Audio-Visual Decision Fusion for WFST-based and seq2seq Models
arxiv(2020)
摘要
Under noisy conditions, speech recognition systems suffer from high Word Error Rates (WER). In such cases, information from the visual modality comprising the speaker lip movements can help improve the performance. In this work, we propose novel methods to fuse information from audio and visual modalities at inference time. This enables us to train the acoustic and visual models independently. First, we train separate RNN-HMM based acoustic and visual models. A common WFST generated by taking a special union of the HMM components is used for decoding using a modified Viterbi algorithm. Second, we train separate seq2seq acoustic and visual models. The decoding step is performed simultaneously for both modalities using shallow fusion while maintaining a common hypothesis beam. We also present results for a novel seq2seq fusion without the weighing parameter. We present results at varying SNR and show that our methods give significant improvements over acoustic-only WER.
更多查看译文
关键词
fusion,audio-visual,wfst-based
AI 理解论文
溯源树
样例
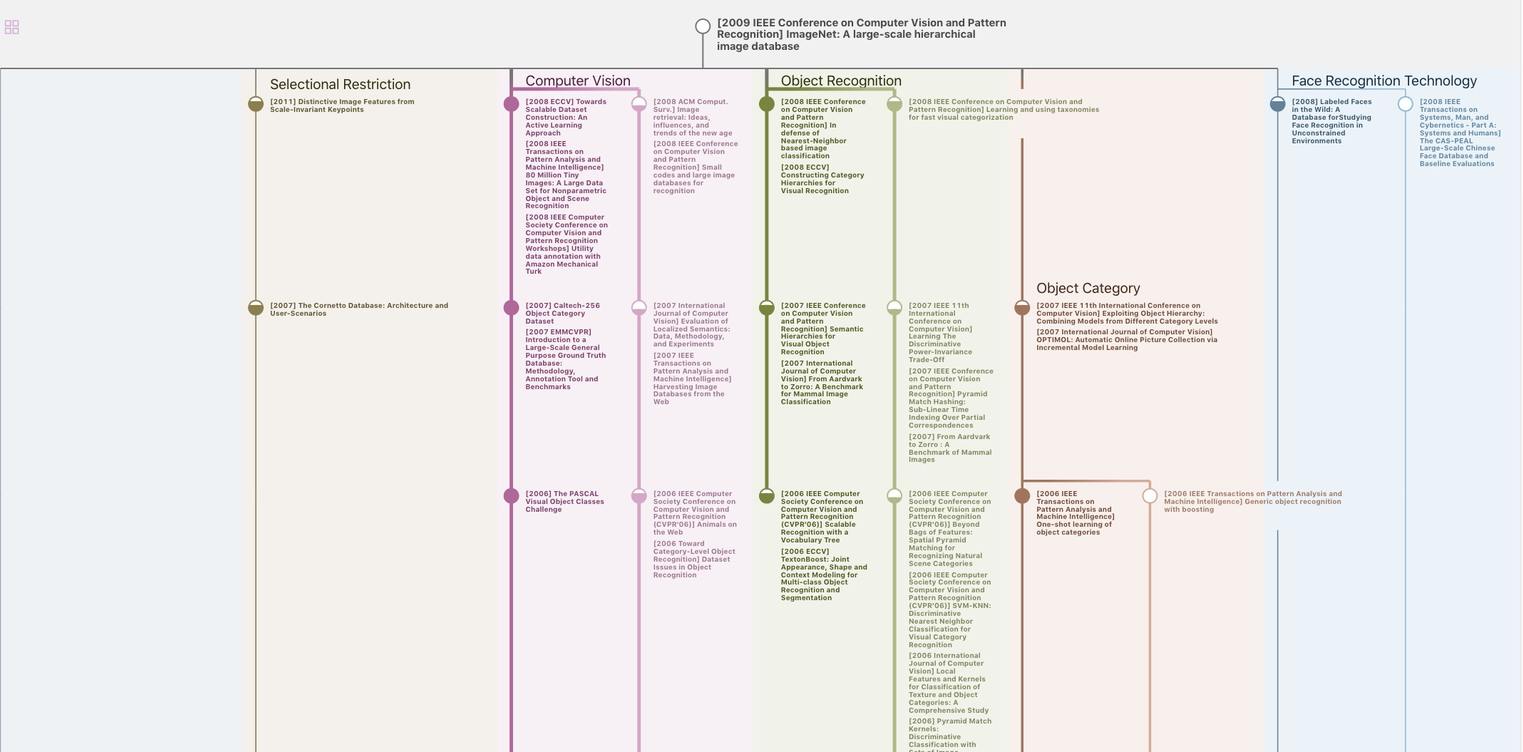
生成溯源树,研究论文发展脉络
Chat Paper
正在生成论文摘要