Empirical tail copulas for functional data
ANNALS OF STATISTICS(2021)
摘要
For multivariate distributions in the domain of attraction of a max-stable distribution, the tail copula and the stable tail dependence function are equivalent ways to capture the dependence in the upper tail. The empirical versions of these functions are rank-based estimators whose inflated estimation errors are known to converge weakly to a Gaussian process that is similar in structure to the weak limit of the empirical copula process. We extend this multivariate result to continuous functional data by establishing the asymptotic normality of the estimators of the tail copula, uniformly over all finite subsets of at most D points (D fixed). An application for testing tail copula stationarity is presented. The main tool for deriving the result is the uniform asymptotic normality of all the D-variate tail empirical processes. The proof of the main result is nonstandard.
更多查看译文
关键词
Extreme value statistics,functional data,tail empirical process,tail dependence,tail copula estimation,uniform asymptotic normality
AI 理解论文
溯源树
样例
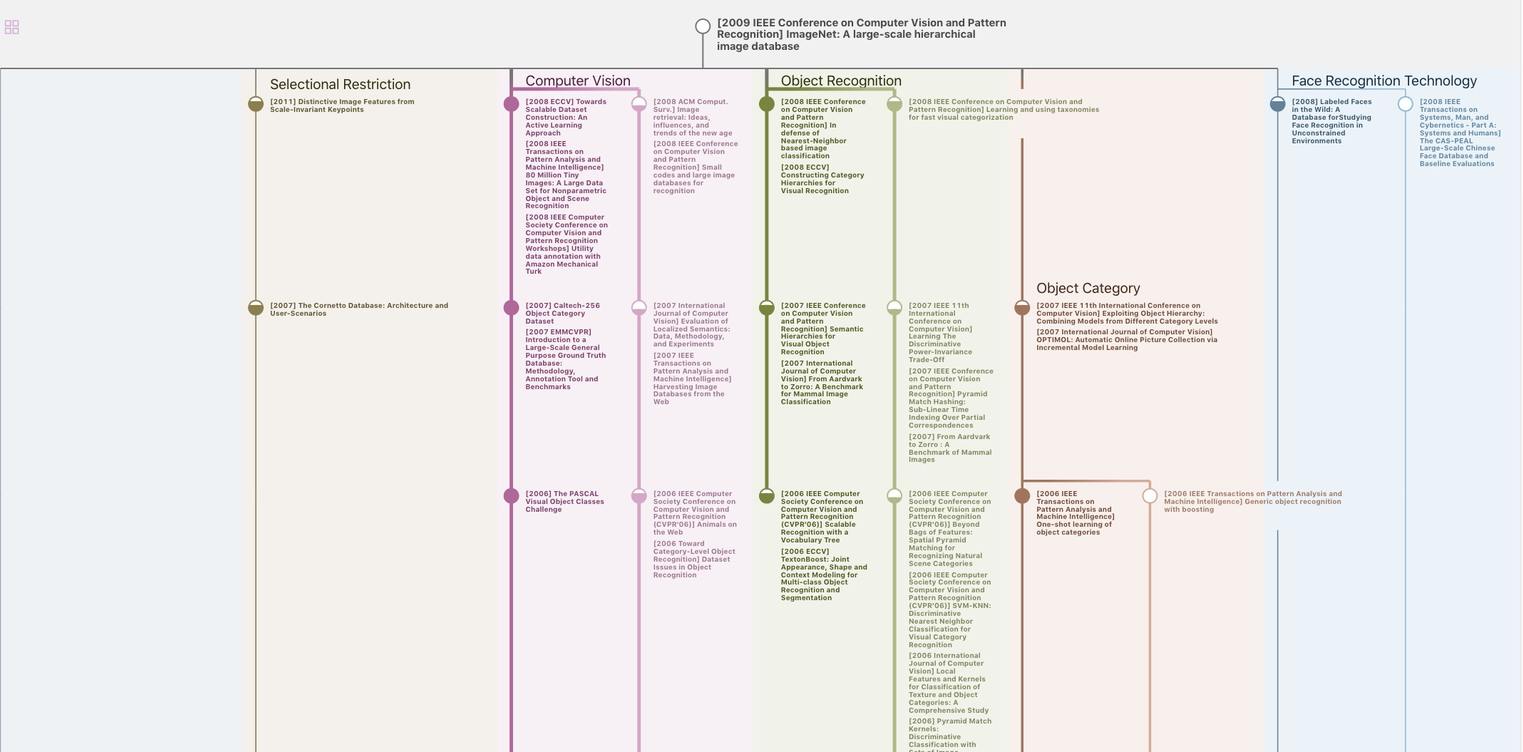
生成溯源树,研究论文发展脉络
Chat Paper
正在生成论文摘要