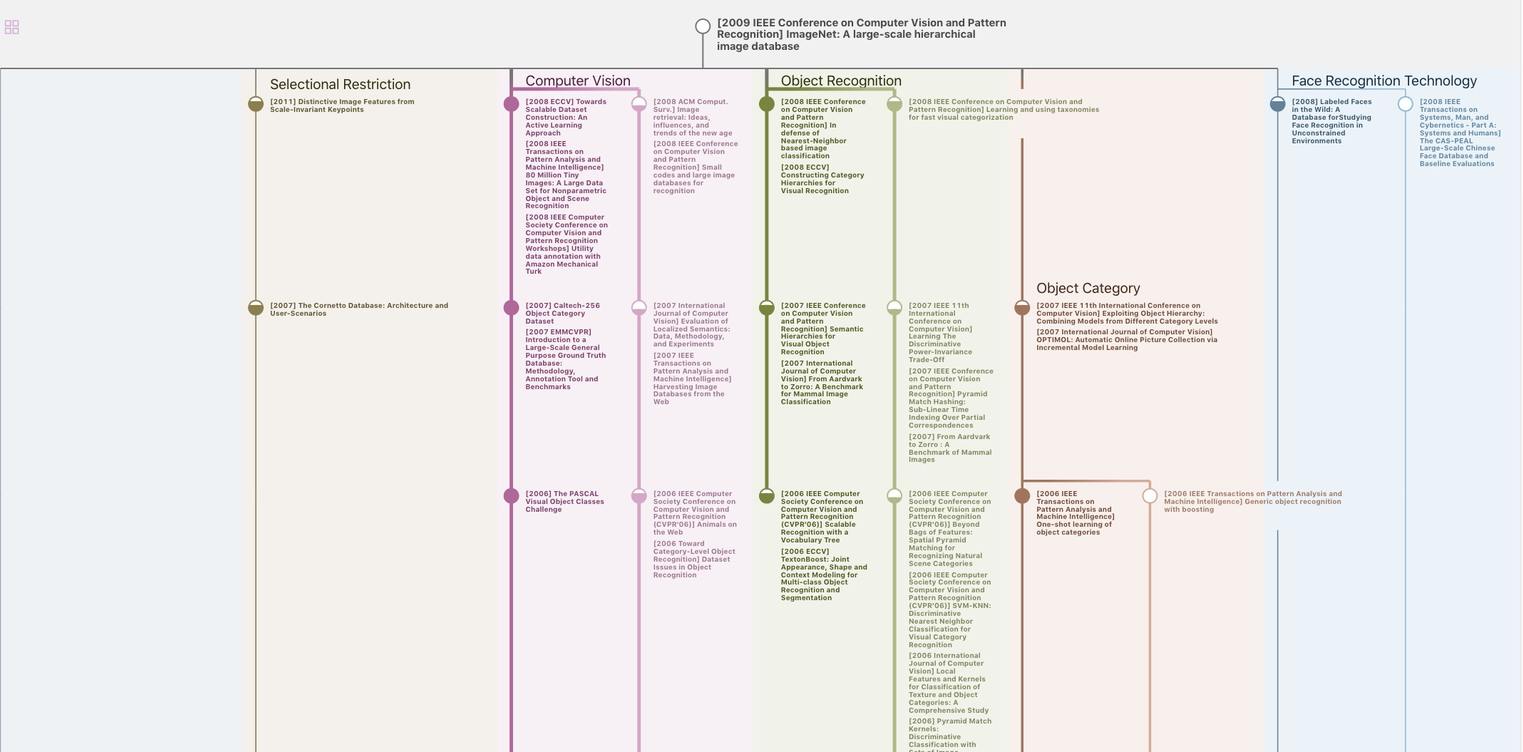
生成溯源树,研究论文发展脉络
Chat Paper
正在生成论文摘要