Analyzing Liquid Pouring Sequences Via Audio-Visual Neural Networks
2019 IEEE/RSJ INTERNATIONAL CONFERENCE ON INTELLIGENT ROBOTS AND SYSTEMS (IROS)(2019)
摘要
Existing work to estimate the weight of a liquid poured into a target container often require predefined source weights or visual data. We present novel audio-based and audio-augmented techniques, in the form of multimodal convolutional neural networks (CNNs), to estimate poured weight, perform overflow detection, and classify liquid and target container. Our audio-based neural network uses the sound from a pouring sequence-a liquid being poured into a target container. Audio inputs consist of converting raw audio into mel-scaled spectrograms. Our audio-augmented network fuses this audio with its corresponding visual data based on video images. Only a microphone and camera are required, which can be found in any modern smartphone or Microsoft Kinect. Our approach improves classification accuracy for different environments, containers, and contents of the robot pouring task. Our Pouring Sequence Neural Networks (PSNN) are trained and tested using the Rethink Robotics Baxter Research Robot. To the best of our knowledge, this is the first use of audio-visual neural networks to analyze liquid pouring sequences by classifying their weight, liquid, and receiving container.
更多查看译文
关键词
liquid pouring sequences,audio-visual neural networks,target container,source weights,audio-augmented techniques,multimodal convolutional neural networks,poured weight,audio-based neural network,audio inputs,raw audio,visual data,robot pouring task,pouring sequence neural networks,audio-augmented network,overflow detection,mel-scaled spectrograms,video images,classification accuracy,Rethink Robotics Baxter research robot
AI 理解论文
溯源树
样例
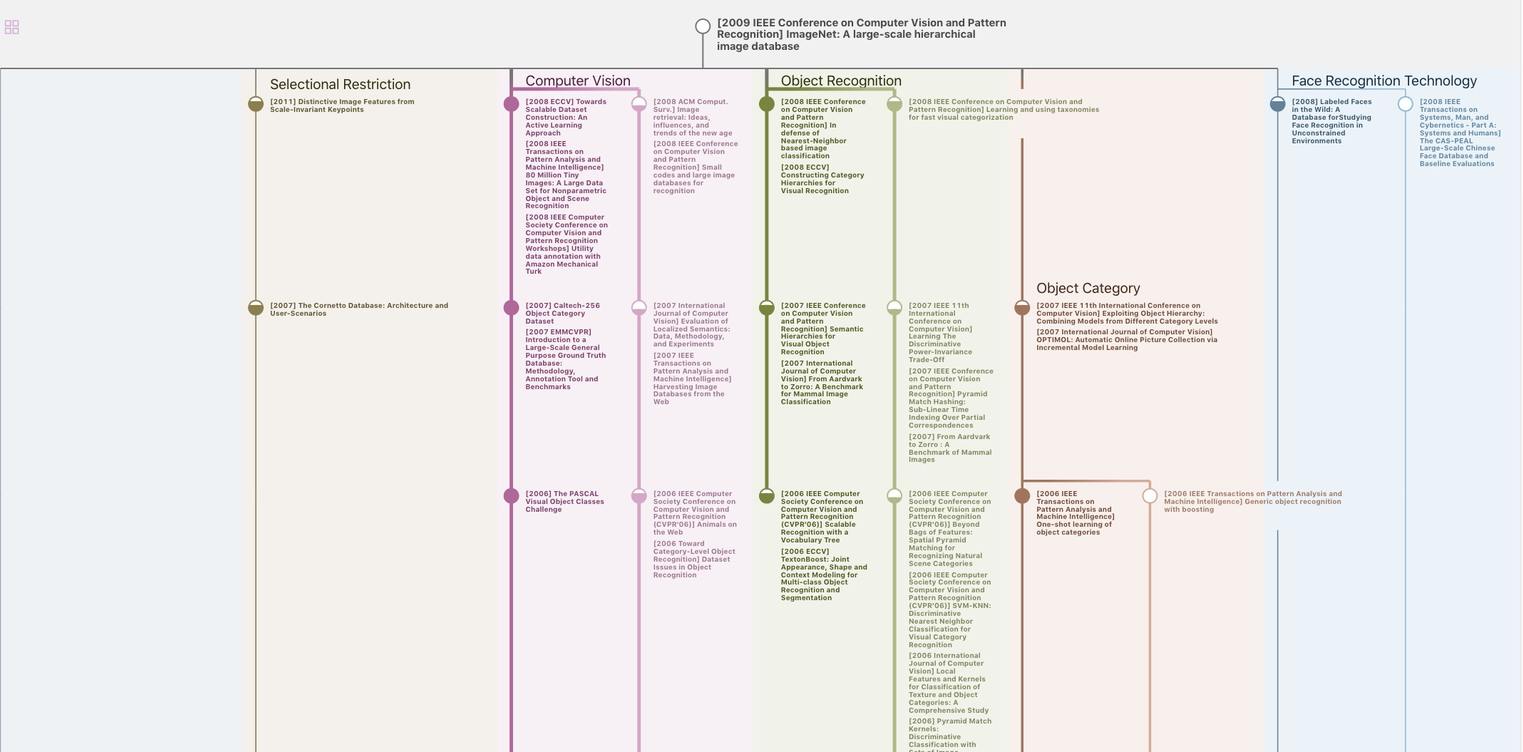
生成溯源树,研究论文发展脉络
Chat Paper
正在生成论文摘要