A family of pairwise multi-marginal optimal transports that define a generalized metric
arxiv(2023)
摘要
The Optimal transport (OT) problem is rapidly finding its way into machine learning. Favoring its use are its metric properties. Many problems admit solutions with guarantees only for objects embedded in metric spaces, and the use of non-metrics can complicate solving them. Multi-marginal OT (MMOT) generalizes OT to simultaneously transporting multiple distributions. It captures important relations that are missed if the transport only involves two distributions. Research on MMOT, however, has been focused on its existence, uniqueness, practical algorithms, and the choice of cost functions. There is a lack of discussion on the metric properties of MMOT, which limits its theoretical and practical use. Here, we prove new generalized metric properties for a family of pairwise MMOTs. We first explain the difficulty of proving this via two negative results. Afterward, we prove the MMOTs’ metric properties. Finally, we show that the generalized triangle inequality of this family of MMOTs cannot be improved. We illustrate the superiority of our MMOTs over other generalized metrics, and over non-metrics in both synthetic and real tasks.
更多查看译文
关键词
Optimal transport,Multi-marginal optimal transport,Metric spaces,Generalized metric spaces,Clustering,Hypergraph clustering
AI 理解论文
溯源树
样例
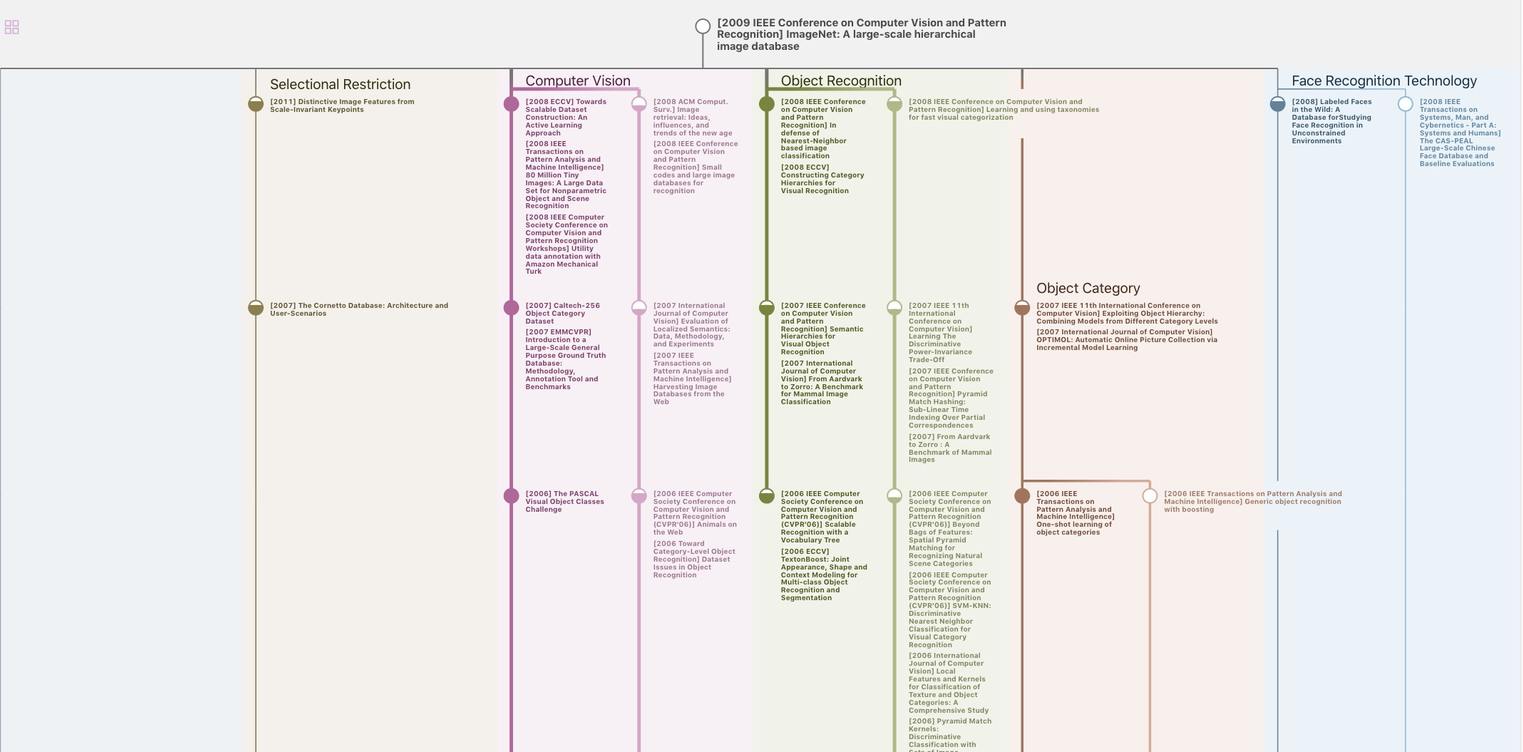
生成溯源树,研究论文发展脉络
Chat Paper
正在生成论文摘要