Ev-Imo: Motion Segmentation Dataset And Learning Pipeline For Event Cameras
2019 IEEE/RSJ INTERNATIONAL CONFERENCE ON INTELLIGENT ROBOTS AND SYSTEMS (IROS)(2019)
摘要
We present the first event-based learning approach for motion segmentation in indoor scenes and the first event-based dataset - EV-IMO - which includes accurate pixelwise motion masks, egomotion and ground truth depth. Our approach is based on an efficient implementation of the SfM learning pipeline using a low parameter neural network architecture on event data. In addition to camera egomotion and a dense depth map, the network estimates independently moving object segmentation at the pixel-level and computes per-object 3D translational velocities of moving objects. We also train a shallow network with just 40k parameters, which is able to compute depth and egomotion.Our EV-IMO dataset features 32 minutes of indoor recording with up to 3 fast moving objects in the camera field of view. The objects and the camera are tracked using a VICON (R) motion capture system. By 3D scanning the room and the objects, ground truth of the depth map and pixel-wise object masks are obtained. We then train and evaluate our learning pipeline on EV-IMO and demonstrate that it is well suited for scene constrained robotics applications.
更多查看译文
关键词
EV-IMO dataset features,3D scanning,event-based learning approach,dense depth map,camera egomotion,event data,low parameter neural network architecture,SfM learning pipeline,truth depth,first event-based dataset,pixel-wise motion masks,indoor scenes,event cameras,motion segmentation dataset,scene constrained robotics applications,pixel-wise object masks,ground truth,motion capture system,indoor recording,shallow network,moving objects,computes per-object 3D translational velocities,pixel-level,object segmentation
AI 理解论文
溯源树
样例
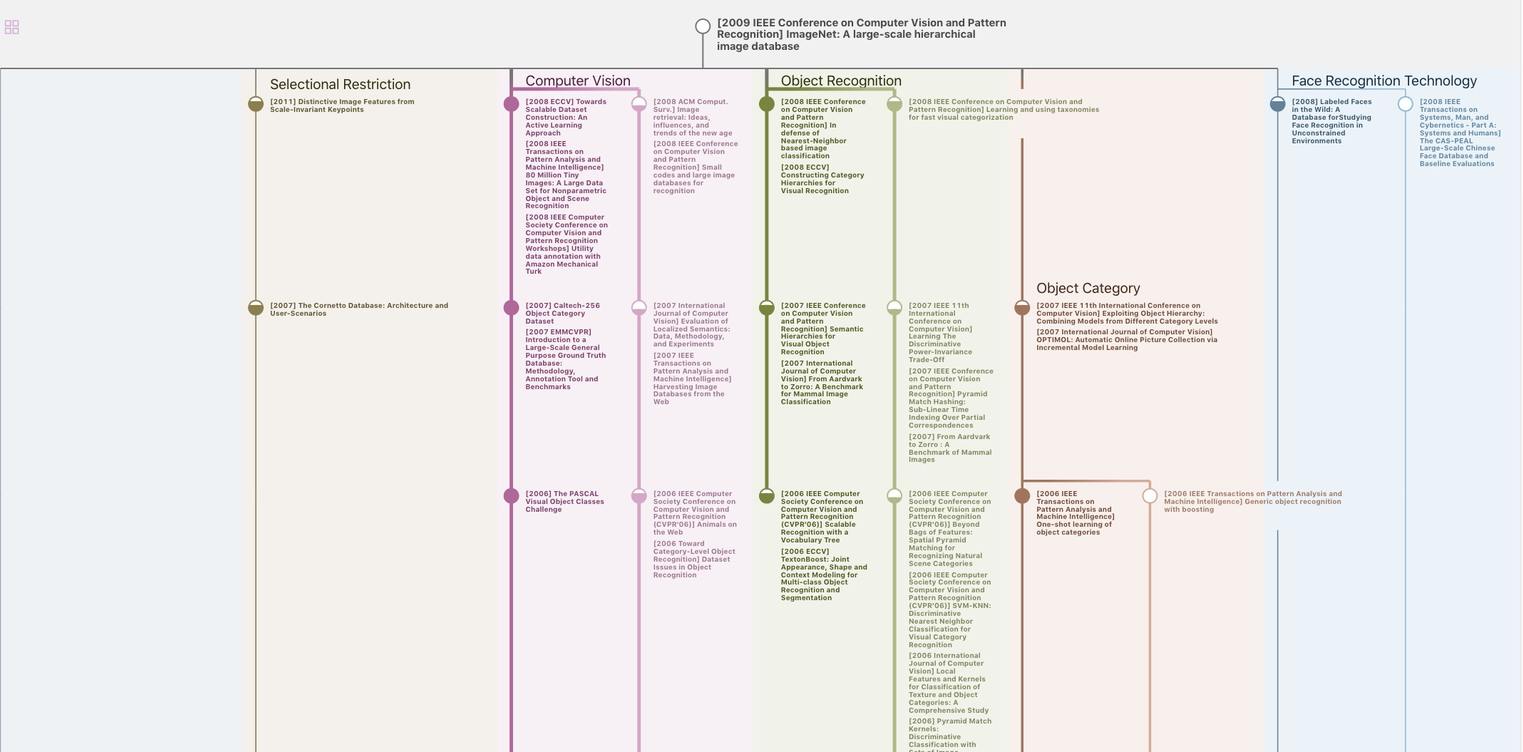
生成溯源树,研究论文发展脉络
Chat Paper
正在生成论文摘要