Ultra-Densenet For Low-Dose X-Ray Image Denoising In Cardiac Catheter-Based Procedures
STATISTICAL ATLASES AND COMPUTATIONAL MODELS OF THE HEART: MULTI-SEQUENCE CMR SEGMENTATION, CRT-EPIGGY AND LV FULL QUANTIFICATION CHALLENGES(2019)
摘要
The continuous development and prolonged use of X-ray fluoroscopic imaging in cardiac catheter-based procedures is associated with increasing radiation dose to both patients and clinicians. Reducing the radiation dose leads to increased image noise and artifacts, which may reduce discernable image information. Therefore, advanced denoising methods for low-dose X-ray images are needed to improve safety and reliability. Previous X-ray imaging denoising methods mainly rely on domain filtration and iterative reconstruction algorithms and some remaining artifacts still appear in the denoised X-ray images. Inspired by recent achievements of convolutional neural networks (CNNs) on feature representation in the medical image analysis field, this paper introduces an ultra-dense denoising network (UDDN) within the CNN framework for X-ray image denoising in cardiac catheter-based procedures. After patch-based iterative training, the proposed UDDN achieves a competitive performance in both simulated and clinical cases by achieving higher peak signal-to-noise ratio (PSNR) and signal-to-noise ratio (SNR) when compared to previous CNN architectures.
更多查看译文
关键词
ultra-densenet,low-dose,x-ray,catheter-based
AI 理解论文
溯源树
样例
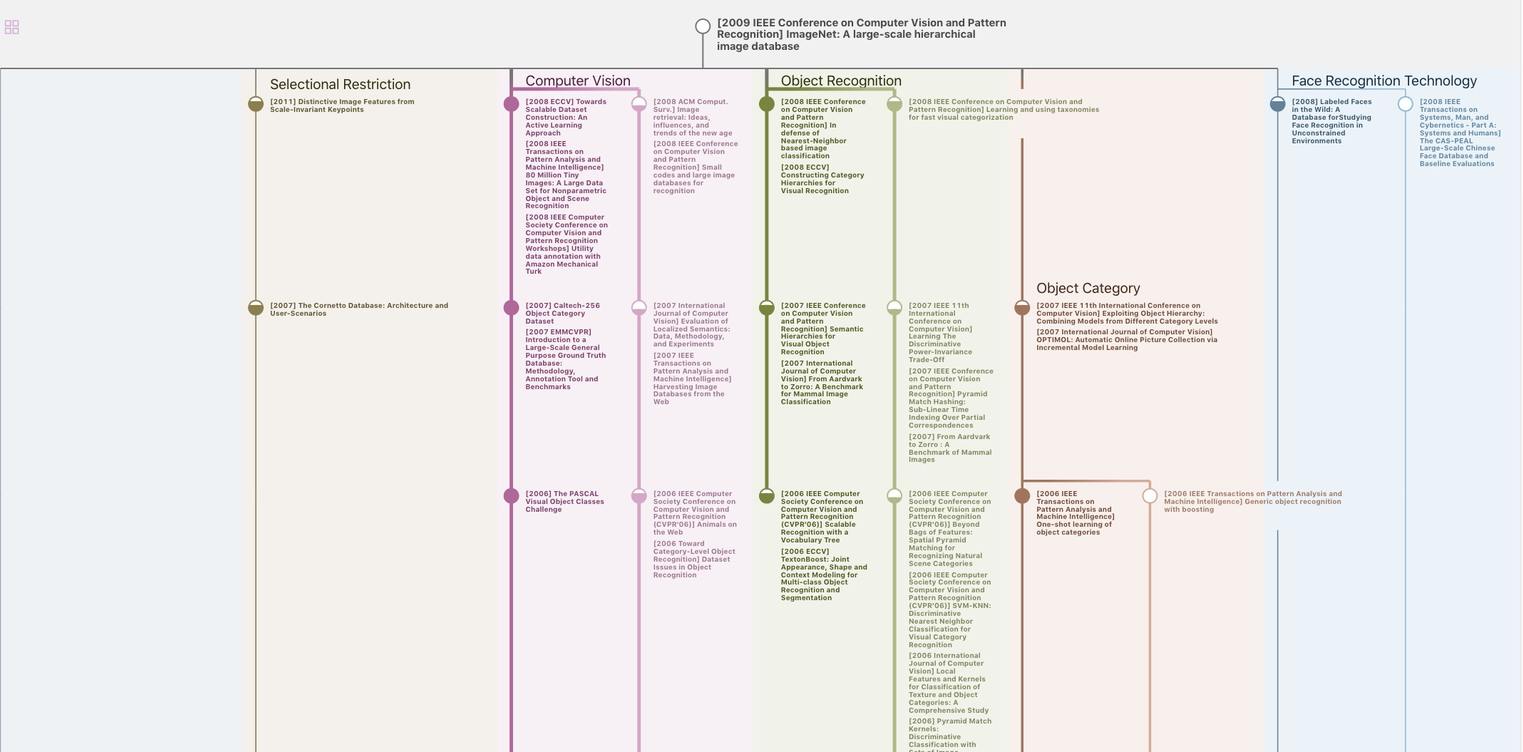
生成溯源树,研究论文发展脉络
Chat Paper
正在生成论文摘要