A random forest based approach for predicting spreads in the primary catastrophe bond market
Insurance: Mathematics and Economics(2021)
摘要
We introduce a random forest approach to enable spreads' prediction in the primary catastrophe bond market. In a purely predictive framework, we assess the importance of catastrophe spread predictors using permutation and minimal depth methods. The whole population of non-life catastrophe bonds issued from December 2009 to May 2018 is used. We find that random forest has at least as good prediction performance as our benchmark-linear regression in the temporal context, and better prediction performance in the non-temporal one. Random forest also performs better than the benchmark when multiple predictors are excluded in accordance with the importance rankings or at random, which indicates that random forest extracts information from existing predictors more effectively and captures interactions better without the need to specify them. The results of random forest, in terms of prediction accuracy and the minimal depth importance are stable. There is only a small divergence between the drivers of catastrophe bond spread in the predictive versus explanatory framework. We believe that the usage of random forest can speed up investment decisions in the catastrophe bond industry both for would-be issuers and investors.
更多查看译文
关键词
G1,G220
AI 理解论文
溯源树
样例
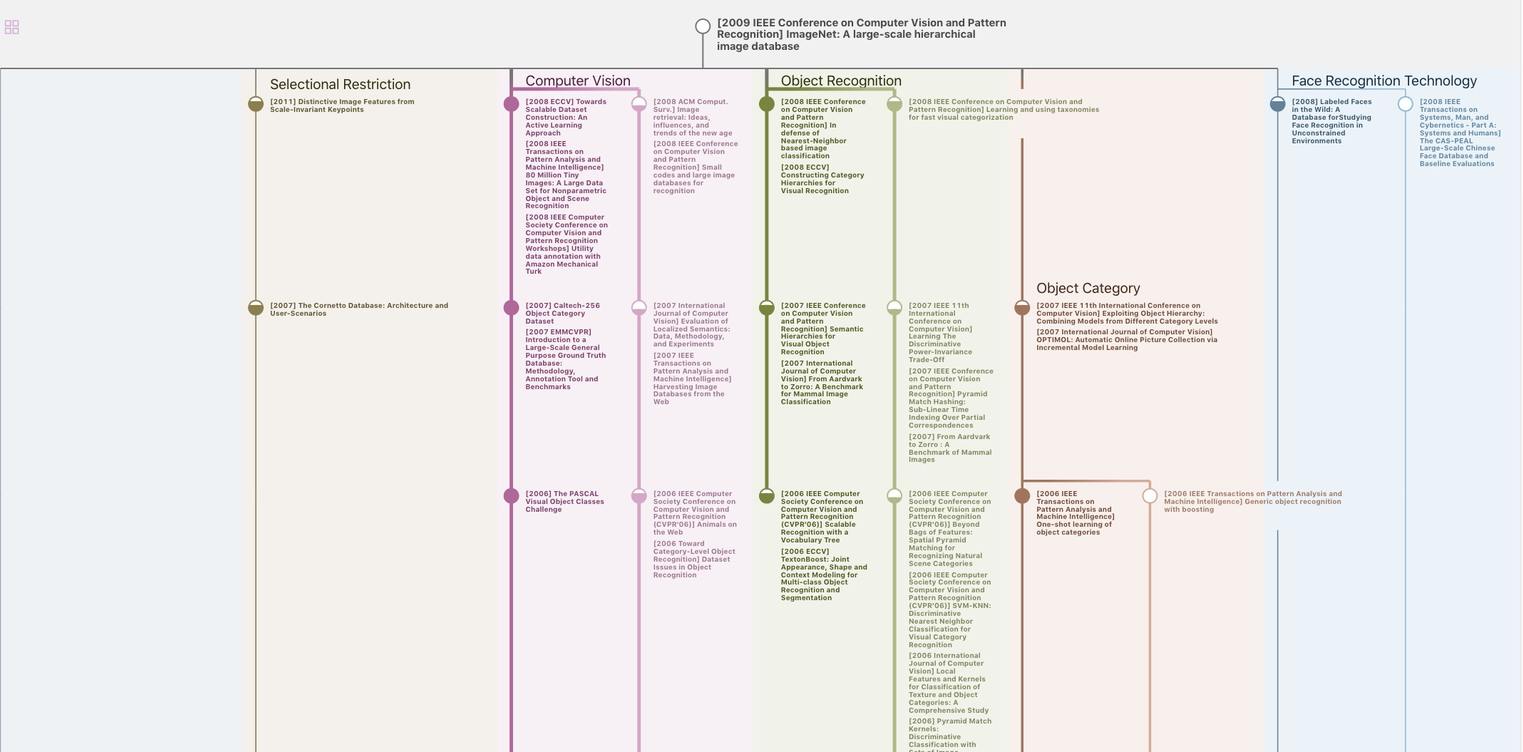
生成溯源树,研究论文发展脉络
Chat Paper
正在生成论文摘要